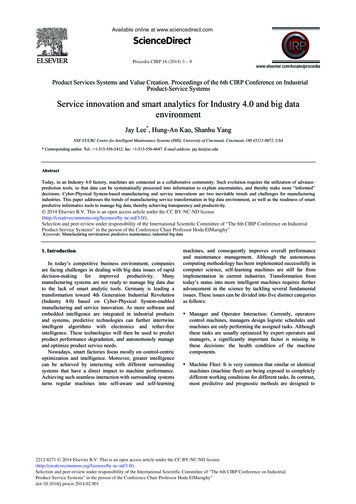
Transcription
Available online at www.sciencedirect.comScienceDirectProcedia CIRP 16 (2014) 3 – 8Product Services Systems and Value Creation. Proceedings of the 6th CIRP Conference on IndustrialProduct-Service SystemsService innovation and smart analytics for Industry 4.0 and big dataenvironmentJay Lee*, Hung-An Kao, Shanhu YangNSF I/UCRC Center for Intelligent Maintenance Systems (IMS), University of Cincinnati, Cincinnati, OH 45221-0072, USA* Corresponding author. Tel.: 1-513-556-3412; fax: 1-513-556-4647. E-mail address: jay.lee@uc.eduAbstractToday, in an Industry 4.0 factory, machines are connected as a collaborative community. Such evolution requires the utilization of advanceprediction tools, so that data can be systematically processed into information to explain uncertainties, and thereby make more “informed”decisions. Cyber-Physical System-based manufacturing and service innovations are two inevitable trends and challenges for manufacturingindustries. This paper addresses the trends of manufacturing service transformation in big data environment, as well as the readiness of smartpredictive informatics tools to manage big data, thereby achieving transparency and productivity. Authors.byopenElsevierB.V.article under the CC BY-NC-ND license 20142014TheElsevierB.V.PublishedThis is anaccessSelectionand peer-review under responsibility of the International Scientific Committee of “The 6th CIRP Conference on Industrial nd/3.0/).ServiceSystems”in the personof theConference ofChairHodaScientifiElMaraghy.c Committee of “The 6th CIRP Conference on IndustrialSelectionand peer-reviewunderresponsibilitythe ProfessorInternationalProduct-Service Systems” in the person of the Conference Chair Professor Hoda ElMaraghy”Keywords: Manufacturing servitization; predictive maintenance; industrial big data1. IntroductionIn today’s competitive business environment, companiesare facing challenges in dealing with big data issues of nufacturing systems are not ready to manage big data dueto the lack of smart analytic tools. Germany is leading atransformation toward 4th Generation Industrial Revolution(Industry 4.0) based on Cyber-Physical System-enabledmanufacturing and service innovation. As more software andembedded intelligence are integrated in industrial productsand systems, predictive technologies can further intertwineintelligent algorithms with electronics and tether-freeintelligence. These technologies will then be used to predictproduct performance degradation, and autonomously manageand optimize product service needs.Nowadays, smart factories focus mostly on control-centricoptimization and intelligence. Moreover, greater intelligencecan be achieved by interacting with different surroundingsystems that have a direct impact to machine performance.Achieving such seamless interaction with surrounding systemsturns regular machines into self-aware and self-learningmachines, and consequently improves overall performanceand maintenance management. Although the autonomouscomputing methodology has been implemented successfully incomputer science, self-learning machines are still far fromimplementation in current industries. Transformation fromtoday’s status into more intelligent machines requires furtheradvancement in the science by tackling several fundamentalissues. These issues can be divided into five distinct categoriesas follows: Manager and Operator Interaction: Currently, operatorscontrol machines, managers design logistic schedules andmachines are only performing the assigned tasks. Althoughthese tasks are usually optimized by expert operators andmanagers, a significantly important factor is missing inthese decisions: the health condition of the machinecomponents. Machine Fleet: It is very common that similar or identicalmachines (machine fleet) are being exposed to completelydifferent working conditions for different tasks. In contrast,most predictive and prognostic methods are designed to2212-8271 2014 Elsevier B.V. This is an open access article under the CC BY-NC-ND nd/3.0/).Selection and peer-review under responsibility of the International Scientific Committee of “The 6th CIRP Conference on IndustrialProduct-Service Systems” in the person of the Conference Chair Professor Hoda ElMaraghy”doi:10.1016/j.procir.2014.02.001
4Jay Lee et al. / Procedia CIRP 16 (2014) 3 – 8support a single or limited number of machines andworking conditions. Currently, available prognostic andhealth management methods are not taking advantage ofconsidering these identical machines as a fleet by gatheringworthwhile knowledge from different instances. Product and Process Quality: As the final outcome of themanufacturing process, product quality can provide muchinsight on machine condition via backward reasoningalgorithms. Product quality can provide feedback forsystem management, which can be used to improveproduction scheduling. Currently, such feedback loop doesnot exist and needs further research. Big Data and Cloud: Data management and distribution inBig Data environment is critical for achieving self-awareand self-learning machines. The importance of leveragingadditional flexibility and capabilities offered by cloudcomputing is inevitable, but adapting prognostics andhealth management algorithms to efficiently implementcurrent data management technologies requires furtherresearch and development. Sensor and Controller Network: Sensors are the machine’sgateway to sense its surrounding physical environment.However, sensor failure and degradation may pass wrongand inaccurate readings to decision-making algorithms,which will result in an incorrect outcome.With these issues in mind, the objective of the paper is toreview how current manufacturing industries evolve for theupcoming industrial big data environment, and propose thekey technology for sustainable innovative service. The paperis organized as follows: Section 2 focuses on trends of serviceinnovation in manufacturing industries and unmet needs of anIndustry 4.0 factory; Section 3 describes the proposed selfaware and self-maintenance machine systems based onindustrial big data analysis; Section 4 presents two casestudies that have been conducted to demonstrate thefeasibility of the proposed framework; and Section 5concludes the paper with some perspectives.section, previous research on these two topics will bereviewed and discussed.2.1. Manufacturing servitization and innovationMany advanced countries whose economic base is themanufacturing industry have made efforts to transform theireconomy and reinvigorate the industry. They suffer threatsfrom emerging markets and the global manufacturing supplychain. Therefore, manufacturing firms not only seekmanufacturing technique innovation, but are also beginning tofocus on induction and impetus of service. This way, thefuzzy boundary of the manufacturing industry and serviceindustry drive will stimulate the development ofmanufacturing servitization.Servitization was proposed by Vandermerve and Rada in1988 [1]. They emphasized the concept of customer focus;combining products, services, support, and knowledge are themost important elements. Furthermore, the authors alsoasserted that not only service industries, but alsomanufacturing industries should focus on innovative valueadded service development in order to quickly enhance rvitization as innovation of organizational capabilities andprocesses, from product sales to integrated product services[2].Servitization is defined as the strategic innovation of anorganization’s capabilities and processes to shift from sellingproducts, to selling an integrated product and service offeringthat delivers value in use, i.e. a Product-Service System [3].The concept of a Product Service-System (PSS) is a specialcase of servitization. Mont defines PSS as a system ofproducts, services, supporting networks, and infrastructurethat is designed to be competitive, satisfy customers' needs,and have a lower environmental impact than traditionalbusiness models [4]. In the PSS business model, industriesdevelop products with value-added services, instead of asingle product itself, and provide their customers withservices that are needed. In this relationship, the market goalof manufacturers is not one-time product selling, butcontinuous profit from customers by total service solution,which can satisfy unmet customers’ needs.2. Trends and unmet needs for Industry 4.0 era2.2. Industrial big data environmentThe discovery of new technologies has escorted industrydevelopment from the early adoption of mechanical systems,to support production processes, to today’s highly automatedassembly lines, in order to be responsive and adaptive tocurrent dynamic market requirements and demands. Under theIndustry 4.0 concept, astounding growth in the advancementand adoption of information technology and social medianetworks has increasingly influenced consumers’ perceptionon product innovation, quality, variety and speed of delivery.This requires establishing the factory with capabilities of lf-reconfiguration, and self--maintenance. Accompaniedwith this new technology, two types of innovativedevelopment are receiving more attention by academia andindustries: service innovation and industrial big data. In thisRecently, big data becomes a buzzword on everyone’stongue. It has been in data mining since human-generatedcontent has been a boost to the social network. It has also beencalled the web 2.0 era since late 2004 [5]. Lots of researchorganizations and companies have devoted themselves to thisnew research topic, and most of them focus on social orcommercial mining. This includes sales prediction, userrelationship mining and clustering, recommendation systems,opinion mining, etc. [6-10]. However, this research focuses on‘human-generated or human-related data’ instead of ‘machinegenerated data or industrial data’, which may include machinecontrollers, sensors, manufacturing systems, etc.Under the above-mentioned Industry 4.0 era, intelligentanalytics and cyber-physical systems are teaming together to
Jay Lee et al. / Procedia CIRP 16 (2014) 3 – 8realize a new thinking of production management and factorytransformation. Using appropriate sensor installations, varioussignals such as vibration, pressure, etc. can be extracted. Inaddition, historical data can be harvested for further datamining. Communication protocols, such as MTConnect [11]and OPC, can help users record controller signals. When all ofthe data is aggregated, this amalgamation is called “Big Data”.The transforming agent consists of several components: anintegrated platform, predictive analytics, and visualizationtools. The deployment platform is chosen based on: speed ofcomputation, investment cost, ease of deployment and update,etc. [12]. The actual processing of big data into usefulinformation is then the key of sustainable innovation within anIndustry 4.0 factory.3. Self-aware and self-maintenance machines forindustrial big data environmentThe recent developments of an Internet of Things (IOT)framework and the emergence of sensing technology havecreated a unified information grid that tightly connectssystems and humans together, which further populates a bigdata environment in the industry. With more advancedanalytics, the advent of cloud computing and a CyberPhysical Systems (CPS) framework, future industry will beable to achieve a fleet-wide information system that helpsmachines to be self-aware and actively prevents potentialperformance issues. A self-aware and self-maintainedmachine system is defined as a system that can self-assess itsown health and degradation, and further use similarinformation from other peers for smart maintenance decisionsto avoid potential issues. Smart analytics for achieving suchintelligence will be used at the individual machine and fleetlevels.For a mechanical system, self-awareness means being ableto assess the current or past condition of a machine, and reactto the assessment output. Such health assessment can beperformed by using a data-driven algorithm to analyzedata/information collected from the given machine and itsambient environment. The condition of the real-time machinecan be fed back to the machine controller for adaptive controland machine managers for in-time maintenance. However, formost industrial applications, especially for a fleet ofmachines, self-awareness of machines is still far from beingrealized. Current diagnosis or prognosis algorithms areusually for a specific machine or application, and are notadaptive or flexible enough to handle more complicatedinformation. The reasons for why a self-aware machine hasnot been fully realized are summarized as follows:Lack of a closely coupled human-machine interaction: amajor influential factor for machine condition andperformance is human operation and management.Productivity and production quality can be greatly affected bytask design and scheduling. Current machines can onlypassively listen to the operators’ commands and react, evenwhen the assigned task is not optimal for its current condition.A smarter machine system, on the other hand, should be ableto actively suggest task arrangements and adjust operationalparameters to maximize productivity and product quality.Lack of adaptive learning and full utilization ofavailable information: PHM systems cannot be widelyimplemented in the industry because of their low level ofadaptability, which eventually leads to a lack of robustness inthe health monitoring algorithms. The problem behind suchan issue is that for a PHM system, development andimplementation are usually separated. The PHM algorithm isdeveloped by data collected from experiments, and does notchange during implementation unless being re
Service innovation and smart analytics for Industry 4.0 and big data environment Jay Lee*, Hung-An Kao, . business models [4]. In the PSS business model, industries develop products with value-added services, instead of a single product itself, and provide their customers with services that are needed. In this relationship, the market goal of manufacturers is not one-time product selling .