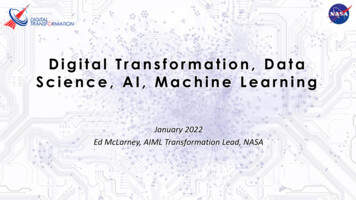
Transcription
Digital Transformation, DataScience, AI, Machine LearningJanuary 2022Ed McLarney, AIML Transformation Lead, NASA
What is Digital Transformation?Digital Transformation has already changed our world Employing digital technologies (e.g.,AI, mobile, cloud, data) to change aprocess, product, or capability sodramatically (e.g., real-time,intelligent, personalized, anywhere,anytime) that it’s unrecognizablecompared to its traditional formTRADITIONALDIGITIZEDTRANSFORMED & technology discovery and convergence is accelerating change! Cloud ComputingMobile AccessAutomation and RoboticsBig Data / Data Mining /AnalyticsArtificial Intelligence / MachineLearningModel-Based SystemsEngineeringAgile Software Development /DevOps / DevSecOps Internet of Things / SensorsDigitized Manufacturing (e.g., 3-DPrinting)Augmented Reality / VirtualRealityMultidisciplinary Modeling andSimulationHigh Performance ComputingCollaboration PlatformsSocial Media / CrowdsourcingVirtual MeetingsAnd more 2
Home & Work: Common DenominatorsSimilar challenges at bothhome and at work: More demands More complex & complicated More interactions Faster paced More frequent changes More budget pressuresThe same kinds of digital solutionsthat are helping us navigate modern life at homewill also transform our work world3
WHY: Enterprise DT GoalsTransform NASA’s WorkTransform NASA’s WorkforceTransform NASA’s WorkplaceDeliver increasingly complexmissions leveraging increasinglycomplicated partnerships, onshorter timelines to achieve bolderoutcomes that inspire the worldCreate a seamless, integrated, andinclusive employee experience thatenergizes our people by feelingconnected to the NASA enterprise,continuously grow, and take pride inrapidly delivering high-value workOptimize a 21st Century cyberphysical work environment thatpowers flexible, adaptable,efficient, and effective employee/ partner teaming.4
WHAT: Enterprise DT Essential ElementsStrategic ThrustsGrand Challenges“Real world”transformation pathfindersthat serve as a lightningrod to catalyze enterpriseDT adoption andintegration and delivertangible end-to-endoutcomes, using integrateddigital ionArchitectureCatalysts for transformation key shared enterprise-leveldigital enablers that orgs canuse to acceleratetransformation outcomesTransformation ArchitectureIntegration plan for how those digitalenablers can/should work together topower our future transformed NASA
WHAT:EnterpriseDT ThrustsWORKProcess Transformation (PTx):Transform our products and processes tomaximize our efficiency and effectivenessto enable bolder missions fasterDT O u tco me s T i e rArtificial Intelligence /Machine Learning (AI/ML):Harness machine capabilities to augmenthuman intelligence in an era of big dataDT A c c e l e rant s T i e rData: Ensure the data weneed is Findable, Accessible,Interoperable, andReusable (FAIR) to powerdata-driven decision makingWORKPLACEDT Fo u ndat ions T ierCollaboration:Enable agile teaming via seamless, secureinternal and external collaborationModel Based Everything (MBx):Employ digital models to enableour people to address increasingcomplexity, scope, speed,uncertainty & changesCulture & Workforce:Foster digital savvy,enterprise connection,and growth mindsetsWORKFORCE6
WHERE: Enterprise DTEarlyWins(selected)WORKProcess Transformation Process Transformation Playbook – guidelines to makePTx easy and repeatable for all NASA orgs; test oninitial use cases including Robotic Process AutomationAI/ML:DataDTcasesO u tcoT i e r Based Everything (MBx): High priority (learning) use– me sModelNatural Language Processing for Orion MBSE Digital Twin Pilot –Requirements Analysis, Inverse Design ofTest common models and standardsMaterials, Extreme Weather ForecastingAgency-wide for MBSE w/Orion Data-Driven Decision Lens – IntegrateNASA data into central analytics/vizplatform to enable holistic, enterpriselevel executive data driven decisionmaking; less rework; better risk forecast;what-if analysis Agency Data Analytics Platform Integrate/scale COTSto C EWanalyticsO R K toolsPLApower data-driven decision makingDT A c c e l e rant s T i e rCollaborationCulture & Workforce SWARM Innovation –Rapidly develop ITAR File Sharing – ISS w/ESAinnovation skills andprototype of 2-way secure sensitive file mechanisms in keysharing for rapid external collabearly adopterDT Fo u ndat ionsT i e rImmersive 3D Visualization community Remote– Visualize engineering models via 3DWORKFORCEheadsets at remotelocations7
NASA AI/ML Strategy – Elevator PitchContext: NASA is forming a Digital Transformation (DT) Strategy and Roadmap, led by the Office of Chief Technologist and Office of ChiefInformation Officer. This strategy includes AI/ML as one of six key strategic thrusts.NASA has a rich history of applying artificial intelligence (AI) to our hardest problems, such as autonomous behaviors in Mars rovers,deep analysis of space suit data, or image analysis to understand material strength. With the advent of powerful, plentiful, andaffordable AI in business and industry, NASA is crafting a strategy to use AI as an accelerant for all NASA missions and business functions.Strategy: As part of NASA’s overall Digital Transformation, NASA's AI strategy includes: Apply: Solve relevant mission and mission support problems via AI / ML.Teamwork: Lead and synchronize NASA AI/ML via an open Agency AI / ML community.Reskill: Expand AI training, education, hiring, and retention across the workforce.Tools: Assess, recommend, and establish AI / ML platforms for NASA-wide adoption.Data: AI-enabled! Establish secure, authoritative access to the right data.Outreach: Make selected data and problems available for public / partner AI / ML work.Adapt: Leverage industry AI / ML work and adapt it to NASA use rather than reinventing.Scale: Plan to promote selected AI / ML capabilities from pilot to production operations.The AI/ML team is from across the Agency with over 60 active members; additional contributors are always welcome.Contact:Ed McLarney (edward.l.mclarney@nasa.gov), Nikunj Oza (nikunj.c.oza@nasa.gov) or Herb Schilling (hschilling@nasa.gov)8
AIML Intersection with Missions and Mission SPT(Examples, not pportCommand & Control AutonomyFor Moon / Mars RobotsEnable ScaledAir Traffic ControlOn-Board Analysis forScience SensorsCondition Based MaintenanceFor Space HabitatsAutonomous NavigationFor UAS/UAMEarly Warning of SevereStorms via ML Image AnalysisAI-Assisted ProjectManagementAI-EnabledVehicle DesignHorizon Scanning viaAI Recommendation EnginesAIML TraditionalHybrid AnalysisML-EnabledRequirements OptimizationAI-Assisted SystemEngineeringBot Automation ofRepetitive ProcessesCondition-Based MaintenanceFor FacilitiesML IT SecurityMonitoringAI-Enabled HR Processes:Resume Review, MoreAI-enabled LessonsLearned RecommendationsML AssistedFraud Detection9
Above Anvil Cirrus Plumes (AACP) ML ProjectPurpose: Identify above anvil cirrus plumes (AACP) in satelliteimagery.Problem: AACPs are typically 31 minute precursors to severeweather such as hail, high winds, and tornadoes. They alsoeject water into the stratosphere which acts as a greenhousegas.AObjective: Identify AACP occurrences in satellite imagery toassist with weather forecasting and long-term climate studies.B(A) Model input with infrared GOES 16 imagery “sandwiched” as a colormap on top of visibleimagery. (B) Model output with semantic segmentation AACP probability prediction overlain inred over visible imagery. Rectangular markings represent SME-labeled non-AACP storm updraftswhile circular markings represent AACP updrafts in both images.Customer/SME: Dr. Kristopher Bedka, Science DirectorateData Scientist: Charles LilesApproach: Deep learning U-net’s for semantic segmentation ofAACPs.Status: Exploring the use of LSTM layers in a U-net to accountfor temporal component of satellite imagery.10U-Net Architecture
Intelligent Contingency ManagementPurpose: UAM Mission - Safely fly from point A to point B under all vehicle-allowable weather conditions,in a high-density airspace complex urban environment, including off-nominal situations without directhuman intervention, i.e. autonomousProblem: For an arbitrary set of sensors and actuators, there is no robust, repeatable method ofidentifying the necessary data needed to train and verify that a vehicle can intelligently address anemergency.Objective: Decision making under uncertainty and incomplete information1. Explore machine learning for intelligent contingency management, with a focus onassessing/projecting vehicle capability and maintaining nominal performance via reinforcementlearning2. Develop vehicle intelligent contingency management system architecture at a functional level andvalidate against a specific UAM-class vehicle3. Incorporate (1) and (2) into an evolving toolset for an autonomous vehicleCustomer/SME: Dr. Irene Gregory (ARMD TTT-Autonomous Systems)Data Scientist: Dr. Newton CampbellApproach:- Develop probabilistic inference techniques to detect changes in vehicle or mission state andcontextualize emergencies.- Develop reinforcement learning and knowledge reasoning techniques to create and assess theperformance of contingency plans.Status:- Completed Year 1 exploration; Preparing high-quality simulation platform for program experimentation- Publishing Problem Specification to AIAA Aviation 2020.11
Watson ExplorerText AnalyticsWatson Explorer User InterfacePurpose: Provide users with a way to intuitively interface withtheir textual data.Problem: NASA has lots of textual data in search of avisualization mechanism.Time SeriesObjective: Perform cognitive search and content analysis foraccessing insight from large amounts of structured andunstructured text. Rapidly identify trends, expert connections,technology gap exploration and connect cross-domainresearch.Concept LinksHeat MapsCustomer/SME: Bob Beil/Delmar Foster (NESC-Kennedy)FacetPairsNLP Data Scientist: Ted SidehamerApproach: WEX is installed on a dedicated server to takeadvantage of its computing and visualization capabilities.Status: Current work focused on FORs: Findings, Observations,Recommendations System attempting to identify previousFORs related to current evaluations. Largest collection - SpaceRadiation: 600k records.FacetedSearchWordClouds12
Working with NASA Internships: https://intern.nasa.gov/– One term at a time: Summer, Fall, Winter/Spring Pathways internships - https://www.nasa.gov/careers/pathways– At least a year– Part time school and internship in parallel– Goal – convert to government civilian upon successful completion and HR approval Government civilian positions – https://www.usajobs.gov/Search/Results?k nasa– Availability comes and goes; be persistent! Contractor positions – see company career / job sites– Sometimes faster & higher availability Term projects or Capstone projects – willing to work with professors & students– National Institute for Aerospace is an easy way to handle the logistics Hints:– Tailor your resume!– Demonstrate strong motivation and passion for NASA13
Thank you!Ed McLarneyedward.l.mclarney@nasa.govI’m in LinkedIn too14
Optimize a 21st Century cyber-physical work environment that powers flexible, adaptable, efficient, and effective employee / partner teaming. Transformation Architecture Grand . ML IT Security Monitoring AI-enabled Lessons Learned Recommendations ML Assisted Fraud Detection AI-Enabled HR Processes: Resume Review, More. 10