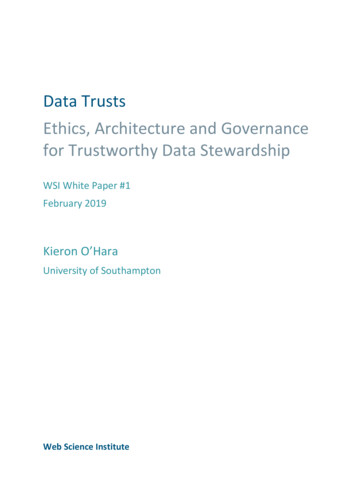
Transcription
Data TrustsEthics, Architecture and Governancefor Trustworthy Data StewardshipWSI White Paper #1February 2019Kieron O’HaraUniversity of SouthamptonWeb Science Institute
Copyright Kieron O’Hara 2019The opinions expressed in this publication are those of the author and do not necessarily reflect theviews of the University of Southampton, the Web Science Institute or its Board of Directors.This work is licensed under a Creative Commons Attribution — Non-commercial — No DerivativesLicence. To view this licence, visit (www.creativecommons.org/licenses/by-nc-nd/3.0/). For reuse ordistribution, please include this copyright notice.Web Science InstituteBuilding 32, Highfield Campus, University of Southampton, SO17 1BJwsi@soton.ac.uk
About the AuthorKieron O’Hara is an associate professor in electronics andcomputer science at the University of Southampton, UK. His interestsare in the philosophy and politics of digital modernity, particularlythe World Wide Web; key themes are trust, privacy and ethics. He isthe author of several books on technology and politics, the latest ofwhich is The Theory and Practice of Social Machines (Springer 2019,with Nigel Shadbolt, David De Roure and Wendy Hall). He has alsowritten extensively on political philosophy and British politics. He isone of the leads on the UKAN Network, which disseminates bestpractices in data anonymisation.About the WSIThe Web Science Institute (WSI) co-ordinates the University ofSouthampton’s (UoS) world-leading, interdisciplinary expertise inWeb Science, to tackle the most pressing global challenges facing theWorld Wide Web and wider society today. Research lies at its heart,positioning it as a leader in Web Science knowledge and innovationand fuelling its extensive education, training, enterprise and impactactivities. The WSI is also UoS’s main point of contact with The AlanTuring Institute, the UK’s national institute for Data Science and AI,of which UoS is a partner andimpact/policy.page
Data TrustsKieron O’Haraintended to help align trust andtrustworthiness, so we trust all and onlytrustworthy actors. The appropriate form oftrust is based not on rules, but on sociallicence to operate.Executive SummaryIn their report on the development of the UKAI industry, Wendy Hall and Jérôme Pesentirecommend the establishment of data trusts,“proven and trusted frameworks andagreements” that will “ensure exchanges [ofdata] are secure and mutually beneficial” bypromoting trust in the use of data for AI. Halland Pesenti leave the structure of data trustsopen, and the purpose of this paper is toexplore the questions of (a) what existingstructures can data trusts exploit, and(b) what relationship do data trusts have totrusts as they are understood in law?2. EthicsAn appropriate ethical regime will help createand support a social licence. Hence a datatrust must generate a meaningful ethical codefor its members. This will vary, depending onwhose trust the data trust is intended tosolicit. However, the code should constrain allwho operate within it. Hence a data trust isexpected to have a membership model, andall the members of the trust would respectthe ethical code when acting within themodel.The paper defends the following thesis:A data trust works within the law to provideethical, architectural and governancesupport for trustworthy data processingOne possible example for the foundation ofan ethical code is proposed in the paper: theAnonymisation Decision-Making Framework(ADF), proposed by UKAN.Data trusts are therefore both constrainingand liberating. They constrain: they respectcurrent law, so they cannot render currentlyillegal actions legal. They are intended toincrease trust, and so they will typically act asfurther constraints on data processors, addingthe constraints of trustworthiness to those oflaw. Yet they also liberate: if data processorsare perceived as trustworthy, they will getimproved access to data.3. ArchitectureThe data trust might not actually have anarchitecture as such – it might be merely acode of governance. However, this paperdiscusses one possible architecture, based onthe Web Observatory developed atSouthampton University, to create a DataTrust Portal. The architecture allows data tobe discovered and used, promotingaccountability and transparency, without thedata leaving the hands of data controllers. Adata trust is not a data store.Most work on data trusts has up to nowfocused on gaining and supporting the trust ofdata subjects in data processing. However, allactors involved in AI – data consumers, dataproviders and data subjects – have trustissues which data trusts need to address.Furthermore, it is not only personal data thatcreates trust issues; the same may be true ofany dataset whose release might involve anorganisation risking competitive advantage.4. Legal statusThe paper sets out the manifold reasons whya data trust cannot be a trust in a legal sense.However, it takes inspiration from the notionof a legal trust, and several instances of thisare also set out. The key issue is defining theset of beneficiaries, and defining what theirrights within the trust will be. Again, theappropriate set of beneficiaries will dependupon the set of agents whose trust is to besolicited by the data trust.The paper addresses four areas.1. Trust and trustworthiness.With regard to trust, the aims of data trustsare twofold. First, data trusts are intended todefine a certain level of trustworthybehaviour for data science. Second, they are4
Data TrustsKieron O’HaraTo conclude, data trusts could help align trustand trustworthiness via a concentration onethics, architecture and governance, allowingdata controllers to be transparent about theirprocessing and sharing, to be heldaccountable for their actions, and to engagewith the community whose trust is to beearned.5
Data TrustsKieron O’Hara(Hardinges & Wells 2018), which they basedon the notion of a literal legal trust: “a legalstructure that provides independent thirdparty stewardship of data”. A trust is a legalrelationship in which an asset is run by atrustee for the benefit of a beneficiary. Eventhough the trustee owns the asset in law, sheis not allowed to run it for her own benefit,but has a fiduciary duty to ensure that thebenefits fall to the beneficiary. The idea of adata trust, then, leans on this concept fromcommon law jurisdictions such as the UK andthe US: whoever have the rights over the datamust commit to administering the data forthe benefit of beneficiaries, rather than forthemselves. Delacroix and Lawrence (2018)argue that data trusts as Hall and Pesenticannot be literal legal trusts.IntroductionIn their report on the development of the AIindustry for the UK government, Hall andPesenti introduce the idea of a data trust as ameans of facilitating data sharing, in order tosupport industry’s, government’s andacademe’s access to the data that is the rawmaterial of AI development (Hall & Pesenti2017). They specify that data trusts should be“proven and trusted frameworks andagreements” that supply the trust that will“ensure exchanges [of data] are secure andmutually beneficial”. In the background is theunspoken assumption that the US and Chinahave the advantage of being larger marketsthan the UK (Hall and Pesenti’s focus), andless fragmented markets than the EU (Lee2018). Another assumption is that datasharing is inherently risky for a number ofreasons, including that sharing personal datamight put the interests of data subjects atrisk, exposing an organisation to a fine or toreputational damage, and that companiesmight lose trade secrets or competitiveadvantage by sharing. Hence data sharingneeds a ‘shove’ to establish the practice, anddata trusts might help to absorb at least someof the perceived risk of data sharing.In this paper, I will broadly endorse the ODIconception, while also agreeing with Delacroixand Lawrence, and look in detail at how wemight implement something like this concept,while also in passing considering the reasonsfor rejecting some of the otherinterpretations. I will also consider whattechnologies and standards might already bein place to support this implementation. Thekey thesis of this paper is:A data trust works within the law to provideethical, architectural and governancesupport for trustworthy data processingHall and Pesenti leave open the exact natureof data trusts, and define them onlyfunctionally. Hardinges (2018), in a survey ofthis nascent field for the UK Open DataInstitute (ODI), whose mission is to increasesafe data sharing and to open up as manydata stores to as much processing as isconsistent with safety, found five particularinterpretations:In particular, a data trust needs to fulfil twofunctions. First, it needs to be an arena inwhich data processing and data science cantake place transparently, allowing datacontrollers to be held accountable. On top ofthis, it should also allow data scientists tointeract and debate what constitutestrustworthy behaviour in their profession.1. A repeatable framework of terms andmechanisms.2. A mutual organisation.3. A legal structure.4. A store of data.5. Public oversight of data access.Second, the data trust also needs to be aninterface between data scientists, datasubjects and other stakeholders. This shouldallow stakeholders both to hold data scientiststo account themselves, and also to inject theirown views about what constitutestrustworthy behaviour by data scientists (i.e.The ODI researchers eventually narroweddown their quest to a single definition6
Data TrustsKieron O’Harawhat they trust data scientists to do).Delacroix and Lawrence argue that “it isunclear what, if anything, such frameworkshave in common with the Trust structures”that we find in English law (2018), but I willargue in the course of this paper that datatrusts can take quite a lot of inspiration from,even if they cannot actually be, legal trusts.data is processed, i.e. exercises overallcontrol. The trust issues that arise in datasharing are not restricted to the sharing ofpersonal data; non-personal data can besensitive too, if for different reasons. In thispaper, I will use the term ‘data controller’loosely to mean whoever exercises controlover any dataset in a data trust, whether ornot it is personal data, and consequently,whenever I refer to data or datasets, I makeno assumption that the data is personal dataunless stated explicitly. However, if I refer tothe data subjects of a dataset, naturally thatimplies that the dataset contains personaldata.We also should note the long list of agentswho have a need for trust. Data controllersneed to trust that their data will not bemisused by data users. Data users need totrust that the data they get access to is of highquality and good provenance. Data subjectsneed to trust that data about them will not beused to harm (or even to irritate) them. Andall data scientists need to trust thatuntrustworthy practices will be stamped out –trust in data science as a whole suffers witheach Cambridge Analytica story. The datatrust is not just about the trust of datasubjects, but of many more. It follows thatthere is no ‘one size fits all’ data trust, but arange of models should be available, asargued, for different reasons, in (Delacroix &Lawrence 2018). The structures described inthis paper are intended to be extremelyflexible, in order to foster the trust ofdifferent communities, not just the datasubject, unlike most previous research(Edwards 2004, Delacroix & Lawrence 2018).The structure of the paper is as follows. Thenext section looks at the notion of trust, howtrust in the use of data is currently promoted,and how it could be. The following sectionconsiders some of the ethical issues, on theunderstanding that the regulatorybackground, which in the UK and EU is basedaround the General Data ProtectionRegulation, is not sufficient for maintainingtrust. Next, I speculate about what kind ofarchitecture might implement a data trust.The penultimate section examines in somedetail the parallels and divergences between atrust in law and a data trust on Hall andPesenti’s and the ODI’s pragmatic, practicalview, and argues that a data trust can takeinspiration for its structure from the legalconcept of a trust, but it should and could notactually be a legal trust. Finally, a concludingsection will revisit the topic of trust.One final preparatory caveat: I have alreadyused the term ‘data controller’, which is aterm of art from data protection law referringto the person who determines the purposesfor which and the manner in which personal7
Data TrustsKieron O’Haraas big data came along, allowing decisions tobe made about us and profiles attached to uswithout any input from personal data, whichis anonymised or aggregated out of scope.The focus on personal data is already tooweak to protect us from all the inappropriateinterventions that data processing can afford.Trust and trustworthinessData processing is highly regulated. There aredifferent jurisdictions across the globe, butthe EU’s GDPR has set high standards, andcombined them with powerful punishments(fines of tens of millions of euros arepossible), with the aims of making datacontrollers more accountable, and of helpingdata subjects to ensure that their preferencesare respected, and that personal data heldabout them is accurate, proportionate andnot excessive. The GDPR regime has beencriticised for being too powerful, although itsets a useful international benchmark. The USregime is patchier, covering some sectorsmore than others, resulting in a focus onsensitivity and the potential for harm; healthdata, financial data, and data about childrenare regulated more than less problematicdata.Second, many of the trust problems thatconcern Hall and Pesenti (2017), and also theODI researchers, go beyond the problems ofthe data subject, covering the doubts of dataproviders, data consumers and otherstakeholders. Data protection does little forthe concerns of these stakeholders.There are also deeper reasons why even anoverhauled data protection regime is not wellplaced to support trust, which I will considerin the next subsection.Rights and neoliberalismThe data protection regime combines twocomplementary ideological positions. In thefirst place, data protection is part of a rightsbased approach. The individual is perceived tobe in possession of certain rights, which shecan use to defend herself against harm. TheEuropean Convention on Human Rights of1953, developed in the aftermath of thehorrors of Nazi Germany, included an articleenshrining her rights to a private life. Dataprotection regimes add more detailed rightsto this basic idea; the GDPR grants a right ofaccess to data subjects to see their ownpersonal data, as well as some rights to erasepersonal data held by others, rights toexplanations of decisions made about themon the basis of algorithmic processes, and soon. In many cases, data processing can beconsented to via a contract between subjectand processor. The Charter of FundamentalRights of the European Union of 2000 includesrights both to privacy and data protection.Yet there is still something of a trust deficitaround data processing, despite theseregulatory regimes. While this may besurprising at first blush (and indeed at thetime of writing, GDPR is relatively new and socould reassure more people once the lines ofits practical operation become clearer), somereflection on the data protection regime willmake it clearer why it is not well set up tosupport trust in this area.To begin with, trust is a relative term – Xtrusts Y to do something in a particularcontext (O’Hara 2012). The data protectionregime is set up to support one particular typeof X and one particular type of action; the X inquestion is a data subject, and the action isthe processing of personal data from which Xis identifiable. This already limits the regime intwo important ways. First, regulation is often,and inevitably, behind the curve ofinnovation. The Data Protection Directive of1995 was painstakingly developed for astandalone database world, just as the WorldWide Web came along to make linking dataeasier. Similarly, the GDPR of 2018 protects usagainst many of the excesses of the Web, justYet the original Data Protection Directive wasconceived in the context of the EuropeanSingle Market, and so has a dual aspect – itgave data subjects some rights to protecttheir privacy, and gave data controllers rights8
Data TrustsKieron O’Harato gain value from the data. Following it, theGDPR also protects some data sharingpractices, and aim to provide a framework fordata controllers to process personal dataaccountably in a stable and predictableenvironment. From this angle, the datasubject is seen as the defender of her owninterests in a complex marketplace. Thisneoliberal view of the data protection regimesits alongside other mechanisms where theonus is on the individual to understand andexpress her own preferences, and to ensurethey are met, where possible, through herown efforts. Such mechanisms includeconsent regimes, which envisage data subjectand data controller entering into a contractwhen the consent button is pressed, andpersonal data stores, where the data subjectundertakes some administration of her ownpersonal data. Tim Berners-Lee’s recentpromotion of ‘personal online data stores’(pods) falls into this category.for risk, and trusts her not to benefit herselfover and above the fees he pays her. Trust isnot legalistic; a technical breach of the ruleswill be overlooked in a trusting relationship,as long as the intentions behind the breachwere benign and the consequences not tooterrible. Indeed, in many technical areas, theindividual may not even know what her owninterests are, and will trust professionals notonly to defend her interests, but also todefine them. Data protection, on the otherhand, is a legalistic regime, giving the datasubject too narrow a focus to generate trustin the way her data is handled overall.Secondly, both the rights-based approach andneoliberalism place too much onus on theindividual. The individual is to defend herrights. This is, as is frequently argued, quite aburden. Most have better things to do, andfew have the expertise to do it well (Delacroix& Lawrence 2018). Even if the individualengages, she will find herself with quite aburden as she tries to deal with giantcorporations under conditions of asymmetricknowledge. For example, when the datasubject signs a consent form or clicks a privacypolicy, she rarely understands what thisactually means, and so the contract betweenthe two parties is one-sided to say the least.These twin approaches of rights andneoliberalism each have several merits whichI will not review here. However, neither ofthem is very conducive to the development oftrust. There are two reasons for this, onemajor and one minor. The minor reason isthat they focus on particular projects forprocessing data, and rely on the individualpushing back where she believes that she maybe harmed, or at least may not benefit from,such projects. This is small scale; theindividual is supposedly trying to ensure thatvarious detailed rules are followed. Yet trust isa big picture view of the world, not a detailedvision of how people should behave. A trustorexpects a trustee to look out for her interestsin various, possibly unspecified, ways. Thepatient (at least, one without medicaltraining) does not trust the doctor to carry outspecific, detailed procedures; she trusts thedoctor to make her well. The saver does nottrust his accountant to put so much of hismoney here and so much of his money there,but rather trusts her to maximise his incomeor security according to his general appetiteBut most importantly, both the rights-basedapproach and neoliberalism are products of alack of trust, assume trust is in short supply,and make trust difficult to build. Therelationship between the individual and theother is deliberately set up antagonistically. Inthe rights arena, the individual is warned thatthe world is full of potential threats to herwell-being, and by bad actors who will nottreat her with the dignity proper to a humanbeing, and that she therefore needsconventions and courts to protect her. Underneoliberalism, which aims to expand freedomby shrinking public space and growing thepowers of private actors under marketconditions, the individual is told that she mustpursue her own interests, because no-oneelse will do it for her. Under neither of these9
Data TrustsKieron O’Haraconditions is the individual (or the other, forthat matter) incentivised to seek out thecompromise or to initiate the dialogue thatwill enable them to bootstrap trust where it isnot pre-existing.profession in the UK presided over thedisastrous roll-out of the care.data scheme touse primary care data for medical researchand other purposes (2015).Key to the negotiation of a social licence iscommunication. As (O’Hara 2012) argues,trust involves aligning the trustors’ and thetrustees’ understanding of what the trustee iscommitted to, which involves communicatingclearly and precisely what the trustees’intentions are. If the trustors fail tounderstand precisely what the trustees intendto do, then their trust may be based on falseassumptions, and their trust could bemisplaced, despite the trustees’ behaving in aperfectly trustworthy manner by their ownlights. Communication requires engagementand response, and trust will be moreforthcoming if the would-be trustees have agood track record for responsive practice inthe past (Gallois et al 2017). Furthermore,communication needs to be a genuinedialogue, not merely the broadcasting of whatfrom the scientific point of view are truismsexpressed in jargon; engagement is requiredto seek a vocabulary that is meaningful toboth sides of the conversation. Furthermorethe trustors’ attitudes towards evidence andtheir risk assessments also need to beunderstood and accommodated (O’Hara2012). Gallois and colleagues argue thatcommunication accommodation theory is agood frame for the necessary engagement(Giles 2016, Gallois et al 2017).Social licenceEnsuring that data processing is trusted needsa different approach. The operation of atechnology or technocratic policy requiressome kind of big picture approach to act asthe locus of trust. One way of viewing this isto see data science as analogous to otherkinds of technological intervention that needto be accepted by a community and otherrelevant stakeholders before they can operatesuccessfully or profitably. Doctors need to betrusted by their patients (Carter et al 2015),and those drilling or mining for naturalresources need to be trusted by stakeholders,particularly the local community (Gallois et al2017), if coercion is not to be used. Thesetechnological interventions are often justifiedusing the resources of a profession, such asprofessional codes of conduct. The professionand its resources provide the big picturecrucial for trust. At the moment, data scienceis only beginning to develop its professionalstanding. There are plenty of rules – GDPRprovides plenty – but they haven’t solved thetrust problem, and more rules will not help.The sociologist Everett Hughes provided thevaluable notions of licence and mandate(1958). Licence is ‘granted’ informally bysociety for some occupational groups to carryout activities that are part of the job, andmembers of those groups claim a ‘mandate’to define what proper conduct looks like. Thisproduces what Hughes called a “moraldivision of labour”, where society andprofession collaborate in “the setting of theboundaries of realms of social behaviour andthe allocation and responsibility of powerover them”. This is a negotiation. The delicateand informal nature of the licence provides noguarantee that trust will be preserved if theprofessional goes too far – Carter et aldescribe how the highly trusted medicalData trusts as explorations oftrustworthinessA data trust, then, could serve the datascience profession as a focus for a sociallicence, and a locus in which the socialmandate could be negotiated. The data trustwould specify a set of boundaries andresponsibilities for data controllers, and givethe controllers a space in which they couldnegotiate the social mandate for theirprofession. The data trust would then have aclear set of aims.10
Data TrustsKieron O’HaraFirstly, unlike the rights approach or theneoliberal approach inherent in dataprotection, its starting point would be thecompromise between trustor and trustee thatis essential for creating trust in the first place.This involves genuine mutual communicationand consultation. Trust may be hard to build –trust of data processing is all of a piece withtrust of companies (or government), of globalcapitalism (or state power), of security andinfrastructure, and so on.everyone round. Trust of expert systems is acomplex matter. The data scientist needs toearn the mandate to impose and defend thestandards of the profession.Thirdly, the data trust would be a centre fordata processing that could be used to holddata scientists accountable, auditing how theytreat the data and who is allowed access.Fourthly, and relatedly, the data trust wouldaid transparency by being inspectable andscrutable. This would allow individual datasubjects to complain and intervene, as withthe data protection approach. More to thepoint, however, this would also allowrepresentative groups (e.g. patients’ groups,or taxpayers’ representatives) to monitor datause. But the real advantage of a data trust isthat it would allow data scientists to betransparent and accountable to their peers.Data scientists all suffer by untrustworthybehaviour in the profession. For example,Facebook claims innocence in the case ofCambridge Analytica, but even if this isjustified, it has suffered reputational damagebecause of its association. So have some ofthe political campaigns which employedCambridge Analytica. A data trust,importantly, would provide an arena in whichdata scientists could clean up their own act.Secondly, again unlike the other twoapproaches, the expertise of the data scientistis a central part of the picture. For example,sending the data subject a notification ofwhere his data has been sent, and which thirdparties now have it in their control, whetheranonymised or fully personal data, is wellmeant transparency, but hardly useful to thedata subject (O’Neill 2009), who not only hasbetter things to do but who also may struggleto understand a highly complex documentcontaining several names of companies ofwhich he has probably not heard, performingactions, such as auctioning adverts, whosesignificance is unclear to him, and which maynot do him any tangible harm. In the rightsbased and neoliberal approaches, the datasubject is on his own. With a data trust, datascientists can (and should) engage with datasubjects and other stakeholders to determinewhat kind of treatment of data is acceptable,and the scientists themselves may well, if theypresent themselves sympathetically, be ableto inject a good deal of their expertise intothis discussion. They might then be able, ifthey can take their stakeholders with them inthe conversation, to determine to a largeextent which data processing is probably OK,and which not. Individual data subjects maynot care, or be interested in engaging, but in abig data repository, enough subjects, orrepresentative groups, may be able to feed inopinions. The data scientists should absolutelynot assume, ab initio, that they have amonopoly of rationality, and that merelystating their case should be enough to winFinally, a data trust might even help withdetermining which processing is legal. GDPRprovides for a number of grounds for dataprocessing, of which one of the mostimportant is consent. If a data trust were wellenough known and trusted, then it mightbecome the focus of consent. Data subjectswould be asked at collection time whetherthey consented to the use of their data withina (specified?) data trust, for purposesconsistent with the principles underlying thetrust. This has the advantage of being clearand flexible, resisting the GDPR’s tendency toclose down big data opportunities, withoutsuccumbing to a hopeless determinism aboutthe rise of big data. The data trust itself couldalso be a convenient point of contact for a11
Data TrustsKieron O’Haradata subject who wished to withdraw consentat a later date.supply of data under fewer formalconditions). Hence the voluntary constraintsimposed by a data trust may liberate theprocessor to achieve more.The data trust would have to obey the law,naturally. However, this would not be itsraison d’être. As we have seen, merely beinglegal is not sufficient to support trust. Itfollows from this that the data trust should bea voluntary arrangement, rather thanmandated by law. If the latter, the trust couldeasily descend into a box-ticking exercise, asdata protection often does. The point of thedata trust is to signal and to demonstrate thetrustworthiness of the data processing.Voluntary participation is an important part ofthe signal.I have so far written mainly of trust. In fact,the key issue is the trustworthiness of theprocessing. Trust and trustworthiness are twosides of the same coin: trustworthiness is thevirtue of reliably meeting one’s commitments,while trust is the belief of another that thetrustee is trustworthy (O’Hara 2012). Trustwithout trustworthiness is a severevulnerability. Hence what is needed is ameans for (a) establishing the parameters oftrustworthy data science, and(b) demonstrating to would-be trustors thatthe data science is indeed trustworthy, so thatthey could be confident that their trust iswarranted.Put another way, legislation and regulationconstrain data processing, but not sufficientlyto promote widespread trust. If it wouldpromote trust beyond that promoted bycentralise
structures can data trusts exploit, and (b) what relationship do data trusts have to trusts as they are understood in law? The paper defends the following thesis: A data trust works within the law to provide ethical, architectural and governance support for trustworthy data processing Data trusts are therefore both constraining and liberating.