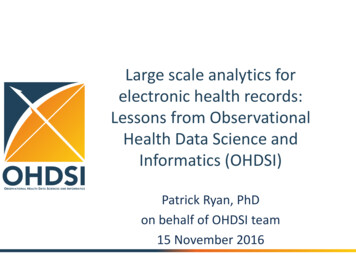
Transcription
Large scale analytics forelectronic health records:Lessons from ObservationalHealth Data Science andInformatics (OHDSI)Patrick Ryan, PhDon behalf of OHDSI team15 November 2016
Odyssey (noun): \oh-d-si\1. A long journey full of adventures2. A series of experiences that giveknowledge or understanding /odyssey
OutcomeTreatmentDiseaseA caricature of the patient journeyConditionsDrugsProceduresMeasurementsPerson timeBaseline time0Follow-up time
Baseline timeBaseline timeBaseline timePerson time0 timePersonFollow-up timeFollow-up time0Person timeFollow-up time0OutcomeOutcomePerson utcomePerson NConditionsDiseasePerson 3DiseasePerson 2DiseaseDiseasePerson 1TreatmentTreatmentTreatmentTreatmentEach observational database is just an(incomplete) compilation of patient journeys
OutcomeTreatmentWhich treatment didpatients choose afterdiagnosis?DiseaseQuestions asked across the patient journeyConditionsWhichDrugspatients chosewhich treatments?Does one treatmentcause the outcome morethan an alternative?ProceduresMeasurementsHow many patientsexperienced the outcomeafter treatment?Person timeBaseline timeWhat is theprobability I willdevelop the disease?Does treatment causeoutcome?Follow-up time0 What is the probability I willexperience the outcome?
Classifying questions across the patientjourney Clinical characterization: What happened to them?– What treatment did they choose after diagnosis?– Which patients chose which treatments?– How many patients experienced the outcome after treatment? Patient-level prediction: What will happen to me?– What is the probability that I will develop the disease?– What is the probability that I will experience the outcome? Population-level effect estimation: What are the causal effects?– Does treatment cause outcome?– Does one treatment cause the outcome more than an alternative?
Complementary evidence to inform thepatient journeyClinicalcharacterization:What happened tothem?observationPatient-levelprediction:What will happento me?Population-leveleffect estimation:What are thecausal effects?inferencecausal inference
Introducing OHDSI The Observational Health Data Sciences andInformatics (OHDSI) program is a multistakeholder, interdisciplinary collaborative tocreate open-source solutions that bring outthe value of observational health data throughlarge-scale analytics OHDSI has established an internationalnetwork of researchers and observationalhealth databases with a central coordinatingcenter housed at Columbia Universityhttp://ohdsi.org
OHDSI’s missionTo improve health, by empowering a communityto collaboratively generate the evidence thatpromotes better health decisions and bettercare.http://ohdsi.org
What is OHDSI’s strategy to deliverreliable evidence? Methodological research– Develop new approaches to observational data analysis– Evaluate the performance of new and existing methods– Establish empirically-based scientific best practices Open-source analytics development– Design tools for data transformation and standardization– Implement statistical methods for large-scale analytics– Build interactive visualization for evidence exploration Clinical evidence generation– Identify clinically-relevant questions that require real-world evidence– Execute research studies by applying scientific best practices throughopen-source tools across the OHDSI international data network– Promote open-science strategies for transparent study design andevidence dissemination
OHDSI communityOHDSI Collaborators: 140 researchers in academia, industry, government, health systems 20 countries Multi-disciplinary expertise: epidemiology, statistics, medicalinformatics, computer science, machine learning, clinical sciencesDatabases converted to OMOP CDM within OHDSI Community: 50 databases 660 million patients
Comparative effectivenessOne common datamodeltosupportHealth economicsmultipleusecases Clinical researchQualityof careDrug safety surveillanceDevice safety surveillanceVaccine safety surveillancePersonObservation periodSpecimenStandardized health system dataLocationProviderDeathVisit occurrenceCostDrug exposureDevice exposureObservationFact relationshipCohort attributeCondition eraDrug eraDose eraStandardized derivedelementsCondition occurrenceNoteConceptVocabularyDomainConcept classConcept relationshipRelationshipConcept synonymCohortMeasurementCDM sourceConcept ancestorSource to concept mapDrug strengthCohort definitionAttribute definitionStandardized vocabulariesProcedure occurrenceStandardized healtheconomicsPayer plan periodStandardized clinical dataCare siteStandardized meta-data
Complementary evidence to inform thepatient journeyClinicalcharacterization:What happened tothem?observationPatient-levelprediction:What will happento me?Population-leveleffect estimation:What are thecausal effects?inferencecausal inference
How should patients with majordepressive disorder be treated?
How are patients with majordepressive disorder ACTUALLY treated?Hripcsak et al, PNAS, 2016
OHDSI participating data partnersCodeAUSOMNameAjou University School of MedicineDescriptionSouth Korea; inpatient hospitalEHRUS private-payer claimsSize (M)2CCAECPRDMarketScan Commercial Claims andEncountersUK Clinical Practice Research DatalinkUK; EHR from general practice11CUMCColumbia University Medical CenterUS; inpatient EHR4GEGE CentricityUS; outpatient EHR33INPCUS; integrated health exchange15JMDCRegenstrief Institute, Indiana Network forPatient CareJapan Medical Data CenterJapan; private-payer claims3MDCDMarketScan Medicaid Multi-StateUS; public-payer claims17MDCRMarketScan Medicare Supplemental andCoordination of BenefitsOptum ClinFormaticsStanford Translational Research IntegratedDatabase EnvironmentHong Kong UniversityUS; private and public-payerclaimsUS; private-payer claimsUS; inpatient EHR9Hong Kong; EHR1OPTUMSTRIDEHKU119402Hripcsak et al, PNAS, 2016
Treatment pathway study design 250,000,000 patient records used across OHDSI network 4 years continuous observation 3 years continuous treatment from first treatmentN 264,841 qualifying patients with depressionHripcsak et al, PNAS, 2016
How are patients with majordepressive disorder ACTUALLY treated? Substantial variation intreatment practice acrossdata sources, health systems,geographies, and over time Consistent heterogeneity intreatment choice as nosource showed one preferredfirst-line treatment 11% of depressed patientsfollowed a treatmentpathway that was sharedwith no one else in any of thedatabasesHripcsak et al, PNAS, 2016
One standardized approach can beapplied to multiple clinical areasType 2 Diabetes sionMDCDGEOPTUMHripcsak et al, PNAS, 2016
Complementary evidence to inform thepatient journeyClinicalcharacterization:What happened tothem?observationPatient-levelprediction:What will happento me?Population-leveleffect estimation:What are thecausal effects?inferencecausal inference
21
Observational research results inliterature85% of exposure-outcome pairs have p 0.05What’s going wrong? Observational study bias Publication bias P-hacking29,982 estimates11,758 papers22
Observational research in depression1,935 estimates23
What if we considered all outcomes?Duloxetine vs. Sertraline for these 22 outcomes:Acute liver injuryHypotensionAcute myocardial onNauseaDecreased libidoOpen-angle de and suicidal ideationGastrointestinal hemorrhageHyperprolactinemiaTinnitusVentricular arrhythmia and sudden cardiacdeathHyponatremiaVertigo24
What if we consider all tazapineElectroconvulsive riptyline25
Large-scale estimation for depression17 treatments17 * 16 272 comparisons22 outcomes272 * 22 5,984 effect size estimates4 databases so far (Truven CCAE, Truven MDCD,Truven MDCR, Optum) 4 * 5,984 23,936 estimates NOT DATA MINING - Each analysis following bestpractice in causal inference26
Estimates are in line with expectations11% of exposure-outcome pairs havecalibrated p 0.05In literature, 85% have p 0.0527
OHDSI’s recommended best practicesfor population-level effect tionDissemination Write and shareprotocol Open source studycode Use validatedsoftware Replicate acrossdatabases Produce standarddiagnostics Include negativecontrols Create positivecontrols Calibrateconfidenceinterval and pvalue Don’t provide onlythe effectestimate Also shareprotocol, studycode, diagnosticsand evaluation Produce evidenceat scale28
Complementary evidence to inform thepatient journeyClinicalcharacterization:What happened tothem?observationPatient-levelprediction:What will happento me?Population-leveleffect estimation:What are thecausal effects?inferencecausal inference
Populations can be used to accuratelypredict outcomes for individualsStrokeAUCCCAEHypothyroidism NauseaMDCDDiarrheaOPTUM MDCRAMI1.000.900.800.700.600.50
Building the LHC of observationalresearch?31
Join the journey Discussion / questions / commentsryan@ohdsi.org
The Observational Health Data Sciences and Informatics (OHDSI) program is a multi-stakeholder, interdisciplinary collaborative to create open-source solutions that bring out the value of observational health data through large-scale analytics OHDSI has established an international network of researchers and observational