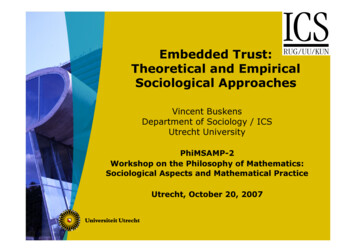
Transcription
Embedded Trust:Theoretical and EmpiricalSociological ApproachesVincent BuskensDepartment of Sociology / ICSUtrecht UniversityPhiMSAMP-2Workshop on the Philosophy of Mathematics:Sociological Aspects and Mathematical PracticeUtrecht, October 20, 2007
Overview Field of application Trust in embedded settings Theoretical approaches Rational choice theory (Behavioral) game theory Learning Empirical approaches Laboratory experiments Surveys Vignette experiments Conclusion
Trust in Words Placing trust means that a buyer (trustor)decides to hand over some resources at thedisposal of a seller (trustee) If the seller handles these resources welland is trustworthy, they both profitcompared to the no trust situation The seller cannot guarantee to acttrustworthy and has an incentive to actuntrustworthy The buyer has to decide first
Trust by Example A buyer of a rare first edition offered ateBay has to decide whether to buy thisedition from a seller and to send the money The seller (after receiving the money) hasto decide whether or not to send this rarefirst edition to the buyer If the seller ships the first edition and thisedition is in correspondence with theclaimed specifications, both buyer and sellerare happier after the deal than before thedeal If the seller does not ship the book, he cantry to sell it again, while the buyer lost themoney
The Trust GameBuyerTrustNo trustSeller / supplierAbuse! P1 "#P % 2 &! S1 "#T % 2&HonorS1 P1 R1! R 1 " P2 R2 T2#R % 2&
Embedded Trust Many trust situations (and other socialand economic interactions) do not occur inisolated encounters but are embedded ina larger context of interactions(Granovetter 1985) Actors deal with each other repeatedly Actors deal with partners of other actors Therefore, we need to extend thepredictions for trust situations toembedded settings
Two Levels and Two Mechanisms Two levels of embeddedness Dyadic embeddedness Network embeddedness Two mechanisms Learning Buyers obtain information aboutpast behavior of sellers Control Buyers can inform other buyersabout past behavior of sellers andsubsequent decisions can bebased on this information
Summary Research Problem Distinguish between different embeddednesseffects on trust theoretically arningPrior ownexperiencesPrior third-partyexperiencesControlOwn sanctionopportunitiesSanctionopportunities w.r.t.third parties
Existing Formal essAdaptive learning modelsInformation diffusion modelsLearning and Game-theoretic models withcontrolincomplete information (hardly innetworks)ControlRepeated games with completeinformation
Remember the Trust GameBuyerTrustNo trustSeller / supplierAbuse! P1 "#P % 2 &! S1 "#T % 2&HonorS1 P1 R1! R 1 " P2 R2 T2#R % 2&
Repeated Games Buyer and seller are involved in a series(rounds) of Trust Games After each round, the same Trust Game isplayed again with probability w If w is large enough, there are equilibriain which trust is possible, because a buyercan threaten the seller with not trustinganymore in the future. If the loss of thisthreat is larger than the short-turn gain ofabusing trust, the seller will honor trust.
Trigger Strategies Buyers trust as long as trust is honored, but neveragain after trust has been abused once. Sellers honortrust as long as trust has always been placed andnever after trust is not placed once. It can be derived that these strategies are anequilibrium if and only ifw"T2 ! R2T2 ! P2 Interpretation: the more restrictive this inequality, theless likely a buyer will trust Hypotheses: the larger R2, the more likely the buyer trusts the larger w, the more likely the buyer trusts
Repeated Games in Networks Networks of buyers play repeated TrustGames with the same seller Networks represent information exchangepossibilities112345B2345D116A623456C23456
Related Hypotheses Again using a model based on triggerstrategies (buyers always trust as long asthey have no information that the sellerever abused trust), we derive thefollowing hypotheses The denser the network, the more buyerswill trust The more relations a buyer has, the morethis buyer will trust We focus here on hypotheses aboutplacing trust, but similar hypotheses canbe derived for honoring trust
Incomplete Information Imagine some sellers exist who neverabuse trust For one-shot games, there is quitesome evidence that actors not onlycare about there own money, but alsoin some way about other peoplesmoney (e.g., fairness preferences) Different tools Game-theoretic models withincomplete information using e.g.,Bayesian updating Learning models that use boundedlyrational approaches to actors decisionmaking processes
New Hypotheses Trust increases if a buyer has less to losein the Trust Game (e.g., S1 is larger) Trust increases with positive ownexperiences and decreases with negativeown experiences (dyadic learning) Trust increases with positive informationfrom third parties and decreases withnegative information from third parties(network learning)
Hypotheses ngTrust decreaseswith the buyer’srisk and increaseswith positiveexperiences with asellerTrust increase withthe density of thebuyer’s network andthe amount of positiveinformation receivedthrough the networkControlTrust decreaseswith the temptationto abuse trust andincreases with thelikelihood of futureinteractionsTrust increases withthe density of thebuyer’s network andthe number ofrelations of a buyer
Overview of Our DataLaboratorySurvey of IT Vignetteexperiments transactions YesYesNetworklearningNetworkcontrolYesYes- Learningand controlhard todisentangleYesYes
Laboratory Experiment Subjects (mostly students) play repeatedTrust Games in the laboratory Interactions are with actual otherparticipants in the laboratory Interactions are anonymous Complete game structure is provided inthe instruction Points that can be earned in the gamesrepresent actual money for the subjects
Interaction Structure Two buyers play with the same seller for 15rounds Information about past might be distributedBuyerSellerOtherbuyer
Laboratory Experiment Six sessions with 6 times 18 148 subjects In total 2160 games played Two conditions Local information: buyers only haveinformation about their own interactionswith the seller Full information: buyers also haveinformation about transactions of anotherbuyer with the same seller
Random-Effects Logistic Regression Predict trusting behavior Information condition Own last experience Other buyers last experience (ifapplicable) Number of the round Additional dummies for two last rounds Interactions of last round dummieswith information condition Random effects for clustering within sellers
Results Logistic Regression Effects of embeddedness variables on trustInformation conditionNo net effectPrevious own: abuse Previous own: honor Previous other: abuse Previous other: honor Rounds to go Round 14 Round 15 Information round 140Information round 15
Evidence Experiment in Words Own experience (dyadic learning) is veryimportant Third-party information (network learning) isalso taken into account although effects area bit smaller Number of rounds to go has a positive effecton trustfulness (dyadic control) Trust decreases dramatically in last round(dyadic control) Effects of number of rounds is hardly effectby the condition (no evidence for networkcontrol) Experimental condition does not have aneffect on top of this explanatory model
Overview of Results kcontrolNo effectSurveyVignetteexperiment
Survey of IT Transactions We obtain completed questionnaires from 788buyers of IT products (SMEs) Some buyers complete the questionnaire fortwo transactions which resulted in 1252transactions Data on transaction management (search,contracting) transaction characteristics (price, risks) dyadic embeddedness (learning andcontrol) (network embeddedness) characteristics of buyer and supplier
Trust and Embeddedness Variables “Lack of trust” measured by time and moneyspent for searching, negotiating, andcontracting (ex ante management) Dyadic embeddedness variables Information on prior transactions Expectation for future transactions atthe time of the focal transaction Network embeddedness variables Information on other buyers of thesame supplier and their relations Geographic distance between buyer andsupplier
Operationalizations Factor score for ex ante management Dummy for past transactions Variation in amount of past / satisfactiondid not matter Hardly any negative evaluations ofsuppliers Five-point scale for future expectations Interaction between past and future Number of other buyers known of the samesupplier Density of the network of other buyers Geographic distance We control also for other transactioncharacteristics
Interaction Past and Future Ex ante management is expected to besmaller for transactions with a longerfuture because of arguments given above However, ex ante management can alsobe expected to be larger if you think thatyou start a long-term relationship (ratherthan a one-time transaction) Therefore, especially in first transactionstheir will be also a positive effect of futuretransactions on ex ante investments, butin particular in later transactions, thenegative effect of future should be visible
Results Linear Regression Effects of embeddedness variables on exante managementPast Future0Future past Number other buyers0Density buyer network0Geographical distance
Evidence Survey in Words Own positive experiences (dyadic learning)induces trust Expected future transactions also inducemore trust as can be seen from the effect offuture in later transactions (dyadic control) Trust is not affected by the information wehave about other buyers of the samesupplier (these data seemed not thatreliable) so we cannot conclude from thiswhether there is no effect or the data is notgood enough to find an effect Trust is affected by geographical distance.Network embeddedness (learning or control)might be part of the mechanisms
Overview of Results problemsNo effectVignetteexperiment
Buying-a-Used-Car Vignettes 125 Dutch and US students 1249 comparisons of used-car dealers Data on choice of a dealer (transaction characteristics) dyadic embeddedness (learning andcontrol disentangled) network embeddedness (learning andcontrol disentangled) characteristics of subjects
Trust and Embeddedness Variables Choice between two dealers Dyadic embeddedness variables Past experiences (learning) Expected future transactions: movingbuyer (control) Network embeddedness variables Density: dealer well-known garage Positive information from other buyers(learning) Close social tie with dealer (control)
A Pair of Vignettes You can buy a car for 4000. You can buy a car for 4000. You never bought a car from You bought a car from TheThe Autoshop before.Autoshop before and youwere satisfied. You do not expect to move You will move to the otherout of town soon.side of the country in a fewweeks. The Autoshop is an unknown The Autoshop is a well-knowngarage in your neighborhood.garage and has many customers in your neighborhood. You have friends who bought As far as you know, none ofa car from The Autoshopyour friends have bought abefore and they werecar from The Autoshopsatisfied.before. You do not have a close social The owner of the garage andyou are members of the samelink with the owner of Thefootball team.Autoshop.
Statistical Model Random utility model. Probit model on choices for vignettes. Coefficients are interpretable as indicatorsfor the increase in utility assigned to avignette related to the given variables. Standard errors modified for clustering.
Results Vignette ExperimentAllChicagoUtrechtTilburgDyadic learningDyadic controlDensityNetwork learningNetwork 0.300.73**0.86**0.51*Number of pairs1249400720129** and * represent two -sided significance at p 0.01 and p 0.05respectively.
Results Vignettes in Words Probability of dealer to be chosen increaseswith positive past experiences (dyadic learning) expected future transactions (dyadiccontrol) density (network learning or control) positive third-party information (networklearning) the presence of a close social tie with thedealer (network control)
Overview of firmedConfirmedNetworkcontrolNo effectSeriousmeasurementproblemsConfirmed
Discussion Hardly dependence of effects onuncertainty just as in the vignetteexperiment Is there a trade-off between complexityand rationality? What about “learning” to play the game? Are network effects stronger ininteractions with strangers?
Acknowledgement The research presented here has beendone in collaboration with many otherresearchers in our research groupincluding Werner Raub Jeroen Weesie Chris Snijders Ronald Batenburg Frits Tazelaar Gerrit Rooks Davide Barrera Joris van de Veer More information on our research line andspecifically the research presented herecan be found at www.fss.uu.nl/soc/iscore
eBay has to decide whether to buy this edition from a seller and to send the money The seller (after receiving the money) has to decide whether or not to send this rare first edition to the buyer If the seller ships the first edition and this edition is in correspondence with the claimed specifications, both buyer and seller