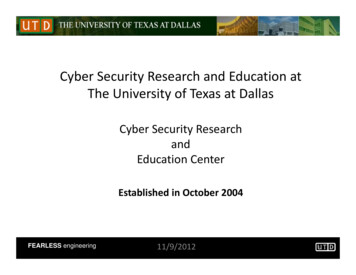
Transcription
Cyber Security Research and Education atThe University of Texas at DallasCyber Security ResearchandEducation CenterEstablished in October 2004FEARLESS engineering11/9/20121
FacultyFounderProf. Bhavani Thuraisingham, PhD, DEng (U of Wales, U of Bristol - UK), October 2004Core FacultyProf. Alvaro Cardenas, PhD (U of MD) – Spring 2013 – Control Systems SecurityProf. Yvo Desmedt, PhD (U. Leuven-Belgium) – Fall 2012 - CryptographyProf. Kevin Hamlen, PhD (Cornell U) – Fall 2006 - Language and Software SecurityProf. Murat Kantarcioglu, PhD (Purdue U) – Fall 2005 - Data Security and PrivacyProf. Zhiqiang Lin, PhD (Purdue U) – Fall 2011 - Systems Security and ForensicsProf. Yiorgos Makris, PhD (UC San Diego) – Fall 2011 - Hardware SecurityProf. Kamil Sarac, PhD (UC Santa Barbara) – Spring 2010 -Network SecurityProf. Latifur Khan, PhD (U of Southern CA) – Spring 2005 - Data Mining for SecuritySeveral additional faculty are affiliated with the Center from ECS, SOM, EPPS.BBS, NSM; They bring expertise inRisk Analysis, Economics of Security, Game Theory for Modelling theAdversary, and Psychology of Hackers, among othersFEARLESS engineering
Our Accomplishments NSA/DHS Center for Excellence in both Education(2004) and Research (2008) 20m in Research Funding and 3m in educationfunding Prestigious grants and contracts including:Multiple NSF Career, AFOSR YIP, DoD MURI Fellowships and Awards: IEEE, AAAS, IACR Fellowships IEEE and ACM Awards Numerous Keynote addresses, Top‐tier Journal andConference Publications, Open Source Tools andPrototype Development, PatentsFEARLESS engineering
Our SponsorsFEARLESS engineering
Research Thrusts Policy‐based Data, Information and KnowledgeManagement– Topics include Assured Information Sharing, PrivacyPreserving Record Linkage, Secure Social Networks,Inference Control Malware Detection/Prevention– Topics include Data Mining for Malware Detection,Active Defense, Botnet Risk Management, LanguageSecurity, Network Defense, Hardware Security, SmartPhone Security Cloud Security– Topics include Secure Virtualization, Secure Storage,Secure Data Management , Malware AnalysisFEARLESS engineering
Information Operations Across Infospheres:Assured Information SharingDr. Bhavani Thuraisingham, University of Texas at DallasObjectives Develop a Framework for Secure and Timely DataSharing across Infospheres Investigate Access Control and Usage Control policiesfor Secure Data Sharing Develop innovative techniques for extractinginformation from trustworthy, semi‐trustworthy anduntrustworthy partners Incentive‐based Information SharingScientific/Technical Approach Conduct experiments as to how much information islost as a result of enforcing security policies in thecase of trustworthy partners Develop more sophisticated policies based on role‐based and usage control based access controlmodels Develop techniques based on game theoreticalstrategies to handle partners who are semi‐trustworthy Develop data mining techniques to carry outdefensive and offensive information operationsFEARLESS engineeringData/Policy for CoalitionPublish Data/PolicyPublish Data/PolicyPublish Data/PolicyComponentData/Policy forAgency AComponentData/Policy forAgency CComponentData/Policy forAgency BAccomplishments Developed an experimental system for determininginformation loss due to security policy enforcement Developed a strategy for applying game theory forsemi‐trustworthy partners; simulation results Developed data mining techniques for conductingdefensive operations for untrustworthy partnersChallenges Handling dynamically changing trust levels;Scalability
Layered Framework forAssured Cloud Computing (AFOSR)PoliciesXACMLUser onitorsSecure VirtualNetwork MonitorFigure2. Layered Framework for Assured Cloud11/9/20127
Assured Information Sharingin the CloudCollaboration with our European Partners; Funded by USAFAgency1Agency2Agencyn User Interface LayerRelational DataFine‐grained AccessControl with HiveRDF DataSPARQL Query Optimizerfor Secure RDF DataProcessing
OS‐Sommelier (ACM SoCC’12):Memory-Only Operating System Fingerprinting in the CloudDr. Zhiqiang Lin MotivationCloud runs virtual machine (VM)Each VM runs a particular OSPenetration testing, IntrospectionKernel update (management)Technical ApproachPGDIdentificationPhysical MemorySnapshotPGD-iKernel CodeIdentificationCore KernelCode PagesSignaturesSignatures(arrays of MD5s)SignatureMatchingSignatureGenerationResult
Virtual Machine (VM) Space Traveler (IEEE S&P’12):Automatically Bridging the Semantic‐Gap for VMIDr. Zhiqiang LinMotivation: Using Secure VM to Supervise other VMsTrusted OSLinuxThe Semantic-Gap ProblemWin-7LinuxTrusted cure‐VMVirtualization LayerProduct‐VMIntrospectHardware LayerThe-State-of-the-Art[VMI, NDSS’03][SBCFI, CCS’07]Our Innovation[VMST, SP’12][Problem,HotOS’01] [VMWatcher,CCS’07] [Virtuoso,SP’11]Semantic Gap
Bin‐Carver (DFRWS’12):Automatic Recovery of Binary Executable FilesDr. Zhiqiang Lin MotivationBinary Executable Files are everywhereMalicious software (virus, trojans)Recovery the deleted executable files foranalysisTechnical ApproachDiskImage DELF-HeaderScannerELF-HeaderhiBlock NodeLinkerConflict NodeResolverELF-File ei
Privacy‐preserving Distributed Data AnalyticsPI: Dr. Murat Kantarcioglu (NSF/NIH) MotivationPrivacy sensitive data that is needed for manycritical tasks is distributed among differentorganizations– Statistical analysis of hospital dischargedata for detecting biological weaponsattacks.Privacy concerns may hinder sharing such datafor legitimate purposesOur goal is to develop techniques to enabledistributed data mining without sacrificingindividual privacyTechnical Approach and Results Idea: Combine sanitization and cryptographictechniques to enable efficient and accurateprivacy‐preserving distributed data analyticsResults: We have developed Privacy‐preserving record linkageprotocols Privacy‐preserving approximate datamining protocols Two open source toolbox related to dataprivacyFEARLESS engineeringCryptographic ProtocolsSanitizedData ProcessingSanitizedData 1 (Public)SanitizedData 2 (Public)Data SanitizationData SanitizationSource Data 1(Private)Source Data 2(Private)Result
Secure Provenance Data ManagementPI: Dr. Murat Kantarcioglu (NSF)Motivation Understanding the pedigree (i.e. provenance)of the data is important for decision making Example: Intelligence reports Where do they come from? Who modified them?Sharing provenance data is important fordecision makingPotential security issues in sharing provenancedataTechnical Approach and Results Represent provenance data as RDF graphDefine access control and redaction policies onRDF data using graph grammarsUse semantic web technologies for efficientenforcement of policiesInitial Results: Developed efficient prototype to handlevarious provenance access control andredaction policiesFEARLESS engineering
Adversarial Data MiningPI: Dr. Murat Kantarcioglu (Army)Motivation Data mining models are built for detectingmalicious activity Spam Filtering Credit card fraud detection Intrusion detectionAttackers adapt to defeat data mining modelsby changing behaviorMore robust models are needed for adversarialdata miningTechnical Approach and ResultsApply game theory ideas to data miningModel adversarial behavior to build morerobust classifiersConsider adversarial goals in attributeselectionInitial Results: We have developed Novel game theoretic framework foradversarial data mining Modified adversarial SVM algorithmFEARLESS engineering
Language‐based Securityat UT DallasDr. Kevin W. Hamlen
Securing Software from the InsideProgramming Languagescompilersbinary translationautomated theoremprovingformal methodsComputer Securityinformation assuranceLanguage-basedtrustworthy computingprivacySecurityreputation managementmalware defense Three example projects:– Securing Web Advertisements– Binary Stirring: Self‐diversifying Software– Frankenstein: A Futuristic Malware Monster
Web AdvertisementsWeb AdSource: WeightwatchersLanguage: ActionScript/FlashAd Distribution ScriptSource: Google AdSenseLanguage: JavaScriptInstant Messaging PortalSource: Microsoft MessengerLanguage: ASP.NETEmail Viewer/Editor ScriptSource: Microsoft HotmailLanguage: JavaScript ASP.NET
The Malicious Ad Problem Web Scripting Languages are Insecure– Flash Remote Code Execution Exploit was most‐attacked web scripting vulnerability in 2011– over 4500 new web‐based attacks per day– average of 82 targeted attacks per day [source: Symantec Vulnerability Trends Report, 2011] Ad Scripting is here to stay– primary source of revenue for most sites– 31B worldwide in 2011 [source: IAB]– Anything that impairs functionality will not beadopted by the industry.
Our Solution: FlashJaXAdvertiseradPagePublisherweb pageFlashJaXWeb Client 1.2M NSF‐funded collaborativeresearch grant with UIC Client‐side / Page Publisher‐sideprotection against malicious ads– transforms untrusted Flash scriptsat a binary level as they load– fully transparent to advertisers– runs as a Java script in the browser– NO change required to browsers,works on all OSes, etc.– works on real ad networks with nonet overhead (Google Adsense,Microsoft Media, Yahoo Ads,Clicksor, etc.)
Binary Stirring:Self‐diversifying SoftwareRichard Wartell, Vishwath Mohan,Dr. Kevin Hamlen, Dr. Zhiqiang LinThe University of Texas at DallasSupported in part by NSF, AFOSR, and DARPA23
Attack VectorMaliciousInputBuffer Overflow24
Attacks TimelineExecuteCode onthe Stack1980Make StackNon‐exec(WxorX)Return toUnsafe UserCode Gadgets(Shacham, Q[8,1])Return zeLibrary ImageBase(ASLR)2010?25
Defense Strategy Most of these attacks require attackers to know or predictthe location of binary features– e.g., function locations, instruction locations, etc. Problem: Locating features is easy for attackers becauseall instances of each application look roughly the sameDefense GoalFrustrate such attacks by randomizingfeature space or removing features26
Our Solution: STIR(Self‐Transforming Instruction Relocation)User AddressSpace231lib1 Imbue x86 native code binaries withthe power to re‐randomize them‐selves at load‐time– Every instance of every binary is different– NO code‐producer cooperation, so easy todeploy– Tested on 100 Windows and Linuxbinaries– 99.99% feature randomization on average– 1.6% performance overhead on averagelib2lib3main2027
“Stirring” Accomplishments Published in CCS 2012, ACSAC 2012, ECML 2011– R. Wartell, V. Mohan, K. W. Hamlen, and Z. Lin. BinaryStirring: Self‐randomizing Instruction Addresses of Legacyx86 Binary Code. ACM CCS, 2012.– R. Wartell, V. Mohan, K. W. Hamlen, and Z. Lin. SecuringUntrusted Code via Compiler‐Agnostic Binary Rewriting.ACSAC 2012, forthcoming.– R. Wartell, Y. Zhou, K. W. Hamlen, M. Kantarcioglu, and B.Thuraisingham. Differentiating Code from Data in x86Binaries. ECML PKDD, 2011. Finalist in the 2012 AT&T CSAW “Best Applied SecurityPaper of the Year” competition– (winner to be decided next month)
Frankenstein:Cyber‐offensive OperationsVishwath Mohan, Dr. Kevin HamlenThe University of Texas at DallasSupported in part by AFOSR Active Defense29
Attackerlow bandwidthInfection Survivability in aHostile EnvironmentVictim NetworkAttacker: maximize infection survivalDefender: minimize infection survival
Diversification as an Attack Randomize features during propagation– Polymorphism encrypt payload with randomly chosen key– Oligomorphism xor payload with randomly chosen one‐time pad– Metamorphism non‐deterministically recompile payload Weaknesses– Obfuscations create diversity but not stealth diverse replicas still differ from surrounding software– Mutation is undirected weak against targeted (e.g., semi‐manual) defenses
Our Solution: Frankenstein Stitch together code harvested frombenign binaries to re‐implementmalware on each propagation. Many advantages– resulting malware is 100% metamorphic no common features between mutants– statistically indistinguishable from benign‐ware everything is plaintext code (no cyphertexts)– no runtime unpacking evades write‐then‐execute protections– obfuscation is targeted and directed evolves to match infected system’s notion of “benign”
Press Coverage Presented at USENIX Workshop on Offensive Technologies mid‐AugustThousands of news stories in August/September– The Economist, New Scientist, NBC News, Wired UK, The Verge,Huffington Post, Live Science,
The Hunt for the Kill SwitchAdee, IEEE Spectrum, 2008Do youTrustYour Chips?Yiorgos Makris(yiorgos.makris@utdallas.edu)Research Supported by:2012 Phobos-Grunt Mission Fails Dueto Counterfeit Non Space-Rated ChipsThe Hacker in Your Hardware,Villasenor, Scientific American 2010
Counterfeit Integrated CircuitsYiorgos Makris (yiorgos.makris@utdallas.edu)Counterfeit ICs: Low quality/reliability replicasor recycled parts from used electronic boardsFailure rateInfant mortalityBrandnew devicesWear-out stageCounterfeitICsFailtimetUse Parametric Profile to Detect CounterfeitsParameter 2UpperspecBrand new performancedistributionSemiconductor Industry Loses 100B/yearCase‐Study on Aged Chip Data Provided by TIEigenvector 3CounterfeitdistributionNon‐linear eter 1Eigenvector 1Eigenvector 2 100% Accuracy for Batches of 10 Chips
Hardware Trojans in Wireless Crypto ICsYiorgos Makris (yiorgos.makris@utdallas.edu)Integrated Circuit (IC) Supply ChainHardware Trojans Can Leak Sensitive Data Wireless Crypto ICs encrypt datavia on‐chip key and transmit Hardware Trojans implanted byadversary can leak the key Contaminated transmissionsappear perfectly legitimateStatistical Analysis to the Rescue Trojans impose structure which can be statistically exposedFunded by the National Science Foundation via NSF 1149465
Proof-Carrying Hardware IP (PCHIP)Yiorgos Makris (yiorgos.makris@utdallas.edu)Third-Party Hardware Intellectual Property Majority of design effort currently on integration ofmodules from 3rd party hardware IP providers How do we assert that 3rd party IP is Trojan-free?Initial Demonstration on DES Core Checker fails when Trojan logic leaking the keyto the primary outputs is added in the designBorrow Proof-Carrying Code (PCC) Principles Vendor & Buyer agreeon security properties Vendor designs circuitand proof codes forsecurity properties Buyer automaticallychecks design‐proofpair through checker Mathematically notpossible to evadePCHIPFunded by the Army Research Lab via ARO / CS 60709
Malware Application Detection for Smart PhonesAppStatic Analysis& Mining Substantial increase of malware applications forAndroid and lack of robust malware detection software. Static analysis techniques fail with the slightest use ofencryption or encoding– Use of Native code extensions thwarts static analysis– Dynamic code loading/execution circumvents staticanalysis techniques– Obfuscation techniques render static analysis ineffective(if the apk is decompiled to java) Extended features extracted from dynamic analysis canbe used in data mining algorithms (e.g., SNOD—streambased novel class detection). AnalyzeManifest files Get list ofevents/activitiesDynamicAnalysis &Mining System Calls connect,open,read Dalvik Calls All API calls Network Calls Http trafficcoming in orgoing out ofthe deviceMalware/Benign?
Our Cyber Security Education ProgramsDirector: Prof. Kamil Sarac Undergraduate level ‐ (in CS Department)– Information Assurance (IA) Certificate (for CS students) 3 senior level cyber security classes– Minor in IA (for non‐CS students) 3 cyber security classes their pre‐requisites Graduate level ‐ (in CS Department)– IA Concentration Track 5 IA specific CS courses 6 other CS courses– IA Certificate (for CS students) 3 IA specific CS courses Extra‐curricular training– Computer Security Group (CSG) ‐‐ our student club Peer/student organized hands on training Participation in cyber security competitions
Scholarships in Cyber Security Education DoD IA Scholarship Program (IASP)– Supports undergrads for junior and senior years– Supports grads for two‐year MS education– DoD employment after graduation NSF Scholarship for Service Program (SFS)– Supports grads for two‐year MS education– Supports fast‐track student for senior year two year MSeducation– Employment in government after graduation Federal or State or Local or Tribal Government Both programs provide full support– Tuition/stipend/book allowance/conference travel
ContactDr. Bhavani ThuraisinghamExecutive Director, Cyber Security Research and Education CenterBhavani.thuraisingham@utdallas.eduDr. Kamil SaracDirector, Cyber Security Educationksarac@utdallas.eduMs. Rhonda WallsProject Coordinator, Cyber Security Research and Education CenterRhonda.walls@utdallas.eduMr. Vaibhav KhadilkarPhD Student, Cyber Security Research and Education Centervvk072000@utdallas.eduFEARLESS engineering
Prof. Zhiqiang Lin, PhD (Purdue U) – Fall 2011 - Systems Security and Forensics Prof. Yiorgos Makris, PhD (UC San Diego) – Fall 2011 - Hardware Security Prof. Kamil Sarac, PhD (UC Santa Barbara) – Spring 2010 -Network Security Pro