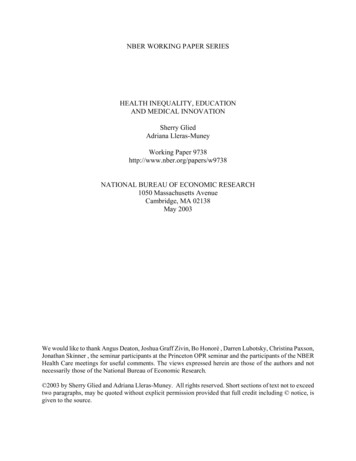
Transcription
NBER WORKING PAPER SERIESHEALTH INEQUALITY, EDUCATIONAND MEDICAL INNOVATIONSherry GliedAdriana Lleras-MuneyWorking Paper 9738http://www.nber.org/papers/w9738NATIONAL BUREAU OF ECONOMIC RESEARCH1050 Massachusetts AvenueCambridge, MA 02138May 2003We would like to thank Angus Deaton, Joshua Graff Zivin, Bo Honoré , Darren Lubotsky, Christina Paxson,Jonathan Skinner , the seminar participants at the Princeton OPR seminar and the participants of the NBERHealth Care meetings for useful comments. The views expressed herein are those of the authors and notnecessarily those of the National Bureau of Economic Research. 2003 by Sherry Glied and Adriana Lleras-Muney. All rights reserved. Short sections of text not to exceedtwo paragraphs, may be quoted without explicit permission provided that full credit including notice, isgiven to the source.
Health Inequality, Education and Medical InnovationSherry Glied and Adriana Lleras-MuneyNBER Working Paper No. 9738May 2003JEL No. I12, I20ABSTRACTRecent studies suggest that health inequalities across socio-economic groups in the US are large andhave been growing. We hypothesize that, as in other, non-health contexts, this pattern occursbecause more educated people are better able than to take advantage of technological advances inmedicine than are the less educated. We test this hypothesis by relating education gradients inmortality with measures medical innovation. We focus on overall mortality and cancer mortality,examining both the incidence of cancer and survival conditional on disease incidence. We findevidence supporting the hypothesis that education gradients are steeper for diseases with moreinnovation.Sherry GliedDepartment of Health Policy and ManagementMailman School of Public Health600 West 168th Street, Room 610New York, NY 10032and NBERsag1@columbia.eduAdriana Lleras-MuneyDepartment of Economics and Woodrow Wilson SchoolPrinceton University320 Wallace HallPrinceton, NJ 08544and NBERalleras@princeton.edu
Socioeconomic disparities in health have increased over the past century in theU.S., the U.K., and continental Europe (Feldman et al., 1989, Pappas et al., 1993,Prston and Elo, 1994, Black Report, 1980, Kunst et al., 2001, Shkolnikov et al.,1998). In the U.S., between 1960 and 1986, the age-adjusted mortality rate for whitemen who had attended college declined from 5.7 to 2.8 per 1000, while the ratedeclined only from 9 to 7.6 for those who had not graduated high school (Pappas etal., 1993).Moreover, the principal causes of death and disability generatingsocioeconomic differentials today are quite different from those a century ago(McKeown, 1976; Cutler and Meara, 2002). Over this period, housing, nutrition, andsanitation have improved; the infectious diseases that were the prime causes of deathbefore World War I account for little mortality today; and access to effective medicalcare for those diseases that remain has become more widespread. Today, gradients arelargest for chronic diseases.Many factors can generate socioeconomic differences in health status at a pointin time.What is more difficult to explain is that these differentials have increasedand have shifted among diseases during the past century (Carroll, Davey, Smith, searchthathassy stematically investigated the question of why gradients move among diseases andwiden over time.In this paper we propose and test one explanation for this pattern: the gradientmoves among diseases because more educated people are able to exploit technologicaladvances in medicine more rapidly. For this reason, the gradient increases where andwhen technological change occurs. The most educated make the best initial use of newinformation about different aspects of health.Over time, this information diffusesalong the education gradient. Thus, all else equal, if technological change ceased, wewould expect the gradient to flatten as well.Our hypothesis is an extension to health of Nelson and Phelps’ (1966) theorythat “the return to education is greater the faster the theoretical level of technologyhas been advancing (p. 72).”A substantial literature examines this pattern in thelabor market (see, for example, Bartel and Sicherman, 1999; Allen, 2001) and in theagricultural sector (Wozniak, 1984). Our hy pothesis is also closely related to thesociological conjecture that socioeconomic status is a “fundamental social cause” ofgradients in health (Link and Phelan 1995, Link, et al., 1998). A fundamental cause is1
one that involves access to resources that can be used to avoid or minimize risks,influences multiple risk factors, and affects multiple disease outcomes. In this view,higher socioeconomic status enables people to better exploit new information andresources.A recurring difficulty in the literature associating gradients in wages totechnological progress is the difficulty of measuring progress (Griliches, 1994). Thisproblem also arises in our context and we follow the labor literature in addressing itby considering several different measures of technological progress. We examine theeffects of these measures in two different datasets that have distinctive strengths andweaknesses for this analy sis. Although our data are limited in several dimensions, wefind evidence that is generally (though not uniformly) supportive of our hypothesis:gradients appear to be largest for diseases where there has been more progress.This paper is organized as follows. We first present a simple formalization ofour proposed explanation of the relationship between education, health, and the rate ofinnovation (Section II).We then present a case study of the HIV epidemic toillustrate how such gradients may evolve.In Section III, we describe our measures of progress and our empiricalapproach. In section IV we look at gradients in 5-year mortality across all diseasesusing data from the National Health Interview Survey (NHIS).We then relate ourestimated education gradients to disease categories with divergent rates of progress.In Section IV, we focus on gradients in cancer incidence and 5-y ear survival(conditional on stage of diagnosis) using data from the Surveillance, Epidemiologyand End Results (SEER) database. Among diseases, cancer is second only tocardiovascular diseases as a contributor to educational gradients in mortality. Cancerprovides a good area to study because improvements (albeit small) have occurred inthe incidence, diagnosis, and treatment of several cancers, and these improvementsvary substantially among cancer subtypes. 1 Additionally, the SEER data links cancerdiagnosis to cancer outcomes in a consistent way across subtypes, whereas for otherdiseases, surveillance data on risk factors cannot be as readily linked to outcomes. 21 For example, there have been substantial improvements in survival after diagnosis of early stage colon cancer(DHHS, 2001). Progress has been made in diagnosing breast and prostate cancer.2 For example, people with high cholesterol or high blood pressure are at risk of mortality from multiplediseases, making it difficult to link mortality outcomes for each of these diseases with progress.2
In Section V, we examine how changes in progress affect changes in theeducation gradient.results.In Section VI, we evaluate different causal explanations of ourIn Section VII, we relate gradients to technological progress prospectively.Section VIII concludes.II. – Relating Outcomes to ProgressMany recent studies of health status differentials focus on gradients associatedwith education. These studies generally find that education is closely correlated withhealth status (even controlling for income), and suggest that the relationship iscausal. 3 Several explanations have been proposed. Grossman (1972) suggested thateducation leads to better health by improving the technology for health production.This might include having access to more information about health risks, makingbetter use of that information or more effectively searching for high quality healthproviders (Rosenzweig, 1995).Education may also have indirect effects on health by increasing income andimproving access to the resources needed to improve health. The extent to whicheducation affects health may also depend on the available health care technology,because better-educated people might be better able to use certain health caretechnologies (Goldman and Lackdawalla, 2002).Rather than understanding thespecific mechanism than generate the education gradient forparticular disease at agiven point in time, this paper’s objective is to understand what makes educationgradients increase over time or shift from one disease to another. To do this, we focuson the role of education in the diffusion of technological progress.A formalization of the relationship between health, education and the rate ofinnovationThe following formalization closely follows Nelson and Phelps (1966). Thismodel is only illustrative. It captures the basic features of our hypothesis in a simplefashion and provides guidelines for our empirical approach.Suppose that the health H of an individual can be modeled as a function of thelevel of technology A that the individual has access to, and other inputs C:3 For example see Lleras-Muney (2002) shows that increases in education induced by compulsory schoolinglaws lead causally to improvements in health status.3
H H ( A, C )The frontier level of technology is given by T(t), whereT (t ) T0eλtT(t) is the level of technology if technology is instantaneously diffused and λ is theexogenous rate of technological progress. Suppose now that the level of dlyindividualsadoptnewtechnologies, and that the lag between innovation and adoption is a decreasingfunction of education, so thatA(t ) T (t w(e)) T0eλ ( t w( e ))where w’(e) 0. This key assumption captures the ideas that were presented in theintroduction -- that is that the more educated “adopt” new technologies at a faster ratebecause of better access to information, better use of information, and better capacityto search for better providers and or treatments. This feature can be generated frommaximization principles simply by assuming differential costs of technology adoption.Note that this model assumes that individuals have chosen education in previousperiods and that technological changes are unanticipated. In this context, we canexpress the health of the individual as:H H (T0eλ ( t w( e )) , C )The derivative of the health production function with respect to education gives u swhat is known as “the education gradient” in health. It gives the marginal gain inhealth induced by an additional unit of schooling. In this model it can be expressedas: H H λw' (e) A 0 e A H H w' (e) A 0 e λ ASince w’(e) is negative, the model predicts that health is an increasing functionof education and that the rate of return of education is larger the higher the rate oftechnological change. We can test this prediction in the data, first by estimating thedisease-specific education gradient, and then by relating the size of the gradient to4
measures of innovations that proxy for the parameter λ. Note that we think oftechnology here in very broad terms: it includes all innovations that affect the mannerand the rate in which we can transform inputs into health. So in this view, newknowledge is considered innovation.This simple model predicts that in the absence of technological change (λ 0),there should be no difference between the educated and the uneducated. We do notbelieve that technology adoption is the only reason why education and health might berelated. For example, even in the absence of progress we can expect educationgradients if the more educated are better at utilizing complex technologies (e.g.Rosenzweig, 1995 Goldman and Smith, 2001). A more elaborate model couldincorporate these other mechanisms. As the literature review in the introductionshowed, there is a substantial amount of work that has investigated these. We willtherefore focus only on whether higher rates of technological progress are associatedwith larger gradients in our empirical work. 4A Case StudyHIV disease provides an interesting case study of the relationship betweeneducation gradients and medical progress because the disease is new.At thebeginning of the HIV epidemic, in the early 1980s, before information abouttransmission or treatment was available, cases were concentrated among gay men, agroup with substantially higher than average educational attainment: 67% have at leasta college degree (Bozzette, 1998).Since the early 1980s, however, there have been markedly different trends inthe HIV/AIDS epidemic for groups with different levels of education.Behavioralchanges among gay men began immediately after the method of transmission of thehuman immunodeficiency virus was identified in 1982.In the largest study oftransmissions in that period, new infections in this group fell from 20.8% of asusceptible cohort in 1982 to 2.1% of that cohort in 1983 (Centers for DiseaseControl, 1987).By the late 1980s, new cases among gay men were well below4 Note that there is no sense in which there exist general equilibrium effects in the health model: if everyoneobtains more schooling everyone’s health improves. While the income returns to education are determined bythe labor market, the returns to education in health are only determined by the individual health productionfunction. In this respect our theory differs from the “fundamental causes” theory in that it is not a zero sumgame.5
projected rates, while rates among IV drug users were at or above projected levels,suggesting that there had been little behavioral change in this group (Bloom andGlied, 1992). As early as 1983, new AIDS cases began to be concentrated in lowincome areas (Fordy ce, 1998).The effects of new information on the gradient in HIV incidence were latermagnified by the effects of new treatment technologies on HIV mortality.We usedata from the Centers for Disease Control WONDER system to map annual death ratesamong the population living with AIDS by exposure category (Figure 1). There weretwo major treatment advances in HIV care over this period. After a short period ofclinical trials, the FDA approved the first effective AIDS drug, AZT, in 1987 (Brown,1987). A second, more effective, group of drugs, the highly active antiretroviraltherapies (HAART) based on protease inhibitors, was introduced beginning in the late1990s.The FDA approved the first HAART-related protease inhibitor, invirase, inDecember 1995 and the development of combination therapies that made use of thesedrugs followed over the subsequent 18 months (McGinley and Womack, 1995;Goldman and Lakdawalla, 2001). As Figure 1 shows, the introduction of AZT and ofHAART drugs was associated with a divergence in the death rate between gay men andother transmission groups. 5 This pattern suggests that the more educated group wasquicker in taking advantage of the new treatment technology.Case studies of specific populations support this finding (see Appendix ereweresignificantsocioeconomic differences (measured by race and exposure group) in receipt of AZTin a cohort studied in 1987-1988, but that these differences had largely disappeared by1989-1990.Two studies examine changing socioeconomic status disparities inHAART between 1996 and 1998 (Cunningham et al, 2001; Sambamoorthi et al, 2001).Cunningham et al. find that the gap between the percentage of college educated HIVinfected people who had ever used HAART compared to the percentage among thosewith less than a high school degree shrank form 27 points in 1996 (49:22) to 14 pointsin 1998 (79:65). Sambamoorthi et al, 2001 find a similar pattern in receipt of HAARTover time by race and exposure group.5 Note that these death rates are conditional on incidence of AIDS. Our data do not capture the extent to whichpeople with HIV began taking these drugs before developing AIDS symptoms and never progressed to AIDS.6
Today, lower educational attainment is highly correlated with mortality amongAIDS patients (Schechter, 1994).More highly educated patients are more likely toadhere to therapy (Goldman and Smith, 2002), have greater access to antiretroviraltherapy and protease inhibitors (Sorvillo, 1999), are more likely to participate inclinical trials (Seltzer, 1989), and have far more knowledge about AIDS (Sorenson,1999).The HIV case study provides an interesting example of how education mayinteract with new treatment technologies.Several other case studies similarlydocument more rapid diffusion of new health innovations among more highly educatedpeople relative to less educated people (see, for example, Link et al. (1998)). In thenext sections, we attempt to find more sy stematic evidence for this pattern.III. –Progress measures and empirical approachMeasures of progressThere is no consensus about how to measure either progress or the relativeimportance of progress (Allen, 2001; Bartel and Sicherman, 1999).Instead, wecompute multiple measures of innovation for each of the 55 diseases in the NHISMCD and the 81 cancer sites in the SEER data. Table 1 describes these measures. Notall progress measures are available in both datasets.Measurement of progress by disease is particularly problematic becauseprogress in the prevention or treatment of one disease can leave a larger straightforwardmeasuresoftechnological progress are those that describe innovation in survival conditional ondiagnosis of a disease. 61. Number of drugsThe SEER data contain information on survival conditional on diagnosis. Wecan link these data to information on the number of drugs approved by the FDA totreat a particular cancer (SEER), a direct measure of the rate of pharmaceuticalinnovation for each particular cancer site. 7We cannot assign drugs to disease6 Note that progress in the treatment or prevention of other diseases may still affect outcomes conditional ondiagnosis if the newly susceptible population is different (for example, more or less fragile) from the originalpopulation.7 As in Lleras-Muney and Lichtenberg (2002) or Lichtenberg (2002), we use the number of new activeingredients approved by the FDA rather than the number of new drugs, which we consider a better measure ofinnovation in drug treatments (the FDA also approves generic equivalents and new dosages of the same drug7
categories in the NHIS because the match between drugs and causes of death is highlyimperfect, since drugs are used for conditions that can lead to death from multiplecauses. For example, drugs to control diabetes can reduce death rates from diabetes,heart disease, stroke, kidney failure, and other conditions.2. Change in 5-year survival ratesUsing the SEER data, we compute the change in the 5-year survival rateconditional on diagnosis. Like the drug measure, this measure is related to innovationthat affects survival conditional on diagnosis. Unlike drugs, which only capture aspecific type of innovation, this measure is more comprehensive: progress in surgicalprocedures, radiation and other aspects will be reflected in the survival rate. Howevernote that this measure is also affected by innovations in diagnostic technology thatlead to cancers being diagnosed earlier. 8Over this period, survival conditional ondiagnosis has increased.3. Change in age-adjusted mortalityA broader measure of progress, which can be confounded by changes in diseaseincidence, is the change in the age-adjusted mortality rate. The National CancerInstitute provides a measure of the trend in age-adjusted mortality from each type ofcancer: the estimated annual percent change (EAPC), which is the coefficient from alog-linear regression of mortality rates on calendar year. The EAPC is positive if ageadjusted mortality increased and negative if age-adjusted mortality decreased;therefore a negative value for EAPC constitutes progress. Across all 81-cancer sitesthere has been progress in cancer mortality over this period.For the NHIS data we calculate the same measure (EAPC) of age-adjustedmortality using data from the compressed mortality files provided by the CDC. Thecompressed mortality files provide us with age-adjusted mortality rates for whites foreach year from 1986 to 1995 that we use to calculate the EAPC using the same metho dthat the National Cancer Institute uses.4. Changes in age-adjusted incidencefor example). For each cancer site we compute the number of drugs that exist in the market as of 1999. Thesedata were constructed using several sources: First Data Bank provided a list of the drugs that are used to treatcancers, and the date of FDA approval of the active ingredient in each drug was kindly provided by FrankLichtenberg.8 We examine this directly by evaluating changes in the gradient in stage of diagnosis, discussed in footnote xxbelow, and by controlling for stage of diagnosis in the regression analyses.8
A final measure of technological change is innovation in disease prevention.New knowledge associated with disease prev ention should allow people to avoidgetting a disease in the first place. Unfortunately, we do not have any direct measuresof information about disease risk factors.The efficiency with which epidemiologists are able to identify risk factors fordisease increases as the proportion of all susceptible people who develop the diseaseapproaches 0.5 (Rothman and Greenland, 1998).In our context, the prevalence ofevery disease or disease subtype in the population is well below 0.5.This suggeststhat the amount of available information about risk factors for a disease or diseasesubtype will be increasing in the incidence of that disease. 9We conjecture that asdisease incidence increases, information (and gradients) also increase.This patternshould hold even if increases in disease incidence are simply a consequence ofimprovements in the prevention and treatment of other diseases.We examine thisinterpretation of changes in incidence in Section VII below.The National Cancer Institute provides a measure of the trend in age-adjustedincidence: the estimated annual percentage change in age-adjusted incidence (EAPCI).In light of our argument above, positive EAPCI values constitute proxies for progressin the identification of disease risk factors. Overall, incidence rates for most cancersubtypes have increased.Note that there are multiple reasons why incidence couldincrease including advances in diagnostic technology, increases in environmental riskor in progress in the prevention and treatment of other diseases (who leave morepeople alive and susceptible to cancer). Our conjecture that information about riskfactors increases with increasing prevalence should hold regardless of the causes forincreasing incidence. 10 Innovation measures by disease are listed in appendices A andB.While these measures are related to one another, the correlation among them isnot very high, suggesting that they all describe distinct components of progress (Table2). Changes in incidence and mortality are positively and highly correlated. Increasesin survival and in mortality are negatively correlated, but survival and incidence arenot highly correlated. Both incidence and mortality are positively correlated with new9 In a preliminary search of Medline, we found that publications on the etiology of diseases are increasing indisease prevalence.10Note that as information about risk factors diffuses incidences will fall, and so we would expect incidenceto diminish9
drugs, which may indicate that pharmaceutical manufacturers target diseases withrising incidence (as we discuss below). Drugs are positively correlated with changesin survival.Empirical implementation Across Diseases and Disease SubtypesOur hypothesis suggests that gradients in education should be greatestwhere medical progress has been greatest.Our empirical strategy to evaluate thishypothesis consists in estimating education gradients (by disease or disease subtype)and relating these gradients to measures of medical progress.Although there existdifferent measures of the gradient, in this study we alway s define gradients as thedifference (rather than the ratio) in health outcomes between educated and uneducatedindividuals. We consider three empirical specifications.In the most flexible, specification, which we employ in both the NHIS andSEER data, we estimate separate regressions for each disease (NHIS) or cancer site(SEER), including a full set of controls,(1) P (died 1)ij β 0 β1 j educationij X ijγ eij , j 1.n .where i indexes individuals, and j indexes the n different diseases. We use thecoefficient on education from each regression as the dependent variable in the secondstage regression that contains as many observations as disease gradients (n),(2) β j δ 0 δ1 progress j ε j , j 1.nAccording to our hypothesis, we expect δ 1 to be negative: larger progress translatesinto more negative gradients. In this fully flexible form, none of the variables areconstrained to be the same across sites.Unfortunately, because of the very large number of dummy variables in ourSEER specification, there is little statistical power available to identify results usingthis specification in these data so for these data, we begin with two less flexiblespecifications. 11 In the least flexible specification, we estimate a linear probabilitymodel of the probability of dying within 5 y ears after diagnosis (conditional on stageat diagnosis), where education is interacted with progress:11 I n p r i n c i p l e , t h e d u m m y v a r i a b l e s a l o n e f u l l y i d e n t i f y 7 3 , 7 8 2 o b s e r v a t i o n s , a n d f o r m a n y c a n c e r s i t e s w ehave far fewer observations available (see Appendix 1).10
(3) P (died 1) β 0 β1education β 2education * progress Xγ e .Our model suggests β 2 should be negative. Note that in this specification we constrainthe coefficients on all variables to be the same across all cancer sites, except that weallow education to vary with our measure of medical progress.In a second, preferred specification, we free up the functional form byseparating the two stages. Initially, we run a single individual level regression,including a full set of controls and interacting education with cancer site dummies andstage of diagnosis dummies:(4) P (died ij 1) β 0 8181j 1j 1 β j educationi * cancerj θ j stage j * cancerj X ijγ eijwhere i indexes individuals, and j indexes the 81 different cancers. As with the fullyflexible form, the resulting 81 coefficients (labeled β j ) from the interaction terms(education*cancer site) become the dependent variable in a second stage regressionwhere medical progress related to that cancer site is the independent variable:(5) β j δ 0 δ1 progress j ε j , j 1.81In this specification, the effects of education and stage are allowed to vary by cancersite, but all other variables are constrained to have the same effect across sites. As inthe fully flexible specification, measures of progress are not included when estimatingeducation gradients, eliminating the possible endogeneity of the progress measureswith respect to the probability of dying.IV- Gradients by Disease – Results from the NHISDataWe first measure education gradients in mortality using the NHIS-MCD files.The NHIS is an annual cross sectional survey of the U.S. population. All respondents18 years of age or older in the 1986-1994 surveys were subsequently matched to ollowingtheepidemiological literature, we focus on five-year mortality and, to avoid censoring,use only observations from the 1986-1990 NHIS interviews. The data contain severalsocio-economic variables including y ears of education and family income, and the11
mortality data contain information on all causes of death. For purposes of comparisonwith the cancer data described below, we restrict the NHIS sample to whites ages ains164,373observations. Summary statistics for the NHIS-MCD data are reported in Table 3.The Effect of Education on MortalityWe first examine the overall effect of education on the probability of surviving5 years.We estimate the model in (1) above where the X includes Hispanic status,gender, marital status, interview y ear and single years-of-age dummies. 12Table 4documents the gradient in education for all-cause mortality. The effect of education isnegative and significant, slightly larger for males than for females but this differenceis not statistically significant. At the mean, these coefficients imply that one moreyear of education reduces 5-y ear mortality by about 5%. Table 5 shows the results bydisease category for broad categories. We find a negative effect of education o nmortality from cancer, respiratory system diseases, cardiovascular diseases, digestivesy stem diseases and other diseases. The effect of education on mortality from diabetesor infectious diseases is negative but not statistically significant.The Effect of Progress on the GradientWe next relate the education gradients by disease with progress in mortality forthat disease. Figure 2 shows the relationship for broad disease categories. The ases),consistentwithourhypothesis, that the gradient is largest for diseases where mortality has decreasedmost.We next examine the relationship using 55 detailed categories of death. 13 Foreach cause of death we estimate the effect of education on the probability of dying inthe next five y ears as in tables 4 and 5. We then match coefficients from theseregressions to changes in disease-specific mortality. Table 6 presents results fromregressions that are weighted b
Mailman School of Public Health 600 West 168th Street, Room 610 New York, NY 10032 and NBER . Princeton, NJ 08544 and NBER alleras@princeton.edu. 1 Socioeconomic disparities in health have increased over the past century in the U.S., the U.K., and continental Europe (Feldman et al., 1989, Pappas et al., 1993, . fashion and provides .