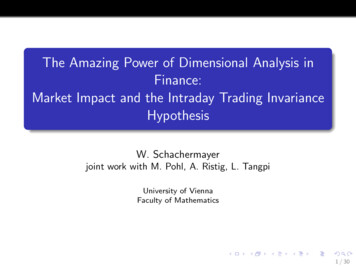
Transcription
The Amazing Power of Dimensional Analysis inFinance:Market Impact and the Intraday Trading InvarianceHypothesisW. Schachermayerjoint work with M. Pohl, A. Ristig, L. TangpiUniversity of ViennaFaculty of Mathematics1 / 30
Dimensional AnalysisThe period of the pendulumFunctional relation:gperiod f (l, m, g).2 / 30
Dimensional AnalysisThe period of the pendulumFunctional relation:gperiod f (l, m, g).Dimensions:the length l of the pendulum, measured in meters: dimension L.2 / 30
Dimensional AnalysisThe period of the pendulumFunctional relation:gperiod f (l, m, g).Dimensions:the length l of the pendulum, measured in meters: dimension L.the mass m of the bob, measured in grams: dimension M.2 / 30
Dimensional AnalysisThe period of the pendulumFunctional relation:gperiod f (l, m, g).Dimensions:the length l of the pendulum, measured in meters: dimension L.the mass m of the bob, measured in grams: dimension M.the acceleration g caused by gravity, measured in meters per secondsquared: dimension L/T2 .2 / 30
Dimensional AnalysisThe period of the pendulumFunctional relation:gperiod f (l, m, g).Dimensions:the length l of the pendulum, measured in meters: dimension L.the mass m of the bob, measured in grams: dimension M.the acceleration g caused by gravity, measured in meters per secondsquared: dimension L/T2 .Basic assumption: The three variables l, m, g fully explain the period ofthe pendulum.2 / 30
Dimensional Analysis: The period of the pendulumAnsatz:period p f (l, m, g) const · ly1 my2 g y3 .3 / 30
Dimensional Analysis: The period of the pendulumAnsatz:period p f (l, m, g) const · ly1 my2 g y3 .L lengthM massT timel100m010g10-2p0013 / 30
Dimensional Analysis: The period of the pendulumAnsatz:period p f (l, m, g) const · ly1 my2 g y3 .L lengthM massT timel100m010g10-2p001Unique solution: y1 12 , y2 0, y3 12 ,speriod const ·l.g3 / 30
Remark:The Ansatzperiod const · ly1 my2 g y3 ,does not restrict the generality of the relationperiod f (l, m, g),4 / 30
Remark:The Ansatzperiod const · ly1 my2 g y3 ,does not restrict the generality of the relationperiod f (l, m, g),or, equivalentlylog(period) F (log(l), log(m), log(g)).4 / 30
Remark:The Ansatzperiod const · ly1 my2 g y3 ,does not restrict the generality of the relationperiod f (l, m, g),or, equivalentlylog(period) F (log(l), log(m), log(g)).Indeed, the first row of the matrix translates into the requirementlog(period) F (log(l) log(x), log(m), log(g) log(x)), x 0.4 / 30
Remark:The Ansatzperiod const · ly1 my2 g y3 ,does not restrict the generality of the relationperiod f (l, m, g),or, equivalentlylog(period) F (log(l), log(m), log(g)).Indeed, the first row of the matrix translates into the requirementlog(period) F (log(l) log(x), log(m), log(g) log(x)), x 0.Hence F (·, ·, ·) must be constant along any line in R3 parallel tothe vector (1, 0, 1).4 / 30
Remark:The Ansatzperiod const · ly1 my2 g y3 ,does not restrict the generality of the relationperiod f (l, m, g),or, equivalentlylog(period) F (log(l), log(m), log(g)).Indeed, the first row of the matrix translates into the requirementlog(period) F (log(l) log(x), log(m), log(g) log(x)), x 0.Hence F (·, ·, ·) must be constant along any line in R3 parallel tothe vector (1, 0, 1).As the row vectors of the above matrix span R3 , this fullydetermines the function F (up to a constant).4 / 30
Market ImpactConsider an agent who intends to buy/sell a large amount(“meta-order” or “bet”) of some fixed stock.5 / 30
Market ImpactConsider an agent who intends to buy/sell a large amount(“meta-order” or “bet”) of some fixed stock. This bet will –ceteris paribus – move the market price to the disadvantage of theagent.5 / 30
Market ImpactConsider an agent who intends to buy/sell a large amount(“meta-order” or “bet”) of some fixed stock. This bet will –ceteris paribus – move the market price to the disadvantage of theagent. The agents are trying hard to minimize the market impact,but are unable to avoid it completely.5 / 30
Market ImpactConsider an agent who intends to buy/sell a large amount(“meta-order” or “bet”) of some fixed stock. This bet will –ceteris paribus – move the market price to the disadvantage of theagent. The agents are trying hard to minimize the market impact,but are unable to avoid it completely.DefinitionThe market impact G is the size of price change caused by a bet(in percentage of the price).5 / 30
Dimensional Analysis: The size of the market impactFunctional relation:market impact G g(Q, P, V, σ 2 ).6 / 30
Dimensional Analysis: The size of the market impactFunctional relation:market impact G g(Q, P, V, σ 2 ).Qthe size of the meta-order, measured in units of shares S,6 / 30
Dimensional Analysis: The size of the market impactFunctional relation:market impact G g(Q, P, V, σ 2 ).Qthe size of the meta-order, measured in units of shares S,Pthe price of the stock, measured in units of money per shareU/S,6 / 30
Dimensional Analysis: The size of the market impactFunctional relation:market impact G g(Q, P, V, σ 2 ).Qthe size of the meta-order, measured in units of shares S,Pthe price of the stock, measured in units of money per shareU/S,Vthe traded volume of the stock, measured in units of sharesper time S/T,6 / 30
Dimensional Analysis: The size of the market impactFunctional relation:market impact G g(Q, P, V, σ 2 ).Qthe size of the meta-order, measured in units of shares S,Pthe price of the stock, measured in units of money per shareU/S,Vthe traded volume of the stock, measured in units of sharesper time S/T,σ 2 the squared volatility of the stock, measured in percentage ofthe stock price per unit of time T 1 ,6 / 30
Dimensional Analysis: The size of the market impactFunctional relation:market impact G g(Q, P, V, σ 2 ).Qthe size of the meta-order, measured in units of shares S,Pthe price of the stock, measured in units of money per shareU/S,Vthe traded volume of the stock, measured in units of sharesper time S/T,σ 2 the squared volatility of the stock, measured in percentage ofthe stock price per unit of time T 1 ,Gthe market impact is a dimensionless quantity.6 / 30
Dimensional Analysis: The size of the market impactFunctional relation:market impact G g(Q, P, V, σ 2 ).Qthe size of the meta-order, measured in units of shares S,Pthe price of the stock, measured in units of money per shareU/S,Vthe traded volume of the stock, measured in units of sharesper time S/T,σ 2 the squared volatility of the stock, measured in percentage ofthe stock price per unit of time T 1 ,Gthe market impact is a dimensionless quantity.Basic assumption: the four variables Q, P, V, σ 2 fully explain the size ofthe market impact G.6 / 30
Dimensional Analysis: The size of the market impactAnsatz:G g(Q, P, V, σ 2 ) const · Qy1 P y2 V y3 σ 2y4 ,Q size of bet, P price of share, V traded daily volume, σ volatility.7 / 30
Dimensional Analysis: The size of the market impactAnsatz:G g(Q, P, V, σ 2 ) const · Qy1 P y2 V y3 σ 2y4 ,Q size of bet, P price of share, V traded daily volume, σ volatility.shares Smoney Utime TQ100P-110V10-1σ200-1G0007 / 30
Dimensional Analysis: The size of the market impactAnsatz:G g(Q, P, V, σ 2 ) const · Qy1 P y2 V y3 σ 2y4 ,Q size of bet, P price of share, V traded daily volume, σ volatility.shares Smoney Utime TQ100P-110V10-1σ200-1G000Leads to three linear equations in four unknowns y1 , y2 , y3 , y4 .The solution has one degree of freedomG const · Qσ 2 yV,where y R and const 0 are still free.7 / 30
This time the ansatz does restrict the generality! The generalsolution for G, respecting the dimensional restrictions isG g Qσ 2 V,where g : R 7 R is an arbitrary function.8 / 30
This time the ansatz does restrict the generality! The generalsolution for G, respecting the dimensional restrictions isG g Qσ 2 V,where g : R 7 R is an arbitrary function.Can we find one more equation which will allow us to get a uniquesolution?8 / 30
This time the ansatz does restrict the generality! The generalsolution for G, respecting the dimensional restrictions isG g Qσ 2 V,where g : R 7 R is an arbitrary function.Can we find one more equation which will allow us to get a uniquesolution?Kyle, Obizhaeva (2016): YES8 / 30
Leverage neutralityTheorem of Modigliani-Miller (1958):At assetsDt debtEt equityAssetsLiabilitiesDtAtEt9 / 30
Leverage neutralityTheorem of Modigliani-Miller (1958):At assetsDt debtEt equityAssetsLiabilitiesDtAtEtBasic assumption: (At )t 0 follows a stochastic process,e.g. Samuelson (1965):dAt (σdWt µdt).At9 / 30
Leverage neutralityTheorem of Modigliani-Miller (1958):At assetsDt debtEt equityAssetsLiabilitiesDtAtEtBasic assumption: (At )t 0 follows a stochastic process,e.g. Samuelson (1965):dAt (σdWt µdt).AtKeeping the debt Dt constant, we therefore get dAt dEt so thatdEtAt (σdWt µdt).EtEt9 / 30
Leverage neutralityTheorem of Modigliani-Miller (1958):At assetsDt debtEt equityAssetsLiabilitiesDtAtEtBasic assumption: (At )t 0 follows a stochastic process,e.g. Samuelson (1965):dAt (σdWt µdt).AtKeeping the debt Dt constant, we therefore get dAt dEt so thatdEtAt (σdWt µdt).EtEttConclusion: Denoting by Lt AEt the leverage of the company, therelative dynamics of (Et )t 0 are simply proportional to theleverage Lt .9 / 30
What happens to the stock price (Pt )t 0 , if you change theleverage? Say, the leverage L is doubled by paying out half of theequity by dividends10 / 30
What happens to the stock price (Pt )t 0 , if you change theleverage? Say, the leverage L is doubled by paying out half of theequity by dividendsP is multiplied by 21 .10 / 30
What happens to the stock price (Pt )t 0 , if you change theleverage? Say, the leverage L is doubled by paying out half of theequity by dividendsP is multiplied by 21 .σ is multiplied by 2.10 / 30
What happens to the stock price (Pt )t 0 , if you change theleverage? Say, the leverage L is doubled by paying out half of theequity by dividendsP is multiplied by 21 .σ is multiplied by 2.G is multiplied by 2.10 / 30
What happens to the stock price (Pt )t 0 , if you change theleverage? Say, the leverage L is doubled by paying out half of theequity by dividendsP is multiplied by 21 .σ is multiplied by 2.G is multiplied by 2.Q, V remain unchanged.10 / 30
Leverage neutrality: (Kyle, Obizhaeva, 2017)The value of a firm does not depend on its capital structure(Modigliani, Miller, 1958).11 / 30
Leverage neutrality: (Kyle, Obizhaeva, 2017)The value of a firm does not depend on its capital structure(Modigliani, Miller, 1958).This no-arbitrage-type condition yields one more equation involvingthe Modigliani-Miller dimension M measuring the leverage of acompany.11 / 30
Leverage neutrality: (Kyle, Obizhaeva, 2017)The value of a firm does not depend on its capital structure(Modigliani, Miller, 1958).This no-arbitrage-type condition yields one more equation involvingthe Modigliani-Miller dimension M measuring the leverage of acompany.Mathematically speaking, the variation of the leverage (dimensionM) is analogous to the scalings of the dimensions S, U, and T.11 / 30
Leverage neutrality: (Kyle, Obizhaeva, 2017)The value of a firm does not depend on its capital structure(Modigliani, Miller, 1958).This no-arbitrage-type condition yields one more equation involvingthe Modigliani-Miller dimension M measuring the leverage of acompany.Mathematically speaking, the variation of the leverage (dimensionM) is analogous to the scalings of the dimensions S, U, and T.SUTMQ1000P-110-1V10-10σ200-12G000111 / 30
Theorem (Pohl, Ristig, S., Tangpi, ’17 based on Kyle, Obizhaeva, ’16):Assume G g(Q, P, V, σ 2 ) is such thatthe variables Q, P, V , and σ 2 fully explain G,the function g is invariant under scalings of the dimensionsS, U, T,12 / 30
Theorem (Pohl, Ristig, S., Tangpi, ’17 based on Kyle, Obizhaeva, ’16):Assume G g(Q, P, V, σ 2 ) is such thatthe variables Q, P, V , and σ 2 fully explain G,the function g is invariant under scalings of the dimensionsS, U, T, and leverage neutrality holds true.12 / 30
Theorem (Pohl, Ristig, S., Tangpi, ’17 based on Kyle, Obizhaeva, ’16):Assume G g(Q, P, V, σ 2 ) is such thatthe variables Q, P, V , and σ 2 fully explain G,the function g is invariant under scalings of the dimensionsS, U, T, and leverage neutrality holds true.Then there is a const 0 such thatrG const · σQ.V12 / 30
Theorem (Pohl, Ristig, S., Tangpi, ’17 based on Kyle, Obizhaeva, ’16):Assume G g(Q, P, V, σ 2 ) is such thatthe variables Q, P, V , and σ 2 fully explain G,the function g is invariant under scalings of the dimensionsS, U, T, and leverage neutrality holds true.Then there is a const 0 such thatrG const · σQ.VIn particular, the market impact G is proportional to the squareroot of the size Q of the meta-order.12 / 30
EmpiricsDoes this relation hold true in the real world?13 / 30
EmpiricsDoes this relation hold true in the real world?Do we have to introduce more explanatory random variables(as analyzed by Kyle and Obizhaeva)?13 / 30
EmpiricsDoes this relation hold true in the real world?Do we have to introduce more explanatory random variables(as analyzed by Kyle and Obizhaeva)?Unfortunately it is hard (if not impossible) to analyze empiricallythe “true” market immpact G of an order size Q.We can hardly observe the meta-orders, however we can observethe actual orders.13 / 30
The Intraday Trading Invariance HypothesisWe now apply the method of dimensional analysis to a differentissue which has the advantage of being empirically observable:14 / 30
The Intraday Trading Invariance HypothesisWe now apply the method of dimensional analysis to a differentissue which has the advantage of being empirically observable:N : the number of trades (actual orders), measured per unit oftime[N ] T 1 .14 / 30
The Intraday Trading Invariance HypothesisWe now apply the method of dimensional analysis to a differentissue which has the advantage of being empirically observable:N : the number of trades (actual orders), measured per unit oftime[N ] T 1 .What are the variables which might explain the quantity N ?14 / 30
The Intraday Trading Invariance HypothesisWe now apply the method of dimensional analysis to a differentissue which has the advantage of being empirically observable:N : the number of trades (actual orders), measured per unit oftime[N ] T 1 .What are the variables which might explain the quantity N ?What are their dimensions?14 / 30
Following Kyle and Obizhaeva (2017) and Bouchaud et al. (2016)the following quantities come into one’s mind.V traded volume (per day),[V ] ST 1P price of a share,[P ] US 1σ 2 squared volatility,[σ 2 ] T 115 / 30
Following Kyle and Obizhaeva (2017) and Bouchaud et al. (2016)the following quantities come into one’s mind.V traded volume (per day),[V ] ST 1P price of a share,[P ] US 1σ 2 squared volatility,[σ 2 ] T 1C cost per trade,[C] U.15 / 30
First attempt of explanatory variables: P, V, σ 2Proposition:Assume that the number of trades N depends only on the 3quantities σ 2 , P and V , i.e.,N g(σ 2 , P, V ),where the function g : R3 R is dimensionally invariant.16 / 30
First attempt of explanatory variables: P, V, σ 2Proposition:Assume that the number of trades N depends only on the 3quantities σ 2 , P and V , i.e.,N g(σ 2 , P, V ),where the function g : R3 R is dimensionally invariant.Then, there is a constant c 0 such that the number of trades Nobeys the relationN c · σ2.16 / 30
First attempt of explanatory variables: P, V, σ 2Proposition:Assume that the number of trades N depends only on the 3quantities σ 2 , P and V , i.e.,N g(σ 2 , P, V ),where the function g : R3 R is dimensionally invariant.Then, there is a constant c 0 such that the number of trades Nobeys the relationN c · σ2.This relation was investigated e.g. in Jones et al. (1994).16 / 30
First attempt of explanatory variables: P, V, σ 2Proposition:Assume that the number of trades N depends only on the 3quantities σ 2 , P and V , i.e.,N g(σ 2 , P, V ),where the function g : R3 R is dimensionally invariant.Then, there is a constant c 0 such that the number of trades Nobeys the relationN c · σ2.This relation was investigated e.g. in Jones et al. (1994).Too simplistic!16 / 30
Second attempt of explanatory variables: P, V, σ 2 , CC: cost per trade17 / 30
Second attempt of explanatory variables: P, V, σ 2 , CC: cost per tradeC hQi · S average order size · bid-ask spread17 / 30
Second attempt of explanatory variables: P, V, σ 2 , CC: cost per tradeC hQi · S average order size · bid-ask spread[C] U money17 / 30
Second attempt of explanatory variables: P, V, σ 2 , CTheorem [(3/2)-law] (Benzaquen, Bouchaud, Donier, 2016):Suppose that the number of trades N depends only on the fourquantities σ 2 , P, V, C and N , i.e.,N g(σ 2 , P, V, C),18 / 30
Second attempt of explanatory variables: P, V, σ 2 , CTheorem [(3/2)-law] (Benzaquen, Bouchaud, Donier, 2016):Suppose that the number of trades N depends only on the fourquantities σ 2 , P, V, C and N , i.e.,N g(σ 2 , P, V, C),where the function g : R4 R is dimensionally invariant18 / 30
Second attempt of explanatory variables: P, V, σ 2 , CTheorem [(3/2)-law] (Benzaquen, Bouchaud, Donier, 2016):Suppose that the number of trades N depends only on the fourquantities σ 2 , P, V, C and N , i.e.,N g(σ 2 , P, V, C),where the function g : R4 R is dimensionally invariant and leverageneutral.18 / 30
Second attempt of explanatory variables: P, V, σ 2 , CTheorem [(3/2)-law] (Benzaquen, Bouchaud, Donier, 2016):Suppose that the number of trades N depends only on the fourquantities σ 2 , P, V, C and N , i.e.,N g(σ 2 , P, V, C),where the function g : R4 R is dimensionally invariant and leverageneutral.Then, there is a constant c 0 such that the number of trades N obeysthe relationσP VN 3/2 c ·.CP V σ2 C NS -1 1000U 10010T0 -1 -1 0 -1M -1 0200Table: A labelled overview of the dimensions of P, V, σ 2 , C and N .18 / 30
Empirical ResultsOur empirical analysis is based on limit order book data provided by theLOBSTER database (https://lobsterdata.com). The consideredsampling period begins on January 2, 2015 and ends on August 31, 2015,leaving 167 trading days. Among all NASDAQ stocks, d 128sufficiently liquid stocks with high market capitalizations are chosen.19 / 30
Empirical ResultsOur empirical analysis is based on limit order book data provided by theLOBSTER database (https://lobsterdata.com). The consideredsampling period begins on January 2, 2015 and ends on August 31, 2015,leaving 167 trading days. Among all NASDAQ stocks, d 128sufficiently liquid stocks with high market capitalizations are chosen.Let us fix an interval length T {30, 60, 120, 180, 360} min for which adeveloped hypothesis is tested.19 / 30
Empirical ResultsOur empirical analysis is based on limit order book data provided by theLOBSTER database (https://lobsterdata.com). The consideredsampling period begins on January 2, 2015 and ends on August 31, 2015,leaving 167 trading days. Among all NASDAQ stocks, d 128sufficiently liquid stocks with high market capitalizations are chosen.Let us fix an interval length T {30, 60, 120, 180, 360} min for which adeveloped hypothesis is tested.Nj denotes the number of trades in the interval j,PNjQj Nj 1 k 1Qtk denotes the average size of the trades in theinterval j, where Qtk denotes the number of shares traded at timetk ,Vj Nj Qj is the traded volume in the interval j,PNjPj Nj 1 k 1Ptk denotes the average midquote price in the intervalj, where Ptk (Atk Btk )/2 and Atk (resp. Btk ) denotes the bestask (resp. bid) price after the transaction at time tk ,19 / 30
Empirical Results contd.σ̂j2 denotes the estimated squared volatility in the interval j,PNjSj Nj 1 k 1Stk denotes the average bid-ask spread in theinterval j, where Stk Atk Btk is the bid-ask spread afterthe transaction at time tk , andCj Qj Sj is the spread cost per trade in the interval j.20 / 30
Statistical analysis of the hypothesesN σ2versusN σP V 2/3C.21 / 30
Statistical analysis of the hypothesesN σ2versusN General form:N (σ 2 )β σP V 2/3C. P V γC21 / 30
Statistical analysis of the hypothesesN σ2versusN General form:N (σ 2 )β σP V 2/3C. P V γCN σ 2 corresponds to β 1, γ 0,21 / 30
Statistical analysis of the hypothesesN σ2versusN General form:N (σ 2 )β σP V 2/3C. P V γCN σ 2 corresponds to β 1, γ 0, 2/3N σPCVcorresponds to β 1/3, γ 2/3,21 / 30
Statistical analysis of the hypothesesN σ2versusN General form:N (σ 2 )β σP V 2/3C. P V γCN σ 2 corresponds to β 1, γ 0, 2/3N σPCVcorresponds to β 1/3, γ 2/3,Linear constraint: β γ 1.21 / 30
Statistical analysis of the hypothesesN σ2versusN General form:N (σ 2 )β σP V 2/3C. P V γCN σ 2 corresponds to β 1, γ 0, 2/3N σPCVcorresponds to β 1/3, γ 2/3,Linear constraint: β γ 1.Multiplicative model: γi 2 βi Pij VijNij σ̂ijexp( ij ),Cij21 / 30
Statistical analysis of the hypothesesN σ2N versusGeneral form:N (σ 2 )β σP V 2/3C. P V γCN σ 2 corresponds to β 1, γ 0, 2/3N σPCVcorresponds to β 1/3, γ 2/3,Linear constraint: β γ 1.Multiplicative model: γi 2 βi Pij VijNij σ̂ijexp( ij ),CijLinear model:2 ) γ loglog(Nij ) βi log(σ̂iji Pij VijCij ij .21 / 30
T 60minT 120min0.50.7 γi0.91.1Density23100011Density23Density23444T 30min0.50.7 γi0.91.10.7 γi0.91.1T 360min0011Density23Density2344T 180min0.50.50.7 γi0.91.10.50.7 γi0.91.1Figure: The panels show kernel density estimates across the estimatedparameters γ̂i for different interval lengths T {30, 60, 120, 180, 360}min.22 / 30
129.0 8.06.5 11 10 9 log(σ2) 8 7.0log(N) 8.5 slope 0.670R2 0.9406.08.58.07.5log(N)7.06.5 6.0AAL 7.59.0AALslope 0.410R2 0.352 8 910111213 PV C)log(σFigure: The dependent variable log N is plotted versus the explanatoryvariables log σ̂ resp. log(σ̂P V /C) for fixed interval T 60 min and theAAL stock. The lines indicate the estimated linear relations between theconsidered quantities.23 / 30
AAPL 9.0log(N) 1011 log(σ2) 8.0 7.09.08.58.07.57.0 912 slope 0.640R2 0.978 8 9.5 10.0 8.5 7.59.5 10.0 log(N)AAPL slope 0.470R2 0.34013 1011121314 PV C)log(σFigure: The dependent variable log N is plotted versus the explanatoryvariables log σ̂ resp. log(σ̂P V /C) for fixed interval T 60 min and theAAPL stock. The lines indicate the estimated linear relations betweenthe considered quantities.24 / 30
An Afterthought: the dimension of volatilityDefinition of volatility per time T :σ 2 Var(log(Pt T ) log(Pt )).25 / 30
An Afterthought: the dimension of volatilityDefinition of volatility per time T :σ 2 Var(log(Pt T ) log(Pt )).Example of price process (Black-Scholes):dPu Pu (σdWu µdu).25 / 30
An Afterthought: the dimension of volatilityDefinition of volatility per time T :σ 2 Var(log(Pt T ) log(Pt )).Example of price process (Black-Scholes):dPu Pu (σdWu µdu).Obvious consequence: [σ 2 ] T 1 .25 / 30
An Afterthought: the dimension of volatilityDefinition of volatility per time T :σ 2 Var(log(Pt T ) log(Pt )).Example of price process (Black-Scholes):dPu Pu (σdWu µdu).Obvious consequence: [σ 2 ] T 1 .But what we really plug into a formula likeN 3/2 c ·σP VCis an estimate σ̂ 2 of the “true” volatility σ 2 (whatever this is).25 / 30
An Afterthought: the dimension of volatilityDefinition of volatility per time T :σ 2 Var(log(Pt T ) log(Pt )).Example of price process (Black-Scholes):dPu Pu (σdWu µdu).Obvious consequence: [σ 2 ] T 1 .But what we really plug into a formula likeN 3/2 c ·σP VCis an estimate σ̂ 2 of the “true” volatility σ 2 (whatever this is).What does the empirical data tell us on this issue?25 / 30
Typical estimator for σ 2 :σ̂ 2 : n Xlog(Ptk ) log(Ptk 1 ) 2.k 1where t t0 t1 . . . tn t T are the points in [t, t T ]where Pt jumps.26 / 30
Typical estimator for σ 2 :σ̂ 2 : n Xlog(Ptk ) log(Ptk 1 ) 2.k 1where t t0 t1 . . . tn t T are the points in [t, t T ]where Pt jumps.But we could also consider, for H ]0, 1[,σ̂ 2 (H) : N Xlog(Ptk ) log(Ptk 1 )1/H 2Hk 1Possible reasons for H 6 12 :26 / 30
Typical estimator for σ 2 :σ̂ 2 : n Xlog(Ptk ) log(Ptk 1 ) 2.k 1where t t0 t1 . . . tn t T are the points in [t, t T ]where Pt jumps.But we could also consider, for H ]0, 1[,σ̂ 2 (H) : N Xlog(Ptk ) log(Ptk 1 )1/H 2Hk 1Possible reasons for H 6 12 :fractional Brownian motion W H instead of W (Mandelbrot,1961,. . . )26 / 30
Typical estimator for σ 2 :σ̂ 2 : n Xlog(Ptk ) log(Ptk 1 ) 2.k 1where t t0 t1 . . . tn t T are the points in [t, t T ]where Pt jumps.But we could also consider, for H ]0, 1[,σ̂ 2 (H) : N Xlog(Ptk ) log(Ptk 1 )1/H 2Hk 1Possible reasons for H 6 12 :fractional Brownian motion W H instead of W (Mandelbrot,1961,. . . )rough volatility (Bayer, Gatheral, Rosenbaum, . . . )26 / 30
Typical estimator for σ 2 :σ̂ 2 : n Xlog(Ptk ) log(Ptk 1 ) 2.k 1where t t0 t1 . . . tn t T are the points in [t, t T ]where Pt jumps.But we could also consider, for H ]0, 1[,σ̂ 2 (H) : N Xlog(Ptk ) log(Ptk 1 )1/H 2Hk 1Possible reasons for H 6 12 :fractional Brownian motion W H instead of W (Mandelbrot,196
P the price of the stock, measured in units of money per share U S, V the traded volume of the stock, measured in units of shares per time S T, 2 the squared volatility of the stock, measured in percentage of t