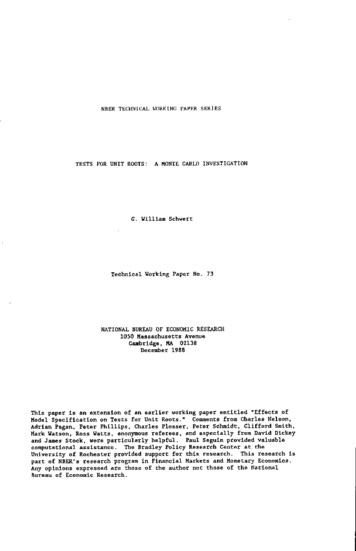
Transcription
NEER TECHNICAL. UORKSNC EAPER SERIESTESTS FOR UNIT ROOTS: A MONTE CARLO INVESTIGATIONC. William SchwertTechnical Working Paper No. 73NATIONAL BUREAU OF ECONOMIC RESEARCH1050 Massachusetts AvenueCambridge, MA 02138Oeceisber 1988This paper is an extension of an earlier working paper entitled "Effects ofModel Specificetion on Tests for Unit Roots. Comments from Charles Nelson,Adrian Pagan, Peter Phillips, Charles Plosser, Peter Schmidt, Clifford Smith,Mark Watson, Ross Watts, anonymoua referees, and especially from Oavid Oickeyand James Stock, were particularly helpful. Paul Seguin provided valuablecomputational assistance. The Bradley Policy Research Center at theUniversity of Rochester provided support for this research. This research ispart of NBER's research program in Financial Markets and Monetary Econoaice.Any opinions expressed are those of the author not those of the NationalRereau of Economic Research.
NSER Technical Working Paper #73December IS8TESTS FOR UNIT ROOTS: A MONTE CARLO INVESTIGATIONRecentwork by Said and Dickey(1964,1965). t'hillips(1957). and Phillipsend Perron(1988) esslEines tests for unit roots in the autoregressive part ofndxed autoregressive-integrated-sioving average (ARIMA) models (tests forstationerity). Monte Carlo experisents show that these unit root tests havedifferent finite sample distributions than the unit root tests developed byFuller(1976) and Dickey and Fuller(1979,198i) for autoregressive processes. Inparticular, the tests developed by Phillips(1987) and Phillips and Perron(1988)seem more sensitive to usodel stespecification thsn the high order autoregressiveapproxi.Estioo suggested by Said and Dickey(1984).C. William SchwertWilliam E. Simon Graduate Schoolof Business AdministrationUniversity of RochesterRochester, NY 14627
1. INTRODUCTIONFuller(1976) and Dickey and Fuller(1919,1981) develop several tests ofwhether a th order autoregressive (AR) process. Y1 Ut.—(1)is stationary. Stationarity implies that the roots of the lag polynomial4(L) — (1-,. 41?)-lie outside the unit circle (see Box andJenkins(1976) for a discussion of stationarity in the context of ARThe null hypothesis in these tests La that the AR processprocesses).contains one unit root, so the sun of the autoregressive coefficients in U)equals 1. Dickey endFuller—a piexcept the coefficientmodelDY u,.(2)which is equivalent to the AR model in (1),-where DYti —estimate the(P) should equal 1.0 if there is s unit root. Dickeyand Fuller use Monte Carlo experiments to tabulate the sampling distributionof the regression t-statistic, r —standard error of the estimate(-l)/s(?). whereis thecalculated by least squares. Thedistribution is skewed to the left and has too many large negative valuesrelativeto theStudent-t distribution. See Dickey, Nell and Miller(1986) fora recent discussion of autoregressive wait root teats. Plosser andSchwert(l971)in thediecuss a similarproblem thatmoving average polynomial. Thisarises whenthereis a unit rootcsn occur when differencing is used toremove nonststionsrity and the true model is a stationary end invertible ANNAmodelarounda time trend.This paper analyzes the sensitivity of the Dicksy-Fuller tests to theassumptionthatthe timeseriesisgenerated bya pure autoregressive process.
In particular, when a variable is generated by a mixed ARrMA process thecritical values implied by the Mckay-Fuller simulations can be .sisleading.Section 2 describes recent extensions of the Dickey-Fuller test proceduresuggested by Said and Dickay(1984, 1985), Phillips(1987), Phillips andPerron(l988), end Perron(1966e.b) that attempt to account for mixed AEtNAprocesses as well as pure AR processes in performing unit root tests. Section3 contains results of a Monte Carlo experiment that calculates the size of theDickey-Fuller and the related test statistics when the true process is ARIMArather then AR. Section 4 contains concluding remarks.2. EXTENSIONS OF TUE DICKET-FULLER TESTSSaidand Dickey(1984) argue that an unknownARIMA(pl,q) processcan beadequately approximated by an ARIHA(k,O,O) process, where k—o(T1"3). Giventhis approximation, the limiting distribution of the unit root test based on ahigh order AR approximation will be the same ss the Dickey-Fullerdistribution. Of course, for a given application this argument does notindicate the appropriate number of lags k.To understand why a finite order autoregressive process may not provide anadequate approximation to a mixed AETNA process, it is useful to consider theinfiniteorder autoregreacive proceaa i.pliad by an AZIMACO,l,l) processforparameter S. The autoregressivedifferent value,of the movingcoefficients arecalculated by matching coefficients c the lag operator L intheaveragerelationsr(L)—(l-L)f(l-eL)— (l-IL)wherer(L)—(l-L),xi is the autoregressive coefficient at lag 1. The autoregressivecoefficients decay slowly for large absoluta values of the moving average2
parameter. The suminfiniteofthecoefficients is equal to unity (the value for thesum of all autoregressive coefficients for this nonstationaryprocess) to four deciisal plates after 24 lags for 8 equal to .5 or -.5. Forvalues of 8 equal to .81 .9end .93,however, the sums of the coefficients tolags are equal to .9953. .9202, and .70801 respectively. This auggeststhat the approximation error caused by estimating finite order A?. process is24largefor moving everage parameters greater than .8. Such series havesutocorrelations for the levels of the series that decay slowly, and firstorder eutocorreletione for the first differences close to -.50(seeWichern(l973) end Schwert(l987)).Said and Dickey(1985) show that the unit root estimator from anA1114&(l.0,l) process,Yt —0 p - 8(3)u1,has the asymptotic distribution tabulated by Dickey and Fuller when one Cause-Newtonstep is taken from initial values p—l end 8estimator conditional on p—1shows the effect oftestTheyequal toa consistentprovide limited Monte Carlo evidence thatestimating the moving average parameter on the unitroot -statistic apFuller(l976. p. 371) presents fractiles of the distributionof T(-l) whenend a-'O for an ARIMACI,0,0) process,Y e PY1 u.Thist'-l, .F.(4)normalized leasure of bias provides another test of the unit roothypothesis.are morebasedDickey end Fuller(l979) show that teatsbased on this statisticpowerful against the alternative hypothesis that p l than theon the r statistic.1teat
depends on the structure of the ARIKAThe distribution of the estimatorprocess that generated the data. As noted by Fuller(1976, pp. 373-382), thestatistic Tc(-l) froa a general ARIKA aodel has the same distribution asT(?-l) from the AR(l) model, where the constant c is the aiim of thecoefficientsfrom the moving average representation of the errors from (4).#(L)—8(L)/#(L). One strategy for estimating the constant c is to use theadditional coefficients from the ARIMA(p,O.O) model in (2), or from enare the (p-i) autoregressive coefficients forARIKA(p,O,q) model, whereDYt -Phillips(1987)end Phillips end Perron(198S) also show that the Dickey-Fuller tests ere affected by autocorrelation in the errors from (4), Theydevelop modifications of the teat statietics r end T(-1) that have-theasymptotic distributions tabulated by Dickey and Fuller, when the data followIn fact, these papers allow for more generalan AXIMA(p.O.q) process.dependence in the error process, including conditional heteroskedasticity.These adjustments involve the autocovariancea of the errors from anARIMA(l,O,O) model in (4). They modify the test statistic T(?-l),Z — T(-l)-. 5(4 a)T2 tE(Y 1 -Y 1)2),(5)where s is the sample variance of the residuala utuT22z1—T Xu 2TLw41IJ—l ' t—J lt—land the weights— (1-j/(1 l))(6)ensure that the estimate of the varianceis positive (ace t4ewey and Weet(l987)). Yellowing the intuition of Saidand Dickey(1984), they suggest that the number of lags 1 of the residualin (6) be allowed to grow with the sample size T.Pbillips and Perron modify the regression etteatt r.autocovariancea4-
- .S(4.s2) I (41tp —where 4is defined In2ç - Y)2I1/2(7)(6).Dickey and Fuller also consider tests with a tine trend included as anadditional regressor, an the alternative hypothesis is a stationary processaround a time trend. Thus, the ARII4A(1,O,O)—a p(t-(T 1)/2) t-l u,the AItIMA(1,O,l) model—a fi (9)in (3) is modified so(t-(T l)/2J u - pand the ARIMMp.O.O) process In—model in (4) is modified so(2) is modified(p-fl4It-(Tl)/2] .(9)u1,Csoi(10)DY i u.The regression "t-tests," r,, are isporcant because Evans and Ssvin(1984) showthatrstatistics are a function of the unknownOn the other hand, Including a tine trend intrendintercept(8) (9),a in (2) or(4).or (10), even when thecoefficient fl—O, makes the distribution of the autoregressive parameterestimatethe valueindependent of o. In empirical applications, where knowledgeofof the intercept e is unavailable, inclusion of a tins trend isprohshly a prudent decision in performing unit root tests.PhillipsandPerron(1988) develop adjustsients to the Dickey-Fuller testsT(-l) and t wherethe alternative hypothesisis a stationary ARIMA(p,0,q)process around a deterministic tine trend. They show that the test statistic,—TO,-l)-(41-s)(T6/24D).(11)has the asymptotic distribution tabulated by Dickey and Fuller for T(-i.) in5
the AR114A(l.0.O)case,whereisthedeterminant of the regressor crossis:product matrix. Their modification to the statisticZ—TV(5U5TJ)-(s-s)(12){T1 4(30)1'2 }This statistic should have the ssyisptotic distribution tabulated by Dickey andFuller for r, even when the regression errors in (8) are autocorrelated.3. A MONTE CARLO EXFERIXENT FOR UNIT ROOT TESTSThe Monte Carlo experiment examines the effects of model misspecificationon the size of unit root tests for mixed ARIMAconstructs the data to follow an—processes.The experimentARIMA(O,l,l) process. Ut - 6t—-19. .where the errors (u} are serially uncorrelated standard normal variables.Thedata are generated by setting u20 and 20 equal to 0observations, discarding the firstinitial conditions.and creating Tt2020 observations to remove the effect of theSamples of size I —25,50, 100, 250. 500, and 1000 mrsused in the experiments. Each experiment is replicated 10.000 times to createthe sampling distribution for the test statistics. The moving averageparameter I is set equal to .8, .5, 0. -.5, and-.8,which implies first orderautocorrelations for the first differentea of these series of -.49,-.40, 0,.40 and .49. The first order autocorrelation coefficient for an ARIMA(0,0,1)process equals 6,(l.I2). Higher order autocorrelations equal 0.3.1. Regression t-tasts"Several tests of nonstationarity are performed on sach data series. First,the regression t-test' from (4) studied by Mckay and Fuller is calculated toillustrate the prblems that occur when the data are generated by a process6
other than piR(i), Second, two versions of the Phillips and t'erron(lS&C) testarecalculated, usingdifferent numbers oflags I of theresidualautocorrelations in calculating s in (6):(13a)14 — Int(4(T/100)V4)l2 —Intpjl2(T/100)),(13b)so 144 and 112 12 when T—100, (when 7—25, 142 and l2 when 7—1000, 247and 112 Il). Third, an AR1MA(l,O,l) model is astimeted to test whether theautoregressive coefficient p equals 1.0, using the t-test,—(?.1)/s().where s() is the standard error calculated by aniterative nonlinear least squares algorithm. Note that this is nt theprocedure suggested hy Said and Dickey(l985), since their results require onlyone Ceuss-Newton etep from the unit root. Nevertheless, empiricel researcherswho estimate ARIMA(l,0,l) models end discover an estimated autoregressiveparameter close to unity would want to know the reliability of the "t-testforthe unit root when iterative least squares is used. Fourth, an AR(14)model is estimated in equation (2) and the regression t-test" is used to testwhether p equals 1. Finally, an AR(112) model is estimated in equation (2)tocalculate r.Thelatter tests follow the suggestion of Said andDicksy(1984) to use a high order autoregressive process to approximate anunknown ARIMA process where the order of the autoregression grows with theseiaple sire T as in (13a,b).Table la contains estimates of the sisea of teats using the 1.1 criticalvalues from the Dickey-Fuller distribution for v patstistics (AR(l); Phillips-Perron with 4 lags,l2 lags, Z(112L;aample sires (7 —ARfl4A(l,0,l);for theZ(14);six different testPhillips-Parron withAR(14); and A1t(1l2)) for the sit different25, 50, 100, 250. 500, and1000), and for the five different
values of the moving average parameter for the true process (6 —.5.8.5. 0. -end -.8). where the alternative hypothesis is a stationary APJ4A processaround a constant mean. Table lb contains the estimates of the sizes of teatsusing the 5'. critical values. These tables do not report the upper tall ofthe sampling distributions because the usual alternative hypothesis ie thatthe process is stationary (p 1). As previously reported by Dickey andFuller the distribution of the r statistics has a negative mean and ispskewed toward negative values for all of the cases considered in theseexperiments, Additional information about these sampling distributions isavailable from the author on request. The simulations were programmed inFORTRANusingthe IMSL subroutine COIIOF to generate pseudo-random normalvariates. All results were checked using the RATS computer program.The first thing to note about Tables la and lb is that the eimple AR(l)testIs severely affected by the presence of moving average components in thedata generation process. The estimated size for this test ie positivelyrelated to the moving average parameter 6, being too large for 8—.S or .8, andtoo small for 6—- .5 or - .8.Of course, this problem is exactly what motivatesthe tests proposed by Said and Dickey end by Phillips and Perron.Second, the Phillips and Patron tests do not have distributions that areclose to the Dickey-Fuller distribution, especially for 9—.5 or .8. At boththe 1% and 5% levels, the size of the Phillips-Perron teats are muchlargerthan the nominal size of the test even for samples as large as T'-lOOO. Asthe number of lags of the residual autocorrelations used in (6) increases from14 to l2 the size estimates become farther away from the Dickey-Fullerresults. The Phillips-Perron tests era muchcloser totheDickey-Pkllerdistribution for negative moving average parameters I—- .5 end -.8,although
the size is too smell for these cases.The second thing to note about tables la and lb is that estimating a movingaverage parameter along with the unit root changes the behavior of thesampling distribution Lot the test tatistic. This is interesting becauseDickey and Fuller show that asymptotically the unit root test r is notaffected byestimation of higher order autoregressive parameters. Said andDickey(1985) show that the asymptotic behavior of the unit root teat shouldnot be affected by the estimation of moving everage parameters when only oneiterative step is taken from the unit root. For positive values of the movingaverage parameter 9. the size of the AItIMA(l.0.l) test is above the nominalsize based on the Dickey-Fuller distribution. This difference is largest forboth small (T25 or 50) end lstge (T—500 or 1000) sample sizes, with the sizebeing closest for moderate sample sizes (1—100 or 250). The apparent lack ofconvergence to the Dickey-Fuller statistic as the sample size grows contrastswith the results of Said end Dickey(l985) who examine samples of 49and99observations. Apparently, the distinction between the one-step methodproposed by Said and Dickey versus the iterative estimation used in theseexperiments is important.The testsbasedDickey-Fulleron the 14-ordar autoregressive model era close to theresults for values of the moving average parameter 9 equal.5, 0. -.5, or - .8.toWith 9 equal to .8, however, the AR(14) approximation isdeficient in that the size of the test is well abovethe nominalsize uaingthe Dickay-Fliller distribution. although this problem seems to be reduced asthe ssmple size grows.The size estimates based on the 112-order sutoregressive model era closerto the nominal size than for the AR(14) model.9Theonly.notabledeparture
from the Dickey-Fullet results is for 8 equal to 8. In this case, with seal!sample sites (T—25) the size of the AR(112) test is below the nominal sizebased on the Dickey-Fuller distribution.Tables 2a and 2b contain estimates of the size of unit root tests at tha liand 5% levels, respectively, where the alternative hypothesis is a stationaryARNA process around a time trend. As noted by Dickey and Fuller, including atime trend causes the critical values of r to be lower than c (i.e., thetpregression t-stsristic must ba more negative to reject the unit roothypotheais). Nevertheless, the relative patterns in Tables la and lb arerepeated in Tables 2a and lb. For example, the sizes of the ARIMA(l,0,l)testand of the AR(14) test are above the nominal size based on the Dickey-Fullercritical values for 6—. 8. As in Tables la and lb, the higher orderautoregressive approximation AR(212) has aize close to the nominal level forsample sites greater than 50. The Phillips-Perron tests bsve sizes that arefurthest from the nominal size, with the largest departures for cases where 8is positive. In fact, with 6—8,thePhillipe-Perron tests reject a unit rootover 99% of the time for a nominal la level rest for sample sizes greater than50.Thus, a low order autoregressive approximation can lead to siisspecificationof unitroottests when the moving average parameter is large. Higher orderAR processes seem to mitigate the problem (although the order of the ARprocess necesssry to provide an adequate approximation can be quite large for6—.8 or higher). Unit root tests based on the mixed JiRIMA(l,O,l) modelrequire moderate sample aizea before the Dickey-Fuller frsctiles are accurate.10
3.2 The Distribution of the Normalized Unit Root EstisatorbasedTables 3s and 3b contain estimates of the size of teatson thenormelized unit root estimator TC?M l) at the 1% and 51 levels, respectively.Six different tests are considered (AR(l);Phillips-Ferron with14 lags.1(24); Phillips-Ferron 12 legs. z 'l2 ARII4A(l.0.1); ARC!4)correctedusing the estimated value of the autoregressive parameters; and AR(112)correctedusing the estimated value of the autoregressive parameters), for thesix different sample sizes (T —25,50, 100, 250. 500, and 1000), and for the—five different values of the moving average parameter (e. B,.5, 0. -.5,and-8), where the alternative hypothesis is a stationary ARMA process around aconstant mean.In many wsys the results in Tables 3m and 3h are easier to summarize thenthe results in Tsblae la, lb. 2a and 2b. For the ARC1) modal, the estimatedsize is above the nominal level for 6 equal to.8 and .5.and the differenceincreases with the sample size. The corrections suggested by Phillips andPerron(l988) do not reduce this problem much. end the useharmstheofmore lags 112performsnce of the test in this case.The results for the ARIMA(l,0,l) model are interesting. For negativesire is close to the nominal size from thevalues of 6.thedistributionfor all sample sizes. For positive values of 6,Dickey-Fullertheestimatedaize is higher than the nominal size for all sample sizes. Unfortunately, Iof this test, T(l-I)(-l). but such adid not compute the 'corrected' versioncorrection would probablyhaveimproved the performance of this testsubstantially.yields estimates of the size that are syatematicallyto the moving average parameter, 6. Higher values of I yield lowerThe 41(14) testrelated11
estimates oftheunit root, so the AR(4)size estimatesarenominal size based on the Dickey-Fuller distribution whenARC!4)aresizereducedestimates are too low when 8 equals -.5 or - .8.well above theequals .8. TheThese problemsfor larger sample sizes.The AR(112) tact is better than the AR(!4) test for larger sample sizes,but worse for smaller sample sizes. For small sample sizes (25 and SO), thelarger number of parameters that must be estimated in the AR(112) modelapparently bias the unit root estimator downward. Note that even when themoving average parameter 8 equals zero, so the true process is a random walkas originally assumed by Dickey end Fuller, the estimated size for the Afl112)test is well above the nominal size of the test. For large samples (T—250 orabove), the sizes sre closer to the nominal level of the tests, although theyere still too high.Tables 4a end Ab contain estimates of the size of tests based on thenormalized unit root estimator T(-l) at the 1% end 5% levels, respectively,where the alternative hypothesis is a stationary ARM process around e timetrend. The relative patterns in Tables 4a and 4b are virtually identical tothose in Tables 3s snd 3b. As noted by FullerCi9l6), the size of the DickeyFuller tests is related to the moving average parameter 8. When 8—.8, theestimated size is far above thenominallevel of the test. The correctionssuggested by Fuller stabilize the behavior of the statistic for differentvalues of 8, although the size of these tests is above the nominal size usingtheDickey-Fuller distribution. The corrections suggested by PhillipsandPerron(1988) do not work as well since the estimated size remains well abovethenominal size for positive values of I.The effects of .odel miespecification ate clearer in the normalized bias12
teats (Tables 3a 3b, 4a and 4h) than in the t-teats (Tables is, lb, 2a and2b). When the data are generated by an integrated moving average process,high order autoregressive approximations yield biased eatimates of the unitroot coefficientcoefficientsunitrootWith positive moving average parametera the unit rootare too small, and with negative moving average parameters thecoefficients are toolarge.Even though the results of Dickey-Fuller(1979) suggest that T(-l) provides a more powerful teat than the rstatistic when pci, the results above suggest than the r andare lesssensitivenormalized unitto model misspscificationroot estimatorsuggested byrstatisticsThe corrections to thePhillips(l987)and Phillips endPerron(l988) do not work well in the cases exaained here. The correctionssuggested by kuller(1976) improve the behavior of the normalized unit roottestfor high orderthey distort theautoregressive models with verysize of the test in smalllarge eeisple sizes, butto moderatesesiplea.3.3 Further Analysis of the Phillips and Perron TestsThe Phillips(1987) and Phillips and Perron(l988) tests perform poorly incases where the true data are generated by an ARIMA(0,l,l) processes with 8—.5ort—.8. This has been documented earlier by Monte Carlo experiments inPerron's dissertstion(l9gia), although the extent of the problem wasclear in his work.postdatednot asPhillips end Perron(l988), in Monte Carlo work thatthis psper, findresults thatare aimiiarto the reeults above. Itis aurprising with sample sites as large as 500 or 1000that these tests arenot close to the Dickey-Puller distribution, as they should be in 'largesamples.'To provide further insight into this problem, additional Monte Carloexperiments are prformed to enelyze the Phillips-Perron tests, 2(t)13and
Z(1) .with 0—. 8The procedure discussed above is used, except only the caseis considered. Sample sizes of T—l,000 and T—10,000 are used. The number ofresidual autocorrelations used to calculate the variance s4, in (6)isandvaried from 0 (no edjuetuient) to 112 (14—7 and 1l2 2l when T—1,000 14—12 12 SI when T—l0.000). Table Iacontains the 5% and 1% fractilee of thesampling distributions from 10.000 replications for the Phillips-Patrontest.1(1). Teble Sb contains the 5% and 1% fractiles of the samplingdistributions froe 10.000 replications for the Phillips-Perron test, Z(1).Tables 5a and Sb also contain the estimated size of the 5% and It level testsin parentheses below the estimated critical values.There are two questions about the best way to do the Phillips-Perrontests.First, there is a question of the number of lags of the residualautocorrelations I to use. Second, there is a question about the way toeatieate the variances2 and suTI-If the unit root estimate is equal to its true value, 5M'the residualautocorrelations should equal -0.49 at lag I and 0.0 at the remaining lags.relatively lowFor the data generating process used in these simulations, anumber of lags should work best. Thus, Tables Sa and Sb show values of thewhere 1—0 is thePhillips-Perron teats based on 1—0, 1, 2, 3, 4, 14 and 'p12'original Dickey-Fuller statistic.Phillips and Parron suggest two strategies for estimating2 and a2TIu.the variancesThe technique used in the simulations above is based onresiduals from the estimate of (4), which is the procedurerecommended in thefirat draft of the Pbillips-Perrofl paper. The alternative procedureassume the autoregressive parameter p equals one,is toand use the diffarencas Wfto calculate the variance estimates (a procedure also diacussed by Phillips14
and Parron). This distinction is important because the autocorrelations oftha residuals are not similar to the autocorrelerions of the differences when9— .8. Because the estimate of the unit rootpis well below one in mostcases when 8—. 8, the residual autocorrelation at leg 1 averages - .36? whent—1,000, and the remaining autocorrelations are positive end decay very slowly(from .071 atlag 2to .060 at leg 21). This is typical of a mixedARXMA(l,O2) process with an autoregressive coefficient close to unity. Foren ARIMk(l.0,2) model, the kth autocorreletion—9k2whereis theautocorreletion at lag k and is the autoregressive perameter. Based on theestimates r2 — .071 and r21 —.060. the implied value of is .99. Thesepositive residual eutocorrelations cause the Phillips-Parrots tests to growfarther from the Dickey-Fuller distribution as more lags are included. Thus,the two-step procedure recommended by Phillips end Perron seams to have animportant flaw: the estimate of the autoregressive rootin (4) is biasedsubstantially below one when 9.8. so the residusls from (4) retain much ofthe nonstationarity from the original series.In contrast, the average mutocorreistion of the differences squals - .486 atlag 1, and equals .000 at all reesining legs when T—l,000. Nevertheless, theperformance of the Phillips-Perron tests based on differences in Tables Be andSbseems to improve as the number of lags increases. This is probably due tothm Nawey-West weighting scheme used to(6). which gives greeter weight toof lags increases.thecalculatethe variance estimateineutocorrelatiosiat lag 1 as the numberThe results for samples of 10,000 observationa in Tables Ba and Sbarecloser to the Dickey.Puller distribution than the results for samples of 1,000observations but the rate of convergence seems vmry slow. Finally, with15
samples oftheT—lO,000. using residuals to calculate the variance estimates,Phillipa.Petron test based on .1412 lags exhibitsunusual behavior. Forexample, the .05 critical values for Z(l) Ia above the Dickey-Fuhlercritical value, although the .01 crItical value is below the Dicksy.Fullervalue,Eased on the results in Tables Sa and Sb, the sire of the Phillipa.Perrontests is better specified when using differences tOcalculateeatisiatee if —.E, although the Said-Dickey teats arethe variancecloser to the Dlckey-Fuller distribution. One should be cautious, however, before concluding thatone should always use differencee In. the Phillips-Perrontest. In discussingthe sultivarlate analog to the Phillips.Ferron teat Z(1). Stock andWetson(l987) show that this teat is not consistent versus some stationaryalternative hypotheses when using the differences to calculate the varianceestimates. Thus, the rhillips-Perron teats using residualsbehave poorlyunder the null hypothesis. but the tests based on the differences behavepoorly under some plausible alternative hypotheses.4. SUMMARIThe ARINA(l.0,l) process used in the Monte Carlo experiments approaches astationary random process ea the moving average parameter I approaches theautoregressiva parameter p. For cases where p is close to or equal to one,and B is leas than but close to p, the autocorrelations ofpositive numbers that decay very slowly. These cases occurthe date are smellfrequently ineconomic date. For example. Nelson and Schwert(lS?l) find the .onthlyC.P.I.inflation rats for the U.S. follows much a process Hubersian and Schwert(l985)findthatthe monthly Israeli C.P.I. inflation rats follows such a process;Franch, Schwert and Stambsugh(199?) find that the log of16monthly stock market
volatility follows such a process. Schwert(1987) applies the unit root testsdiscussed in this paper to 11 l.eportant iS, macroeconomic time series andconcludes that many of the tests would falsely reject the untt root hypothesisusing the Dickey-Fuller critical values. In euch cases, the cosason argumentthat the unit root in the autoregressive part of the model dominates theasymptotic behavior of the process is misleading for large finite samples.The simulations in this paper chow that the tests for unit roots developedby Dickey and Fuller are sensitive to the assumption that the date aregenerated by e pure autoregressive process. When the underlying processa moving average component, the distribution of the unit root testcontainsstatisticscan be far different from the distributions reported by Dickey andFuller. Moreover, the tests recently suggested by Said and Dickey(l984,1965),Phillips(l987) and Phillips and PerronCl9BS) tomisspecificationproblem do not seem to workcorrect themodelwell when the moving averageis large. In particular, the tests proposed by Phillips and Perrondo not come close to their asymptotic distribution for samples as large asparameter10,000 observations. The best test, in the sense that it hasitssize close tonominal level for all values of the moving average parameter 8, isSaidtheand Dickey(l984) high order autoregressive 'ft-teat"
William E. Simon Graduate School of Business Administration University of Rochester Rochester, NY 14627. 1. INTRODUCTION Fuller(1976) and Dickey and Fuller(1919,1981) develop several tests of whether a th order autoregressive (AR) process .