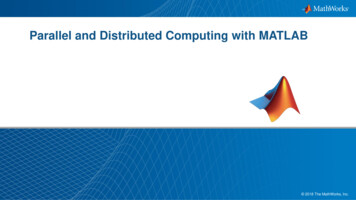
Transcription
Parallel and Distributed Computing with MATLAB 2018 The MathWorks, Inc.1
Practical Application of Parallel Computing Why parallel computing? Need faster insight on more complex problems with larger datasets Computing infrastructure is broadly available (multicore desktops, GPUs, clusters) Why parallel computing with MATLAB Leverage computational power of more hardware Accelerate workflows with minimal to no code changes to your original code Focus on your engineering and research, not the computation2
Steps for Improving Performance First get code working Speed up code with core MATLAB Include compiled languages and additional hardwareWebinar: Optimizing and Accelerating Your MATLAB Code3
Programming Parallel Applications Built-in multithreading– Automatically enabled in MATLAB since R2008a– Multiple threads in a single MATLAB computation engine Parallel computing using explicit techniques– Multiple computation engines controlled by a single session– High-level constructs to let you parallelize MATLAB applications– Perform MATLAB computations on GPUs4
Parallel rWorkerWorker5
Agenda Utilizing multiple cores on a desktop computer Scaling up to cluster and cloud resources Tackling data-intensive problems on desktops and clusters Accelerating applications with NVIDIA GPUs Summary and resources6
Programming Parallel ApplicationsBuilt in supportGreater Control– ., ‘UseParallel’, true)Ease of Use 7
Parallel-enabled ToolboxesImage ProcessingBatch Image Processor, BlockProcessing, GPU-enabled functionsSignal Processing andCommunicationsGPU-enabled FFT filtering,cross correlation, BERsimulationsStatistics and Machine LearningResampling Methods, k-Meansclustering, GPU-enabled functionsComputer VisionParallel-enabled functionsin bag-of-words workflowNeural NetworksDeep Learning, Neural Networktraining and simulationOptimizationParallel estimation ofgradientsOther Parallel-enabled Toolboxes8
Programming Parallel Applications Built in supportSimple programming constructs– parfor, batchEase of Use Greater Control– ., ‘UseParallel’, true)9
Embarrassingly Parallel: Independent Tasks or Iterations No dependencies or communication between tasks Examples:– Monte Carlo simulations– Parameter sweeps– Same operation on many filesTimeTime10
Mechanics of parfor Loops11223 34 4 55 6678 8 9 910 10Workera(i) i;a zeros(10, 1)parfor i 1:10a(i) i;endaWorkera(i) i;Workera(i) i;Workera(i) i;11
Tips for Leveraging PARFOR Consider creating smaller arrays on each worker versus one large arrayprior to the parfor loop Take advantage of parallel.pool.Constant to establish variableson pool workers prior to the loop Encapsulate blocks as functions when neededUnderstanding parfor12
Programming Parallel Applications Built in supportSimple programming constructs– parfor, batch Full control of parallelizationEase of Use Greater Control– ., ‘UseParallel’, true)– spmd, parfeval13
Agenda Utilizing multiple cores on a desktop computer Scaling up to cluster and cloud resources Tackling data-intensive problems on desktops and clusters Accelerating applications with NVIDIA GPUs Summary and resources14
Offloading dulerWorkerWorkerWorker15
Offloading Serial Computations job batch(.);WorkMATLABDesktop (Client)WorkerWorkerWorkerResultWorker16
Offloading and Scaling Computations job batch(. , 'Pool', n);WorkMATLABDesktop (Client)WorkerWorkerWorkerResultWorker17
Migrate to Cluster / Cloud Use MATLAB Distributed Computing Server Change hardware without changing algorithm18
Use MATLAB Distributed Computing Server1.Prototype codeDesktop ComputerLocalMATLABMATLABcodeDesktop (Client)Profile(Local)19
Use MATLAB Distributed Computing Server1.Prototype codeComputer ClusterCluster2.Get access to an enabled clusterSchedulerProfile(Cluster)20
Use MATLAB Distributed Computing Server1.Prototype codeDesktop ComputerComputer ClusterClusterLocal2.Get access to an enabled cluster3.Switch cluster profile to runon cluster resourcesMATLABMATLABcodeDesktop (Client)SchedulerProfileProfile(Local)(Cluster)21
Take Advantage of Cluster Hardware Offload computation:Computer Cluster– Free up desktopCluster– Access better computers Scale speed-up:– Use more cores– Go from hours to minutes MATLABDesktop (Client)SchedulerScale memory:– Utilize tall arrays and distributed arrays– Solve larger problems without re-coding algorithms22
Scale your applications beyond the desktopOptionParallel ComputingToolboxMATLAB Parallel CloudMATLAB DistributedComputing Server for AmazonEC2MATLAB DistributedComputing Server forCustom CloudMATLAB DistributedComputing ServerDescriptionExplicit desktop scalingSingle-user, basic scalingto cloudScale to EC2 with somecustomizationScale to custom cloudScale to clustersMaximumworkersNo limit16256No limitNo limitHardwareDesktopMathWorks ComputeCloudAmazon EC2Amazon EC2, OthersAnyAvailabilityWorldwideUnited States and CanadaUnited States, Canada and otherselect countries in EuropeWorldwideWorldwideLearn More: Parallel Computing on the Cloud23
Agenda Utilizing multiple cores on a desktop computer Scaling up to cluster and cloud resources Tackling data-intensive problems on desktops and clusters Accelerating applications with NVIDIA GPUs Summary and resources24
Tall and Distributed Data Tall Data – Columnar data that does not fit inmemory of a desktop or clusterDistributed Data– Large matrices using the combinedmemory of a clusterMachineMemory Common Actions– Data manipulation, math, statistics– Summary visualizations– Machine learning Common Actions– Matrix Manipulation– Linear Algebra and Signal Processing25
Tall Arrays New data type in MATLAB R2016b Applicable when:MachineMemory– Data is columnar – with many rows– Overall data size is too big to fit into memory– Operations are mathematical/statistical in nature Statistical and machine learning applications– Hundreds of functions supported in MATLAB andStatistics and Machine Learning ToolboxTall Data26
Execution Environments for Tall ArraysRun on Compute Clusters,or Spark if your data isstored in HDFS, for largescale analysisLocal diskShared foldersDatabasesProcess out-of-memory data onyour Desktop to explore,analyze, gain insights and todevelop analyticsUse Parallel ComputingToolbox for increasedperformanceSpark Hadoop27
Distributed Arrays Distributed Arrays hold data remotely on workers running on a cluster Manipulate directly from client MATLAB (desktop) 200 MATLAB functions overloaded for distributed arraysSupported functions for Distributed Arrays28
Agenda Utilizing multiple cores on a desktop computer Scaling up to cluster and cloud resources Tackling data-intensive problems on desktops and clusters Accelerating applications with NVIDIA GPUs Summary and resources29
Graphics Processing Units (GPUs) For graphics acceleration and scientific computing Many parallel processors Dedicated high speed memory30
GPU Requirements Parallel Computing Toolbox requires NVIDIA GPUs www.nvidia.com/object/cuda gpus.htmlMATLAB ReleaseRequired Compute CapabilityMATLAB R2014b and newer releases2.0 or greaterMATLAB R2014a and earlier releases1.3 or greater31
Built in toolbox support Simple programming constructs– gpuArray, gatherGreater Control Ease of UseProgramming with GPUs32
Built in toolbox support Simple programming constructs– gpuArray, gather Advanced programming constructs– spmd, arrayfun Greater Control Ease of UseProgramming with GPUsInterface for experts– CUDAKernel, mex33
Speed-up using NVIDIA GPUs Ideal Problems– Massively Parallel and/or Vectorized operations– Computationally Intensive– Algorithm consists of supported functions 300 GPU-enabled MATLAB functions Additional GPU-enabled Toolboxes– Algorithm consists of supported functions– Neural Networks– Image Processing– Communications– Signal Processing. Learn more34
Agenda Utilizing multiple cores on a desktop computer Scaling up to cluster and cloud resources Tackling data-intensive problems on desktops and clusters Accelerating applications with NVIDIA GPUs Summary and resources35
Summary Easily develop parallel MATLAB applications without being a parallelprogramming expert Speed up the execution of your MATLAB applications using additionalhardware Develop parallel applications on your desktop and easily scale to a clusterwhen needed36
Some Other Valuable Resources MATLAB Documentation– MATLAB Advanced Software Development Performance and Memory– Parallel Computing Toolbox Parallel and GPU Computing Tutorials– nd-gpu-computing-tutorials97719.html Parallel Computing on the Cloud with MATLAB– ng/parallel-computing-on-thecloud/37
MATLAB and Simulink are registered trademarks of The MathWorks, Inc. See www.mathworks.com/trademarks for a list of additional trademarks. Otherproduct or brand names may be trademarks or registered trademarks of their respective holders. 2018 The MathWorks, Inc. 2018 The MathWorks, Inc.38
Programming Parallel Applications Built-in multithreading –Automatically enabled in MATLAB since R2008a –Multiple threads in a single MATLAB computation engine Parallel computing using explicit techniques –Multiple computation engines controlled by a single session –High-level constructs to