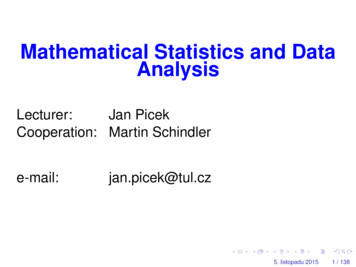
Transcription
Mathematical Statistics and DataAnalysisLecturer:Jan PicekCooperation: Martin Schindlere-mail:jan.picek@tul.cz5. listopadu 20151 / 138
Course outline:1Descriptive statistics: Basic descriptive statistics. Types ofvariables, frequency distribution, graphical data processing.Basic characteristics of location and variability, ordered data.2The calculations of basic characteristics of the ordered data.Boxplot. Multidimensional data - correlation coefficient.3Probability theory: event, the definition of probability,probability properties.4Random variable. Probability distribution. Distributionfunction, density, quantile function. Characteristics ofrandom variables.5Discete distribution: alternative, binomial, geometric,hypergeometric, Poisson.6Normal distribution, Central limit theorem - Moivreova Laplace theorem. Continuous distribution: uniform,exponential, Student and F distributions.5. listopadu 20152 / 138
891011121314Multivariate random variable (vector). Dependence covariance and correlation coefficient.Introduction to Mathematical Statistics. Point estimates,interval estimates for parameters of normal and binomialdistribution.Basic concepts of statistical hypothesis testing. Tests ofhypotheses on the parameters of normal distribution.Non-parametric tests. Tests of hypotheses about theparameters of the binomial distributionGoodness of fit tests and their application.Correlation and regression. Spearman’s coefficient of serialcorrelation.Linear regression, method of least squares.5. listopadu 20153 / 138
StatisticsStatistics is a discipline that deals with the collection,organization, analysis, interpretation and presentation ofdata. Only events appearing at a large set of cases, not onlyat individual cases, are of interest.Data set is a set of statistical units (inhabitants, towns,companies,.), on which we measure values ofvariable(age, number of inhab., turn-over,.)Measurements are recorded in an appropriate scale (levelsof measurement).On one unit we can measure several characteristics - thatallows to study correlation (Is there a relationship betweenheight and weight in the studied population?).5. listopadu 20154 / 138
StatisticsStatistics is a discipline that deals with the collection,organization, analysis, interpretation and presentation ofdata. Only events appearing at a large set of cases, not onlyat individual cases, are of interest.Data set is a set of statistical units (inhabitants, towns,companies,.), on which we measure values ofvariable(age, number of inhab., turn-over,.)Measurements are recorded in an appropriate scale (levelsof measurement).On one unit we can measure several characteristics - thatallows to study correlation (Is there a relationship betweenheight and weight in the studied population?).5. listopadu 20154 / 138
StatisticsStatistics is a discipline that deals with the collection,organization, analysis, interpretation and presentation ofdata. Only events appearing at a large set of cases, not onlyat individual cases, are of interest.Data set is a set of statistical units (inhabitants, towns,companies,.), on which we measure values ofvariable(age, number of inhab., turn-over,.)Measurements are recorded in an appropriate scale (levelsof measurement).On one unit we can measure several characteristics - thatallows to study correlation (Is there a relationship betweenheight and weight in the studied population?).5. listopadu 20154 / 138
StatisticsStatistics is a discipline that deals with the collection,organization, analysis, interpretation and presentation ofdata. Only events appearing at a large set of cases, not onlyat individual cases, are of interest.Data set is a set of statistical units (inhabitants, towns,companies,.), on which we measure values ofvariable(age, number of inhab., turn-over,.)Measurements are recorded in an appropriate scale (levelsof measurement).On one unit we can measure several characteristics - thatallows to study correlation (Is there a relationship betweenheight and weight in the studied population?).5. listopadu 20154 / 138
We can treat the data set two different ways:1Descriptive statistics - we make conclusions only for thestudied data set from the observed data (we measured allthe units in the population we want to describe)2Mathematical (inferential) statistics - studied data set istreated as a sample data – set of units randomly andindependently selected from target population that is large(cannot be explored completely for time, financial ororganizational reasons). We want to make conclusionsabout the whole population only from the sample values(second half of semester).5. listopadu 20155 / 138
We can treat the data set two different ways:1Descriptive statistics - we make conclusions only for thestudied data set from the observed data (we measured allthe units in the population we want to describe)2Mathematical (inferential) statistics - studied data set istreated as a sample data – set of units randomly andindependently selected from target population that is large(cannot be explored completely for time, financial ororganizational reasons). We want to make conclusionsabout the whole population only from the sample values(second half of semester).5. listopadu 20155 / 138
Descriptive statisticsTypes of scaleszero-one (male/female, smoker/nonsmoker)nominal (marital status, eye color) - disjoint categories thatcannot be orderedordinal (education level, satisfaction level) - nominal scalewith ordered categoriesinterval (temperature in Celsia degree, year of birth) values are numeric, distance between the neighboringvalues is constant, an arbitrarily-defined zero pointratio (weight, hight, number of inhabitants) - values aregiven in a multiple of a unit quantity, zero meansnonexistence of the measured characteristic.- Qualitative: zero-one, nominal, ordinal- Quantitative (continuous): interval, ratio5. listopadu 20156 / 138
Descriptive statisticsTypes of scaleszero-one (male/female, smoker/nonsmoker)nominal (marital status, eye color) - disjoint categories thatcannot be orderedordinal (education level, satisfaction level) - nominal scalewith ordered categoriesinterval (temperature in Celsia degree, year of birth) values are numeric, distance between the neighboringvalues is constant, an arbitrarily-defined zero pointratio (weight, hight, number of inhabitants) - values aregiven in a multiple of a unit quantity, zero meansnonexistence of the measured characteristic.- Qualitative: zero-one, nominal, ordinal- Quantitative (continuous): interval, ratio5. listopadu 20156 / 138
Descriptive statisticsTypes of scaleszero-one (male/female, smoker/nonsmoker)nominal (marital status, eye color) - disjoint categories thatcannot be orderedordinal (education level, satisfaction level) - nominal scalewith ordered categoriesinterval (temperature in Celsia degree, year of birth) values are numeric, distance between the neighboringvalues is constant, an arbitrarily-defined zero pointratio (weight, hight, number of inhabitants) - values aregiven in a multiple of a unit quantity, zero meansnonexistence of the measured characteristic.- Qualitative: zero-one, nominal, ordinal- Quantitative (continuous): interval, ratio5. listopadu 20156 / 138
Descriptive statisticsTypes of scaleszero-one (male/female, smoker/nonsmoker)nominal (marital status, eye color) - disjoint categories thatcannot be orderedordinal (education level, satisfaction level) - nominal scalewith ordered categoriesinterval (temperature in Celsia degree, year of birth) values are numeric, distance between the neighboringvalues is constant, an arbitrarily-defined zero pointratio (weight, hight, number of inhabitants) - values aregiven in a multiple of a unit quantity, zero meansnonexistence of the measured characteristic.- Qualitative: zero-one, nominal, ordinal- Quantitative (continuous): interval, ratio5. listopadu 20156 / 138
Descriptive statisticsTypes of scaleszero-one (male/female, smoker/nonsmoker)nominal (marital status, eye color) - disjoint categories thatcannot be orderedordinal (education level, satisfaction level) - nominal scalewith ordered categoriesinterval (temperature in Celsia degree, year of birth) values are numeric, distance between the neighboringvalues is constant, an arbitrarily-defined zero pointratio (weight, hight, number of inhabitants) - values aregiven in a multiple of a unit quantity, zero meansnonexistence of the measured characteristic.- Qualitative: zero-one, nominal, ordinal- Quantitative (continuous): interval, ratio5. listopadu 20156 / 138
Descriptive statisticsTypes of scaleszero-one (male/female, smoker/nonsmoker)nominal (marital status, eye color) - disjoint categories thatcannot be orderedordinal (education level, satisfaction level) - nominal scalewith ordered categoriesinterval (temperature in Celsia degree, year of birth) values are numeric, distance between the neighboringvalues is constant, an arbitrarily-defined zero pointratio (weight, hight, number of inhabitants) - values aregiven in a multiple of a unit quantity, zero meansnonexistence of the measured characteristic.- Qualitative: zero-one, nominal, ordinal- Quantitative (continuous): interval, ratio5. listopadu 20156 / 138
Descriptive statisticsExample - one-dimensional- one-dimesional datawe study IQ scores of 62 pupils from 8-th grade in a certainprimary schoolhow to describe and evaluate what have the data in commonor how much they differ from each other?from the data set (values of the variable) we calculatecharacteristics (characteristics of location, variability, shapeof the distribution, for multi-dimensional dat alsocharacteristics of correlation)a characteristic (a statistic) expresses (evaluate) givenproperty by one number5. listopadu 20157 / 138
Descriptive statisticsExample - one-dimensional- one-dimesional datawe study IQ scores of 62 pupils from 8-th grade in a certainprimary schoolhow to describe and evaluate what have the data in commonor how much they differ from each other?from the data set (values of the variable) we calculatecharacteristics (characteristics of location, variability, shapeof the distribution, for multi-dimensional dat alsocharacteristics of correlation)a characteristic (a statistic) expresses (evaluate) givenproperty by one number5. listopadu 20157 / 138
Descriptive statisticsExample - one-dimensional- one-dimesional datawe study IQ scores of 62 pupils from 8-th grade in a certainprimary schoolhow to describe and evaluate what have the data in commonor how much they differ from each other?from the data set (values of the variable) we calculatecharacteristics (characteristics of location, variability, shapeof the distribution, for multi-dimensional dat alsocharacteristics of correlation)a characteristic (a statistic) expresses (evaluate) givenproperty by one number5. listopadu 20157 / 138
Descriptive statisticsExample - data setmeasured values denote by x1 , x2 . . . , xn , now n 62.1079210713810413496141 105 111 11296 103 14072 123 140 112 127 120 106108 117 141 109 109 106 11310980 11186 111 12096103 125 101 132 113 108 10684 10884 129 116 107 112941369211792112 119103 11297 121128 133ordered data set denote by x(1) x(2) . 9697 101 103 103 103 104 105 106107 107 107 108 108 108 109 109111 111 112 112 112 112 112 113117 117 119 120 120 121 123 125129 132 133 134 136 138 140 1405. listopadu 20158 / 138
Descriptive statisticsExample - data setmeasured values denote by x1 , x2 . . . , xn , now n 62.1079210713810413496141 105 111 11296 103 14072 123 140 112 127 120 106108 117 141 109 109 106 11310980 11186 111 12096103 125 101 132 113 108 10684 10884 129 116 107 112941369211792112 119103 11297 121128 133ordered data set denote by x(1) x(2) . 9697 101 103 103 103 104 105 106107 107 107 108 108 108 109 109111 111 112 112 112 112 112 113117 117 119 120 120 121 123 125129 132 133 134 136 138 140 1405. listopadu 20158 / 138
Descriptive statisticsFrequency distributionIf the values are often repeated we can produce so calledfrequency table.If the variable is continuous and n (number of observations)is large, it is advisable to divide the range of values into Mintervals with endpointsa a0 a1 a2 . aM 1 aM b.all the observations from a interval can be represented byone value (usually the center of the interval) xi , i 1, . . . , k.let ni denotes number of observations that falls to intervalhai 1 , ai ), i 1, . . . , M – so called absolute frequency(Intervals are called classes).cumulative frequency Ni gives the number of observationsin the (i-th) and all the preceding classesnumbers ni /n gives relative frequency.5. listopadu 20159 / 138
Descriptive statisticsFrequency distributionIf the values are often repeated we can produce so calledfrequency table.If the variable is continuous and n (number of observations)is large, it is advisable to divide the range of values into Mintervals with endpointsa a0 a1 a2 . aM 1 aM b.all the observations from a interval can be represented byone value (usually the center of the interval) xi , i 1, . . . , k.let ni denotes number of observations that falls to intervalhai 1 , ai ), i 1, . . . , M – so called absolute frequency(Intervals are called classes).cumulative frequency Ni gives the number of observationsin the (i-th) and all the preceding classesnumbers ni /n gives relative frequency.5. listopadu 20159 / 138
Descriptive statisticsFrequency distributionIf the values are often repeated we can produce so calledfrequency table.If the variable is continuous and n (number of observations)is large, it is advisable to divide the range of values into Mintervals with endpointsa a0 a1 a2 . aM 1 aM b.all the observations from a interval can be represented byone value (usually the center of the interval) xi , i 1, . . . , k.let ni denotes number of observations that falls to intervalhai 1 , ai ), i 1, . . . , M – so called absolute frequency(Intervals are called classes).cumulative frequency Ni gives the number of observationsin the (i-th) and all the preceding classesnumbers ni /n gives relative frequency.5. listopadu 20159 / 138
Descriptive statisticsFrequency distributionIf the values are often repeated we can produce so calledfrequency table.If the variable is continuous and n (number of observations)is large, it is advisable to divide the range of values into Mintervals with endpointsa a0 a1 a2 . aM 1 aM b.all the observations from a interval can be represented byone value (usually the center of the interval) xi , i 1, . . . , k.let ni denotes number of observations that falls to intervalhai 1 , ai ), i 1, . . . , M – so called absolute frequency(Intervals are called classes).cumulative frequency Ni gives the number of observationsin the (i-th) and all the preceding classesnumbers ni /n gives relative frequency.5. listopadu 20159 / 138
Descriptive statisticsFrequency distributionIf the values are often repeated we can produce so calledfrequency table.If the variable is continuous and n (number of observations)is large, it is advisable to divide the range of values into Mintervals with endpointsa a0 a1 a2 . aM 1 aM b.all the observations from a interval can be represented byone value (usually the center of the interval) xi , i 1, . . . , k.let ni denotes number of observations that falls to intervalhai 1 , ai ), i 1, . . . , M – so called absolute frequency(Intervals are called classes).cumulative frequency Ni gives the number of observationsin the (i-th) and all the preceding classesnumbers ni /n gives relative frequency.5. listopadu 20159 / 138
Descriptive statisticsFrequency distributionIf the values are often repeated we can produce so calledfrequency table.If the variable is continuous and n (number of observations)is large, it is advisable to divide the range of values into Mintervals with endpointsa a0 a1 a2 . aM 1 aM b.all the observations from a interval can be represented byone value (usually the center of the interval) xi , i 1, . . . , k.let ni denotes number of observations that falls to intervalhai 1 , ai ), i 1, . . . , M – so called absolute frequency(Intervals are called classes).cumulative frequency Ni gives the number of observationsin the (i-th) and all the preceding classesnumbers ni /n gives relative frequency.5. listopadu 20159 / 138
Descriptive statisticsExample - frequency distributionInterval 80h80, 90)h90, 100)h100, 110)h110, 120)h120, 130)h130, 140) 140xi 758595105115125135145absol. ni1481814854ni /n0.0160.0650.1290.2900.2260.1290.0810.065cumul. Ni15133145535862Ni /n0.0160.0810.2100.5000.7260.8550.9351.0005. listopadu 201510 / 138
Descriptive statisticsHistogramgraphic display of frequency distributionwe assign to each interval a box, such that its area isproportional to the frequency of the intervalmost often the intervals have equal length (oftenappropriately rounded), then the hight of the boxescorresponds with the frequencies.problem: choice of the number of intervals Mwe can use e.g. Sturges rule:.M 1 3.3 log10 (n) 1 log2 (n)for our example: 1 log2 (62) 6.955. listopadu 201511 / 138
Descriptive statisticsHistogramgraphic display of frequency distributionwe assign to each interval a box, such that its area isproportional to the frequency of the intervalmost often the intervals have equal length (oftenappropriately rounded), then the hight of the boxescorresponds with the frequencies.problem: choice of the number of intervals Mwe can use e.g. Sturges rule:.M 1 3.3 log10 (n) 1 log2 (n)for our example: 1 log2 (62) 6.955. listopadu 201511 / 138
Descriptive statisticsHistogramgraphic display of frequency distributionwe assign to each interval a box, such that its area isproportional to the frequency of the intervalmost often the intervals have equal length (oftenappropriately rounded), then the hight of the boxescorresponds with the frequencies.problem: choice of the number of intervals Mwe can use e.g. Sturges rule:.M 1 3.3 log10 (n) 1 log2 (n)for our example: 1 log2 (62) 6.955. listopadu 201511 / 138
Descriptive statisticsHistogramgraphic display of frequency distributionwe assign to each interval a box, such that its area isproportional to the frequency of the intervalmost often the intervals have equal length (oftenappropriately rounded), then the hight of the boxescorresponds with the frequencies.problem: choice of the number of intervals Mwe can use e.g. Sturges rule:.M 1 3.3 log10 (n) 1 log2 (n)for our example: 1 log2 (62) 6.955. listopadu 201511 / 138
Descriptive statisticsHistogramgraphic display of frequency distributionwe assign to each interval a box, such that its area isproportional to the frequency of the intervalmost often the intervals have equal length (oftenappropriately rounded), then the hight of the boxescorresponds with the frequencies.problem: choice of the number of intervals Mwe can use e.g. Sturges rule:.M 1 3.3 log10 (n) 1 log2 (n)for our example: 1 log2 (62) 6.955. listopadu 201511 / 138
Descriptive statisticsExample - histogram05četnost1015Histogram IQ80100120140IQ5. listopadu 201512 / 138
Descriptive statisticsCharacteristics of locationCharacteristic of locationallows to characterize the level of a variable by one number evaluation, how the observations are small or large.it should hold for a characteristic m of a data set x , that itnaturally changes with the change of the scale, i.e. forarbitrary constants a, b:m(a · x b) a · m(x) bif we add a constant b to all observations, then thecharacteristic gets larger by bif we multiple each observation by a, then the resultingcharacteristic gets bigger a-times5. listopadu 201513 / 138
Descriptive statisticsCharacteristics of locationCharacteristic of locationallows to characterize the level of a variable by one number evaluation, how the observations are small or large.it should hold for a characteristic m of a data set x , that itnaturally changes with the change of the scale, i.e. forarbitrary constants a, b:m(a · x b) a · m(x) bif we add a constant b to all observations, then thecharacteristic gets larger by bif we multiple each observation by a, then the resultingcharacteristic gets bigger a-times5. listopadu 201513 / 138
Descriptive statisticsCharacteristics of locationAritmetic meann1X1x xi (x1 x2 . . . xn )nni 11for our example: x 62(107 141 . . . 94) 111.0645sensitive to outliers. Only for quantitative scales.can be computed from the frequency table as a weightedaveragePMM 1X1 · 75 4 · 85 . . . 4 · 145 i 1 ni xix ni xi P 111.7742Mn62nii 1i 1number of onesfor zero-one variable: number relative frequencyof zeros andones(percent) of ones (observations with the given property).for our example yi 0 (i-th pupil is a man) ,32yi 1 (i-th pupil is a female): y 62 0.5165. listopadu 201514 / 138
Descriptive statisticsCharacteristics of locationAritmetic meann1X1x xi (x1 x2 . . . xn )nni 11for our example: x 62(107 141 . . . 94) 111.0645sensitive to outliers. Only for quantitative scales.can be computed from the frequency table as a weightedaveragePMM 1X1 · 75 4 · 85 . . . 4 · 145 i 1 ni xix ni xi P 111.7742Mn62nii 1i 1number of onesfor zero-one variable: number relative frequencyof zeros andones(percent) of ones (observations with the given property).for our example yi 0 (i-th pupil is a man) ,32yi 1 (i-th pupil is a female): y 62 0.5165. listopadu 201514 / 138
Descriptive statisticsCharacteristics of locationAritmetic meann1X1x xi (x1 x2 . . . xn )nni 11for our example: x 62(107 141 . . . 94) 111.0645sensitive to outliers. Only for quantitative scales.can be computed from the frequency table as a weightedaveragePMM 1X1 · 75 4 · 85 . . . 4 · 145 i 1 ni xix ni xi P 111.7742Mn62nii 1i 1number of onesfor zero-one variable: number relative frequencyof zeros andones(percent) of ones (observations with the given property).for our example yi 0 (i-th pupil is a man) ,32yi 1 (i-th pupil is a female): y 62 0.5165. listopadu 201514 / 138
Descriptive statisticsCharacteristics of locationAritmetic meann1X1x xi (x1 x2 . . . xn )nni 11for our example: x 62(107 141 . . . 94) 111.0645sensitive to outliers. Only for quantitative scales.can be computed from the frequency table as a weightedaveragePMM 1X1 · 75 4 · 85 . . . 4 · 145 i 1 ni xix ni xi P 111.7742Mn62nii 1i 1number of onesfor zero-one variable: number relative frequencyof zeros andones(percent) of ones (observations with the given property).for our example yi 0 (i-th pupil is a man) ,32yi 1 (i-th pupil is a female): y 62 0.5165. listopadu 201514 / 138
Descriptive statisticsCharacteristics of locationAritmetic meann1X1x xi (x1 x2 . . . xn )nni 11for our example: x 62(107 141 . . . 94) 111.0645sensitive to outliers. Only for quantitative scales.can be computed from the frequency table as a weightedaveragePMM 1X1 · 75 4 · 85 . . . 4 · 145 i 1 ni xix ni xi P 111.7742Mn62nii 1i 1number of onesfor zero-one variable: number relative frequencyof zeros andones(percent) of ones (observations with the given property).for our example yi 0 (i-th pupil is a man) ,32yi 1 (i-th pupil is a female): y 62 0.5165. listopadu 201514 / 138
Descriptive statisticsCharacteristics of locationAritmetic meann1X1x xi (x1 x2 . . . xn )nni 11for our example: x 62(107 141 . . . 94) 111.0645sensitive to outliers. Only for quantitative scales.can be computed from the frequency table as a weightedaveragePMM 1X1 · 75 4 · 85 . . . 4 · 145 i 1 ni xix ni xi P 111.7742Mn62nii 1i 1number of onesfor zero-one variable: number relative frequencyof zeros andones(percent) of ones (observations with the given property).for our example yi 0 (i-th pupil is a man) ,32yi 1 (i-th pupil is a female): y 62 0.5165. listopadu 201514 / 138
Descriptive statisticsCharacteristics of locationModex̂ - most frequent valuecan be used even for nominal and ordinal scalesnot necessarily uniquefor our example:5. listopadu 201515 / 138
Descriptive statisticsCharacteristics of locationModex̂ - most frequent valuecan be used even for nominal and ordinal scalesnot necessarily uniquefor our example:5. listopadu 201515 / 138
Descriptive statisticsCharacteristics of locationModex̂ - most frequent valuecan be used even for nominal and ordinal scalesnot necessarily uniquefor our example:5. listopadu 201515 / 138
Descriptive statisticsCharacteristics of locationModex̂ - most frequent valuecan be used even for nominal and ordinal scalesnot necessarily uniquefor our 86929292949697 101 103 103 103 104 105 106107 107 107 108 108 108 109 109111 111 112 112 112 112 112 113117 117 119 120 120 121 123 125129 132 133 134 136 138 140 1405. listopadu 201515 / 138
Descriptive statisticsCharacteristics of locationModex̂ - most frequent valuecan be used even for nominal and ordinal scalesnot necessarily uniquefor our 86929292949697 101 103 103 103 104 105 106107 107 107 108 108 108 109 109111 111 112 112 112 112 112 113117 117 119 120 120 121 123 125129 132 133 134 136 138 140 140x̂ 1125. listopadu 201515 / 138
Descriptive statisticsCharacteristics of locationMedianx̃ - number that divides the ordered sample into two equalhalves, is located in the middle of the ordered samplex̃ x( n 1 )2 1 x̃ x( n2 ) x( n2 1)2for n oddfor n evenrobust - not influenced by large changes of a few values.often also for ordinal scale. For our example:5. listopadu 201516 / 138
Descriptive statisticsCharacteristics of locationMedianx̃ - number that divides the ordered sample into two equalhalves, is located in the middle of the ordered samplex̃ x( n 1 )2 1 x̃ x( n2 ) x( n2 1)2for n oddfor n evenrobust - not influenced by large changes of a few values.often also for ordinal scale. For our example:5. listopadu 201516 / 138
Descriptive statisticsCharacteristics of locationMedianx̃ - number that divides the ordered sample into two equalhalves, is located in the middle of the ordered samplefor n oddx̃ x( n 1 )2 1 x̃ for n evenx( n2 ) x( n2 1)2robust - not influenced by large changes of a few values.often also for ordinal scale. For our 05109112123140961061091131251405. listopadu 201516 / 138
Descriptive statisticsCharacteristics of locationMedianx̃ - number that divides the ordered sample into two equalhalves, is located in the middle of the ordered samplefor n oddx̃ x( n 1 )2 1 x̃ x( n2 ) x( n2 1)for n even2robust - not influenced by large changes of a few values.often also for ordinal scale. For our 10711111712984101107111117132x̃ 692104108112121138 1x(31) x(32) 110294105109112123140961061091131251405. listopadu 201516 / 138
Descriptive statisticsCharacteristics of locationQuantiles: percentiles, deciles, quartilesα-quantile xα ( α (0, 1)) - Dividing ordered data into two part,such that α-ratio of the smallest values is smaller than xαxα x(dαne) ,where dae denotes a, if it is a integer, otherwise the nearestlarger integer.special quantiles:percentiles: α 0.01, 0.02, . . . , 0.99deciles: α 0.1, 0.2, . . . , 0.9quartiles: α 0.25, 0.5, 0.751-st (lower) quartile is denoted by Q1 x0.253-rd (upper) quartile is denoted by Q3 x0.75median is the 50% quantile, 50-th percentile, 5-th decile a2-nd quartile5. listopadu 201517 / 138
Descriptive statisticsCharacteristics of locationQuantiles: percentiles, deciles, quartilesα-quantile xα ( α (0, 1)) - Dividing ordered data into two part,such that α-ratio of the smallest values is smaller than xαxα x(dαne) ,where dae denotes a, if it is a integer, otherwise the nearestlarger integer.special quantiles:percentiles: α 0.01, 0.02, . . . , 0.99deciles: α 0.1, 0.2, . . . , 0.9quartiles: α 0.25, 0.5, 0.751-st (lower) quartile is denoted by Q1 x0.253-rd (upper) quartile is denoted by Q3 x0.75median is the 50% quantile, 50-th percentile, 5-th decile a2-nd quartile5. listopadu 201517 / 138
Descriptive statisticsCharacteristics of locationQuantiles: percentiles, deciles, quartilesα-quantile xα ( α (0, 1)) - Dividing ordered data into two part,such that α-ratio of the smallest values is smaller than xαxα x(dαne) ,where dae denotes a, if it is a integer, otherwise the nearestlarger integer.special quantiles:percentiles: α 0.01, 0.02, . . . , 0.99deciles: α 0.1, 0.2, . . . , 0.9quartiles: α 0.25, 0.5, 0.751-st (lower) quartile is denoted by Q1 x0.253-rd (upper) quartile is denoted by Q3 x0.75median is the 50% quantile, 50-th percentile, 5-th decile a2-nd quartile5. listopadu 201517 / 138
Descriptive statisticsCharacteristics of locationQuantiles: percentiles, deciles, quartilesα-quantile xα ( α (0, 1)) - Dividing ordered data into two part,such that α-ratio of the smallest values is smaller than xαxα x(dαne) ,where dae denotes a, if it is a integer, otherwise the nearestlarger integer.special quantiles:percentiles: α 0.01, 0.02, . . . , 0.99deciles: α 0.1, 0.2, . . . , 0.9quartiles: α 0.25, 0.5, 0.751-st (lower) quartile is denoted by Q1 x0.253-rd (upper) quartile is denoted by Q3 x0.75median is the 50% quantile, 50-th percentile, 5-th decile a2-nd quartile5. listopadu 201517 / 138
Descript
1 Descriptive statistics - we make conclusions only for the studied data set from the observed data (we measured all the units in the population we want to describe) 2 Mathematical (inferential) statistics - studied data set is treated as a sample data - set of units randomly and independently selected from target population that is large