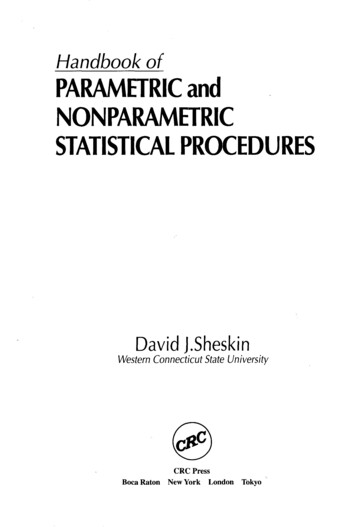
Transcription
Handbook ofPARAMETRIC andNONPARAMETRICSTATISTICAL PROCEDURESDavid J.SheskinWestern Connecticut State UniversityBoca RatonCRC PressNew York LondonTokyo
Table of Contentswith Summary of TopicsIntroductionDescriptive versus inferential statistics; Statistic versus parameter; Levels ofmeasurement; Continuous versus discrete variables; Measures of central tendency;Measures of variability; The normal distribution; Hypothesis testing; Type I andType II errors in hypothesis testing; Estimation in inferential statistics; Basicconcepts and terminology employed in experimental design; Correlational research;Parametric versus nonparametric inferential statistical tests; Selection of the appropriate statistical procedureOutline of Inferential Statistical Tests and Measures ofCorrelation/Association23Guidelines and Decision Tables for Selecting the AppropriateStatistical Procedure27Inferential Statistical Tests Employed with a Single Sample31Test 1. The Single-Sample z TestI.II.III.IV.V.VI.Hypothesis Evaluated with Test and Relevant Background InformationExampleNull versus Alternative HypothesesTest ComputationsInterpretation of the Test ResultsAdditional Analytical Procedures for the Single-Sample z Test and/orRelated TestsVII. Additional Discussion of the Single-Sample z Test1. The interpretation of a negative z value2. The standard error of the population mean and graphical representation of results of the single-sample z test3. Additional examples illustrating the interpretation of a computed zvalue4. The z test for a population proportionVIII. Additional Examples Illustrating the Use of the Single-Sample z Test33
Test 2. The Single-Sample / Test47I.II.III.IV.V.VI.Hypothesis Evaluated with Test and Relevant Background InformationExampleNull versus Alternative HypothesesTest ComputationsInterpretation of the Test ResultsAdditional Analytical Procedures for the Single-Sample t Test and/orRelated Tests1. Determination of the power of the single-sample t test and thesingle-sample z test2. Computation of a confidence interval for the mean of a populationrepresented by a sampleVII. Additional Discussion of the Single-Sample t Test1. Degrees of freedomVIII. Additional Examples Illustrating the Use of the Single-Sample t TestTest 3. The Single-Sample Chi-Square Test for a Population VarianceHypothesis Evaluated with Test and Relevant Background InformationExampleNull versus Alternative HypothesesTest ComputationsInterpretation of the Test ResultsAdditional Analytical Procedures for the Single-Sample Chi-Square Testfor a Population Variance and/or Related Tests1. Large sample normal approximation of the chi-square distribution2. Computation of a confidence interval for the variance of a population represented by a sample3. Computation of the power of the single-sample chi-square test fora population varianceVII. Additional Discussion of the Single-Sample Chi-Square Test for a Population VarianceVIII. Additional Examples Illustrating the Use of the Single-Sample ChiSquare Test for a Population Variance71I.II.III.IV.V.VI.Test 4. The Wilcoxon Signed-Ranks TestI.II.III.IV.V.VI.Hypothesis Evaluated with Test and Relevant Background InformationExampleNull versus Alternative hypothesesTest ComputationsInterpretation of the Test ResultsAdditional Analytical Procedures for the Wilcoxon Signed-Ranks Testand/or Related Tests1. The normal approximation of the Wilcoxon T statistic for largesample sizes2. The correction for continuity for the normal approximation of theWilcoxon signed-ranks test\83
3. Tie correction for the normal approximation of the. Wilcoxon teststatisticVII. Additional Discussion of the Wilcoxon Signed-Ranks TestVIII. Additional Examples Illustrating the Use of the Wilcoxon Signed-RanksTestTest 5. The Chi-Square Goodness-of-Fit Test95I.II.III.IV.V.VI.Hypothesis Evaluated with Test and Relevant Background InformationExampleNull versus Alternative hypothesesTest ComputationsInterpretation of the Test ResultsAdditional Analytical Procedures for the Chi-Square Goodness-of-FitTest and/or Related Tests1. Comparisons involving individual cells when k 22. The analysis of standardized residuals3. Computation of a confidence interval for the chi-square goodnessof-fit test4. Brief discussion of the z test for a population proportion and thesingle-sample test for the median5. The correction for continuity for the chi-square goodness-of-fit test6. Sources for computing of the power of the chi-square goodness-offit testVII. Additional Discussion of the Chi-Square Goodness-of-Fit Test1. Directionality of the chi-square goodness-of-fit test2. Modification of procedure for computing the degrees of freedom forthe chi-square goodness-of-fit test3. Additional goodness-of-fit testsVIII. Additional Examples Illustrating the Use of the Chi-Square Goodness-ofFit TestTest 6. The Binomial Sign Test for a Single SampleI.II.III.IV.V.VI.Hypothesis Evaluated with Test and Relevant Background InformationExampleNull versus Alternative HypothesesTest ComputationsInterpretation of the Test ResultsAdditional Analytical Procedures for the Binomial Sign Test for a SingleSample and/or Related Tests1. Test (a: The z test for a population proportion (with discussionof correction for continuity, computation of a confidence interval;extension of the z test for a population proportion in order to evaluate, the performance of m subjects on n trials on a binomiallydistributed variable)2. Test 6b: The single-sample test for the median3. Sources for computing the power of the binomial sign test for singlesample113
VII. Additional Discussion of the Binomial Sign Test for a Single SampleVIII. Additional Example Illustrating the Use of the Binomial Sign Test fora Single SampleTest 7. The Single-Sample Runs Test (and Other Tests of Randomness)135I.II.III.IV.V.VI.Hypothesis Evaluated with Test and Relevant Background InformationExampleNull versus Alternative HypothesesTest ComputationsInterpretation of the Test ResultsAdditional Analytical Procedures for the Single-Sample Runs Testand/or Related Tests1. The normal approximation of the single-sample runs test for largesample sizes2. The correction for continuity for the normal approximation of thesingle-sample runs testVII. Additional Discussion of the Single-Sample Runs Test1. Alternative tests of randomness (The frequency test; the gap test;the poker test; autocorrelation; the serial test; the coupon collector'stest; Von Neumann ratio test on independence/mean square successive difference test; test of trend analysis/time series analysis)VIII. Additional Examples Illustrating the Use of the Single-Sample Runs TestInferential Statistical Tests Employed with Two IndependentSamples (and Related Measures of Association/Correlation)Test 8. The / Test for Two Independent SamplesI.II.III.IV.V.VI.Hypothesis Evaluated with Test and Relevant Background InformationExampleNull versus Alternative HypothesesTest ComputationsInterpretation of the Test ResultsAdditional Analytical Procedures for the t Test for Two IndependentSamples and/or Related Tests1. The equation for the t test for two independent samples when avalue for a difference other than zero is stated in the null hypothesis2. Test 8a: Hartley's Fmax test for homogeneity of variance/F testfor two population variances: Evaluation of the homogeneity ofvariance assumption of the t test for two independent samples3. Computation of the power of the t test for two independent samples4. Measure of magnitude of treatment effect for the t test for twoindependent samples: Test 8b: Omega squared5. Computation of a confidence interval for the t test for two independent samples6. Test 8c: The z test for two independent samples151153
VII. Additional Discussion of the t Test for Two Independent Samples1. Unequal sample sizes2. Outliers3. Robustness of the t test for two independent samplesVIII. Additional Examples Illustrating the Use of the t Test for Two Independent SamplesTest 9. The Mann-Whitney U Test181I.II.III.IV.V.VI.Hypothesis Evaluated with Test and Relevant Background InformationExampleNull versus Alternative HypothesesTest ComputationsInterpretation of the Test ResultsAdditional Analytical Procedures for the Mann-Whitney U Test and/orRelated Tests1. The normal approximation of the Mann-Whitney U statistic forlarge sample sizes2. The correction for continuity for the normal approximation of theMann-Whitney U test3. Tie correction for the normal approximation of the Mann-WhitneyU statistic4. Sources for computing a confidence interval for the Mann-WhitneyU testVII. Additional Discussion of the Mann-Whitney U Test1. Power efficiency of the Mann-Whitney U test2. Alternative nonparametric rank-order procedures for evaluating adesign involving two independent samplesVIII. Additional Examples Illustrating the Use of the Mann-Whitney [/TestTest 10. The Siegel-Tukey Test for Equal VariabilityI.II.III.IV.V.VI.Hypothesis Evaluated with Test and Relevant Background InformationExampleNull versus Alternative HypothesesTest ComputationsInterpretation of the Test ResultsAdditional Analytical Procedures for the Siegel-Tukey Test for EqualVariability and/or Related Tests1. The normal approximation of the Siegel-Tukey test statistic forlarge sample sizes2. The correction for continuity for the normal approximation of theSiegel-Tukey test for equal variability3. Tie correction for the normal approximation of the Siegel-Tukeytest statistic4. Adjustment of scores for the Siegel-Tukey test for equal variabilitywhen 0i * 62195
VII. Additional Discussion of the Siegel-Tukey Test for Equal Variability1. Analysis of the homogeneity of variance hypothesis for the same setof data with both a parametric and nonparametric test2. Alternative nonparametric tests of dispersionVIII. Additional Examples Illustrating the Use of the Siegel-Tukey Test forEqual VariabilityTest 11. The Chi-Square Test for r x c Tables [Test l l a : The Chi-SquareTest for Homogeneity; Test l i b : The Chi-Square Test ofIndependence (employed with a single sample)]I.II.III.IV.V.VI.209Hypothesis Evaluated with Test and Relevant Background InformationExampleNull versus Alternative HypothesesTest ComputationsInterpretation of the Test ResultsAdditional Analytical Procedures for the Chi-Square Test for r X cTables and/or Related Tests1. Yates' correction for continuity2. Quick computational equation for a 2 X 2 table3. Evaluation of a directional alternative hypothesis in the case of a2 x 2 contingency table4. Test l i e : The Fisher exact test5. Test l i d : The z test for two independent proportions6. Computation of a confidence interval for a difference between proportions7. Test l i e : The median test for independent samples8. Extension of the chi-square test for r X c tables to contingencytables involving more than two rows and/or columns, and associatedcomparison procedures9. The analysis of standardized residuals10. Sources for the computation of the power of the chi-square test forr X c tables11. Measures of association for r X c contingency tables (Test llf:The contingency coefficient; Test l l g : The phi coefficient; Testl l h : CrameVs phi coefficient; Test Hi: Yule's Q; Test l l j : Theodds ratio)VII. Additional Discussion of the Chi-Square Test for r X c Tables1. Analysis of multidimensional contingency tablesVIII. Additional Examples Illustrating the Use of the Chi-Square Test forr X c TablesInferential Statistical Tests Employed with Two DependentSamples (and Related Measures of Association/Correlation)Test 12. The / Test for Two Dependent SamplesI. Hypothesis Evaluated with Test and Relevant Background InformationII. ExampleIII. Null versus Alternative Hypotheses257259
IV. Test ComputationsV. Interpretation of the Test ResultsVI. Additional Analytical Procedures for the t Test for Two DependentSamples and/or Related Tests1. Alternative equation for the t test for two dependent samples2. The equation for the t test for two dependent samples when a valuefor a difference other than zero is stated in the null hypothesis3. Test 12a: The t test for homogeneity of variance for twodependent samples: Evaluation of the homogeneity of varianceassumption of the t test for two dependent samples4. Computation of the power of the t test for two dependent samples5. Measure of magnitude of treatment effect for the t test for twodependent samples: Omega squared (Test 12b)6. Computation of a confidence interval for the t test for two dependent samples7. Test 12c: Sandler's A test8. Test 12d: The z test for two dependent samplesVII. Additional Discussion of the t Test for Two Dependent Samples1. The use of matched subjects in a dependent samples design2. Relative power of the t test for two dependent samples and thet test for two independent samples3. Counterbalancing and order effects4. Analysis of a before-after design with the t test for two dependentsamplesVIII. Additional Example Illustrating the Use of the t Test for Two Dependent SamplesTest 13. The Wilcoxon Matched-Pairs Signed-Ranks TestI.II.III.IV.V.VI.Hypothesis Evaluated with Test and Relevant Background InformationExampleNull versus Alternative HypothesesTest ComputationsInterpretation of the Test ResultsAdditional Analytical Procedures for the Wilcoxon Matched-PairsSigned-Ranks Test and/or Related Tests1. The normal approximation of the Wilcoxon T statistic for largesample sizes2. The correction for continuity for the normal approximation of theWilcoxon matched-pairs signed-ranks test3. Tie correction for the normal approximation of the Wilcoxon teststatistic4. Sources for computing a confidence interval for the Wilcoxonmatched-pairs signed-ranks testVII. Additional Discussion of the Wilcoxon Matched-Pairs Signed-Ranks Test1. Alternative nonparametric rank-order procedures for evaluating adesign involving two dependent samplesVIII. Additional Examples Illustrating the Use of the Wilcoxon Matched-PairsSigned-Ranks Test291
Test 14. The Binomial Sign Test for Two Dependent Samples303I. Hypothesis Evaluated with Test and Relevant Background InformationII. ExampleIII. Null versus Alternative HypothesesIV. Test ComputationsV. Interpretation of the Test ResultsVI. Additional Analytical Procedures for the Binomial Sign Test for TwoDependent Samples and/or Related Tests1. The normal approximation of the binomial sign test for two dependent samples with and without a correction for continuity2. Computation of a confidence interval for the binomial sign test fortwo dependent samples3. Sources for computing the power of the binomial sign test for twodependent samplesVII. Additional Discussion of the Binomial Sign Test for Two DependentSamples1. The problem of an excessive number of zero difference scores2. Equivalency of the Friedman two-way analysis variance by ranksand the binomial sign test for two dependent samples when k 2VIII. Additional Examples Illustrating the Use of the Binomial Sign Test forTwo Dependent SamplesTest 15. The McNemar TestI. Hypothesis Evaluated with Test and Relevant Background InformationII. ExampleIII. Null versus Alternative HypothesesIV. Test ComputationsV. Interpretation of the Test ResultsVI. Additional Analytical Procedures for the McNemar Test and/or RelatedTests1. Alternative equation for the McNemar test statistic based on thenormal distribution2. The correction for continuity for the McNemar test3. Computation of the exact binomial probability for the McNemartest model with a small sample size4. Additional analytical procedures for the McNemar testVII. Additional Discussion of the McNemar Test1. Alternative format for the McNemar test summary table andmodified test equation2. Extension of McNemar test model beyond 2 x 2 contingency tablesVIII. Additional Examples Illustrating the Use of the McNemar Test315
Inferential Statistical Tests Employed with Two or MoreIndependent Samples (and Related Measures ofAssociation/Correlation)331Test 16. The Single-Factor Between-Subjects Analysis of Variance333I.II.III.IV.V.VI.Hypothesis Evaluated with Test and Relevant Background InformationExampleNull versus Alternative HypothesesTest ComputationsInterpretation of the Test ResultsAdditional Analytical Procedures for the Single-Factor Between-SubjectsAnalysis of Variance and/or Related Tests1. Comparisons following computation of the omnibus F value for thesingle-factor between-subjects analysis of variance (Planned versusunplanned comparisons; Simple versus complex comparisons;Linear contrasts; Orthogonal comparisons; Test 16a: Multiple/tests/Fisher's LSD test; Test 16b: The Bonferroni-Dunntest; Test 16c: Tukey's HSD test; Test 16d: The NewmanKeuls test; Test 16e: The Scheffe* test; Test 16f: The Dunnetttest; Additional discussion of comparison procedures and finalrecommendations; The computation of a confidence interval for acomparison)2. Comparing the means of three or more groups when k 43. Evaluation of the homogeneity of variance assumption of the singlefactor between-subjects analysis of variance4. Computation of the power of the single-factor between-subjectsanalysis of variance5. Measures of magnitude of treatment effect for the single-factorbetween-subjects analysis of variance: Test 16g: Omega squaredand Test 16h: Eta squared6. Computation of a confidence interval for the mean of a treatmentpopulation7. The analysis of covarianceVII. Additional Discussion of the Single-Factor Between-Subjects Analysisof Variance1. Theoretical rationale underlying the single-factor between-subjectsanalysis of variance2. Definitional equations for the single-factor between-subjects analysisof variance3. Equivalency of the single-factor between-subjects analysis of variance and the t test for two independent samples when k - 24. Robustness of the single-factor between-subjects analysis of variance5. Fixed-effects versus random-effects models for the single-factorbetween-subjects analysis of varianceVIII. Additional Examples Illustrating the Use of the Single-Factor BetweenSubjects Analysis of Variance
Test 17. The Kruskal-Wallis One-Way Analysis of Variance by Ranks397I.II.III.IV.V.VI.Hypothesis Evaluated with Test and Relevant Background InformationExampleNull versus Alternative HypothesesTest ComputationsInterpretation of the Test ResultsAdditional Analytical Procedures for the Kruskal-Wallis One-WayAnalysis of Variance by Ranks and/or Related Tests1. Tie correction for the Kruskal-Wallis one-way analysis of varianceby ranks2. Pairwise comparisons following computation of the test statistic forthe Kruskal-Wallis one-way analysis of variance by ranksVII. Additional Discussion of the Kruskal-Wallis One-Way Analysis ofVariance by Ranks1. Exact tables of the Kruskal-Wallis distribution2. Equivalency of the Kruskal-Wallis one-way analysis of variance byranks and the Mann-Whitney U test when k 23. Alternative nonparametric rank-order procedures for evaluating adesign involving k independent samplesVIII. Additional Examples Illustrating the Use of the Kruskal-Wallis OneWay Analysis of Variance by RanksInferential Statistical Tests Employed with Two or MoreDependent Samples (and Related Measures ofAssociation/Correlation)411Test 18. The Single-Factor Within-Subjects Analysis of Variance413I.II.III.IV.V.VI.Hypothesis Evaluated with Test and Relevant Background InformationExampleNull versus Alternative HypothesesTest ComputationsInterpretation of the Test ResultsAdditional Analytical Procedures for the Single-Factor Within-SubjectsAnalysis of Variance and/or Related Tests1. Comparisons following computation of the omnibus F value forthe single-factor within-subjects analysis of variance (Test 18a:Multiple t tests/Fisher's LSD test; Test 18b: The BonferroniDunn test; Test 18c: Tukey's HSD test; Test 18d: The NewmanKeuls test; Test 18e: The Scheffe" test; Test 18f: The Dunnetttest; The computation of a confidence interval for a comparison;Alternative methodology for computing MSm for a comparison)2. Comparing the means of three or more conditions when k 43. Evaluation of the sphericity assumption underlying the single-factorwithin-subjects analysis of variance4. Computation of the power of the single-factor within-subjectsanalysis of variance5. Measure of magnitude of treatment effect for the single-factorwithin-subjects analysis of variance: Test 18g: Omega squared
6. Computation of a confidence interval for the mean of a treatmentpopulationVII. Additional Discussion of the Single-Factor Within-Subjects Analysis ofVariance1. Theoretical rationale underlying the single-factor within-subjectsanalysis of variance2. Definitional equations for the single-factor within-subjects analysisof variance3. Relative power of the single-factor within-subjects analysis ofvariance and the single-factor between-subjects analysis of variance4. Equivalency of the single-factor within-subjects analysis of varianceand the t test for two dependent samples when k 2VIII. Additional Examples Illustrating the Use of the Single-Factor WithinSubjects Analysis of VarianceTest 19. The Friedman Two-Way Analysis of Variance by Ranks453I.II.III.IV.V.VI.Hypothesis Evaluated with Test and Relevant Background InformationExampleNull versus Alternative HypothesesTest ComputationsInterpretation of the Test ResultsAdditional Analytical Procedures for the Friedman Two-Way AnalysisVariance by Ranks and/or Related Tests1. Tie correction for the Friedman two-way analysis variance by ranks2. Pairwise comparisons following computation of the test statistic forthe Friedman two-way analysis of variance by ranksVII. Additional Discussion of the Friedman Two-Way Analysis Variance byRanks1. Exact tables of the Friedman distribution2. Equivalency of the Friedman two-way analysis variance by ranksand the binomial sign test for two dependent samples when k 23. Alternative nonparametric rank-order procedures for evaluating adesign involving k dependent samples4. Relationship between the Friedman two-way analysis of variance byranks and Kendall's coefficient of concordanceVIII. Additional Examples Illustrating the Use of the Friedman Two-WayAnalysis of Variance by RanksTest 20. The Cochran Q TestI.II.III.IV.V.VI.Hypothesis Evaluated with Test and Relevant Background InformationExampleNull versus Alternative HypothesesTest ComputationsInterpretation of the Test ResultsAdditional Analytical Procedures for the Cochran Q Test and/or RelatedTests1. Pairwise comparisons following computation of the test statistic forthe Cochran Q test469
VII. Additional Discussion of the Cochran Q Test1. Issues relating to subjects who obtain the same score under all ofthe experimental conditions2. Equivalency of the Cochran Q test and the McNemar test whenk 23. Alternative nonparametric procedures for categorical data forevaluating a design involving k dependent samplesVm. Additional Examples Illustrating the Use of the Cochran Q TestInferential Statistical Test Employed with Factorial Designs(and Related Measures of Association/Correlation)Test 21. The Between-Subjects Factorial Analysis of VarianceI.II.III.IV.V.VI.Hypothesis Evaluated with Test and Relevant Background InformationExampleNull versus Alternative HypothesesTest ComputationsInterpretation of the Test ResultsAdditional Analytical Procedures for the Between-Subjects FactorialAnalysis of Variance and/or Related Tests1. Comparisons following computation of the F values for thebetween-subjects factorial analysis of variance (Test 21a: Multiplet tests/Fisher's LSD test; Test 21b: The Bonferroni-Dunn test;Test 21c: Tukey's HSD test; Test 21d: The Newman-Keuls test;Test 21e: The Scheffe* test; Test 21f: The Dunnett test; Comparisons between the marginal means; Evaluation of an omnibushypothesis involving more than two marginal means; Comparisonsbetween specific groups that are a combination of both factors; Thecomputation of a confidence interval for a comparison; Analysis ofsimple effects)2. Evaluation of the homogeneity of variance assumption of thebetween-subjects factorial analysis of variance3. Computation of the power of the between-subjects factorial analysisof variance4. Measure of magnitude of treatment effect for the between-subjectsfactorial analysis of variance: Test 21g: Omega squared5. Computation of a confidence interval for the mean of a populationrepresented by a group6. Additional analysis of variance procedures for factorial designsVII. Additional Discussion of the Between-Subjects Factorial Analysis ofVariance1. Theoretical rationale underlying the between-subjects factorialanalysis of variance2. Definitional equations for the between-subjects factorial analysis ofvariance3. Unequal sample sizes4. Final comments on the between-subjects factorial analysis of variance (Fixed-effects versus random-effects versus mixed-effectsmodels; Nested factors/hierarchical designs and designs involvingmore than two factors)487489
VIII. Additional Examples Illustrating the Use of the Between-SubjectsFactorial Analysis of VarianceIX. Addendum (Discussion of and computational procedures for additionalanalysis of variance procedures for factorial designs: Test 21h: Thefactorial analysis of variance for a mixed design; Test 21i: Thewithin-subjects factorial analysis of variance)Measures of Association/CorrelationTest 22. The Pearson Product-Moment Correlation CoefficientI.II.III.IV.V.Hypothesis Evaluated with Test and Relevant Background InformationExampleNull versus Alternative HypothesesTest ComputationsInterpretation of the Test Results (Test 22a: Test of significance fora Pearson product-moment correlation coefficient; The coefficient ofdetermination)VI. Additional Analytical Procedures for the Pearson Product-MomentCorrelation Coefficient and/or Related Tests1. Derivation of a regression line2. The standard error of estimate3. Computation of a confidence interval for the value of the criterionvariable4. Computation of a confidence interval for a Pearson product-momentcorrelation coefficient5. Test 22b: Test for evaluating the hypothesis that the truepopulation correlation is a specific value other than zero6. Computation of power for the Pearson product-moment correlationcoefficient:7. Test 22c: Test for evaluating a hypothesis on whether there isa significant difference between two independent correlations8. Test 22d: Test for evaluating a hypothesis on whether k independent correlations are homogeneous9. Test 22e: Test for evaluating the null hypothesis Ho: pxz - pyz10. Tests for evaluating a hypothesis regarding one or more regressioncoefficients (Test 22f: Test for evaluating the null hypothesisi/ 0 : 0 0; Test 22g: Test for evaluating the null hypothesis11. Additional correlational proceduresVII. Additional Discussion of the Pearson Product-Moment CorrelationCoefficient1. The definitional equation for the Pearson product-moment correlation coefficient2. Residuals3. Covariance4. The homoscedasticity assumption of the Pearson product-momentcorrelation coefficient5. The phi coefficient as a special case of the Pearson product-momentcorrelation coefficient6. Autocorrelation/serial correlation537539
VIII. Additional Examples Illustrating the Use of the Pearson Product-MomentCorrelation CoefficientIX. Addendum1. Bivariate measures of correlation that are related to the Pearsonproduct-moment correlation coefficient (Test 22h: The pointbiserial correlation coefficient (and Test 22h-a: Test of significance for a point-biserial correlation coefficient); Test 22i: Thebiserial correlation coefficient (and Test 22i-a: Test of significance for a biserial correlation coefficient); Test 22j: The tetrachoric correlation coefficient (and Test 25j-a: Test of significancefor a tetrachoric correlation coefficient))2. Multiple regression analysisGeneral introduction to multiple regression analysis Computationalprocedures for multiple regression analysis involving three variables(Test 22k: The multiple correlation coefficient; The coefficient ofmultiple determination; Test 22k-a: Test of significance for amultiple correlation coefficient; The multiple regression equation;The standard error of multiple estimate; Computation of a confidence interval for Y'; Evaluation of the relative importance ofthe predictor variables; Evaluating the significance of a regressioncoefficient; Computation of a confidence interval for a regressioncoefficient; Partial and semipartial correlation (Test 221: Thepartial correlation coefficient and Test 221-a: Test of significancefor a partial correlation coefficient; Test 22m: The semipartialcorrelation coefficient and Test 22m-a: Test of significance fora semipartial correlation coefficient); Final comments on multipleregression analysisTest 23. Spearman's Rank-Order Correlation CoefficientI.II.III.IV.V.Hypothesis Evaluated with Test and Relevant Background InformationExampleNull versus Alternative HypothesesTest ComputationsInterpretation of the Test Results (Test 23a: Test of significance forSpearman's rank-order correlation coefficient)VI. Additional Analytical Procedures for Spearman's Rank-Order Correlation Coefficient and/or Related Tests1. Tie correction for Spearman's rank-order correlation coefficient2. Spearman's rank-order correlation coefficient as a special case ofthe Pearson product-moment correlation coefficient3. Regression analysis and Spearman's rank-order correlation coefficient4. Partial rank correlationVII. Additional Discussion of Spearman's Rank-Order Correlation Coefficient1. The relationship between Kendall's coefficient of concordance (Test25), Spearman's rank-order correlation coefficient, and theFriedman two-way analysis of variance by ranks (Test 19)2. Power efficiency of Spearman's rank-order correlation coefficient3. Brief discussion of Kendall's tau (Test 24): An alternative measureof association for two sets of ranks609
VIII. Additional Examples Illustrating the Use of the Spearman's Rank-OrderCorrelation CoefficientTest 24. Kendall's Tau627I.II.III.IV.V.Hypothesis Evaluated with
Test 11. The Chi-Square Test for r x c Tables [Test lla: The Chi-Square Test for Homogeneity; Test lib: The Chi-Square Test of Independence (employed with a single sample)] 209 I. Hypothesis Evaluated with Test and Relevant Background Information II. Example III. Null versus Alternative Hypotheses IV. Test Computations V. Interpretation of the .