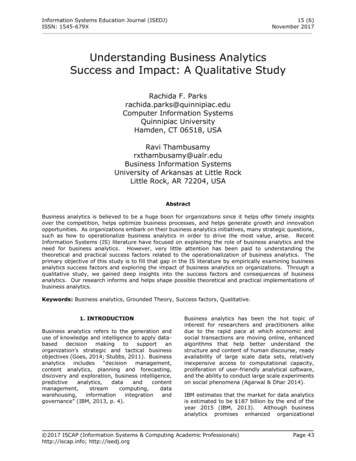
Transcription
Information Systems Education Journal (ISEDJ)ISSN: 1545-679X15 (6)November 2017Understanding Business AnalyticsSuccess and Impact: A Qualitative StudyRachida F. Parksrachida.parks@quinnipiac.eduComputer Information SystemsQuinnipiac UniversityHamden, CT 06518, USARavi Thambusamyrxthambusamy@ualr.eduBusiness Information SystemsUniversity of Arkansas at Little RockLittle Rock, AR 72204, USAAbstractBusiness analytics is believed to be a huge boon for organizations since it helps offer timely insightsover the competition, helps optimize business processes, and helps generate growth and innovationopportunities. As organizations embark on their business analytics initiatives, many strategic questions,such as how to operationalize business analytics in order to drive the most value, arise. RecentInformation Systems (IS) literature have focused on explaining the role of business analytics and theneed for business analytics. However, very little attention has been paid to understanding thetheoretical and practical success factors related to the operationalization of business analytics. Theprimary objective of this study is to fill that gap in the IS literature by empirically examining businessanalytics success factors and exploring the impact of business analytics on organizations. Through aqualitative study, we gained deep insights into the success factors and consequences of businessanalytics. Our research informs and helps shape possible theoretical and practical implementations ofbusiness analytics.Keywords: Business analytics, Grounded Theory, Success factors, Qualitative.1. INTRODUCTIONBusiness analytics refers to the generation anduse of knowledge and intelligence to apply databaseddecisionmakingtosupportanorganization’s strategic and tactical businessobjectives (Goes, 2014; Stubbs, 2011). tent analytics, planning and forecasting,discovery and exploration, business nintegrationandgovernance” (IBM, 2013, p. 4).Business analytics has been the hot topic ofinterest for researchers and practitioners alikedue to the rapid pace at which economic andsocial transactions are moving online, enhancedalgorithms that help better understand thestructure and content of human discourse, readyavailability of large scale data sets, relativelyinexpensive access to computational capacity,proliferation of user-friendly analytical software,and the ability to conduct large scale experimentson social phenomena (Agarwal & Dhar 2014).IBM estimates that the market for data analyticsis estimated to be 187 billion by the end of theyear 2015 (IBM, 2013).Although businessanalytics promises enhanced organizational 2017 ISCAP (Information Systems & Computing Academic Professionals)http://iscap.info; http://isedj.orgPage 43
Information Systems Education Journal (ISEDJ)ISSN: 1545-679X15 (6)November 2017performance and profitability, improved decisionmaking processes, better alignment of resourcesand strategies, increased speed of decisionmaking, enhanced competitive advantage, andreduced risks (Computerworld, 2009; Goodnight,2015; Harvard Business Review Analytics Report,2012), implementation success is far fromassured. A survey of 3,000 executives conductedby MIT Sloan Management Review along with IBMInstitute of Business Value (LaValle, Lesser,Shockley, Hopkins, & Kruschwitz, 2011) revealedthat the leading obstacle to widespread analyticsadoption is “lack of understanding of how to useanalytics to improve the business”. Gartner’s2014 annual big data survey shows that whileinvestment in big data technologies continues toincrease, “the hype is wearing thin as businessintelligence and information management leadersface challenges when tackling diverse objectiveswith a variety of data sources and technologies”(Gartner, 2014a). Several studies (Ariyachandra& Watson, 2006; Eckerson, 2005; Imhoff, 2004;Popovič et al., 2012; Yeoh & Koronios, 2010)have focused on the critical success factorsrelated to business analytics implementation,while several others (Computerworld, 2009;Goodnight, 2015; Harvard Business ReviewAnalytics Report, 2012) have covered theconsequences of business analytics. However,there is a lack of a unified model of businessanalytics success factors and business analyticsimpact.The research questions for this study are asfollows: What are the determinants of businessanalytics success? What impact does businessanalytics have on organizations that plan toimplement it? How can these success factors andimpact dimensions be integrated into a unifiedmodel of business analytics value? Our studyaddresses these research questions by applying agrounded theory approach to 17 qualitativeinterviews conducted with 18 senior executivesfrom 15 business analytics organizations in 7industries.The structure of this paper is as follows: The nextsection briefly reviews the most importantbusiness analytics conceptualizations and studiesthat informed our research. We then outline ourmethodological approach for answering theresearch questions. Subsequently, we presentour findings and synthesize them into a unifiedmodel of business analytics success and impact.We conclude the paper with a discussion of ourcontributions to theory development and practice,limitations of our study, and strategic implicationsof our findings.2. LITERATURE REVIEWBusiness AnalyticsIS researchers are familiar with the data information knowledge continuum. Pearlson &Saunders (2013) define data as “a set of specific,objective facts or observations” (p. 14). They addthat information is data that has been “endowedwith relevance and purpose” (Pearlson &Saunders, 2013, p. 15). Knowledge is thendefined as “information that is synthesized andcontextualized to provide value” (Pearlson &Saunders, 2013, p. 16).Business analytics refers to the application ofrelevant measurable knowledge to strategic andtactical business objectives through data-baseddecision making (Stubbs, 2011). Goes (2014)adds that analytics refers to the higher stages inthe data–knowledge continuum and is directlyrelated to decision support systems, a wellestablished area of IS research.Businessanalytics is “the generation of knowledge andintelligence to support decision making andstrategic objectives” (Goes, 2014, p. vi).Business analytics represents the analyticalcomponent in business intelligence (Davenport,2006).Chen et al., (2012) traced the evolution ofbusiness analytics and categorized businessintelligence and analytics (BI&A) into BI&A 1.0(DBMS-based, structured content), BI&A 2.0(web-based, unstructured content), and BI&A 3.0(mobile and sensor based, unstructured content).Chen et al. (2012) add that in addition to beingdata-driven, business analytics is highly applied,with the potential to revolutionize areas such ase-commerce and market intelligence, egovernment and politics, science and technology,smart health and well-being, and security andpublic safety.Most of the research on business analytics till datehave focused on its application in marketing(Chau & Xu, 2012; Lau et al., 2012; Park et al.,2012; Sahoo et al., 2012) and financial services(Abbasi et al., 2012; Hu et al., 2012). Chau & Xu(2012) proposed a framework for gatheringbusiness intelligence from user-generated blogs(BI&A 2.0) using content analysis on the blogsand social network analysis of the bloggers’interaction networks to help increase sales andcustomer satisfaction in a marketing context. Lauet al., (2012) developed a novel due diligencebalanced scorecard model that uses collectiveweb intelligence (BI&A 2.0) techniques such asdomain-specific sentiment analysis, businessrelation mining, and statistical learning to 2017 ISCAP (Information Systems & Computing Academic Professionals)http://iscap.info; http://isedj.orgPage 44
Information Systems Education Journal (ISEDJ)ISSN: 1545-679X15 (6)November 2017enhance decision making related to globalmergers and acquisitions. Park et al. (2012)proposed a social network-based (BI&A 2.0)relational inference model which incorporatedtechniques such as social network analysis, userprofiling, and query processing to determine thevalidity of self-reported customer profiles whichform the basis of many organizational externaldata acquisition efforts to boost their businessanalytics outcomes.Sahoo et al., (2012)proposed a hidden Markov model that usestechniques such as statistical modeling andcollaborative filtering (BI&A 1.0) to makepersonalized recommendations under conditionsof changing user preferences. Abbasi et al.,(2012) developed a meta-learning model thatutilizes techniques such as adaptive learning, andclassification and generalization (BI&A 1.0) togenerate a confidence score associated with eachof its predictions to help detect fraud in thefinancial services industry. Hu et al., (2012) usea network approach to risk management (NARM)which includes predictive modeling, niques (BI&A 1.0) to identify systemic risk inbanking systems.Determinants of Business Analytics SuccessPopovič et al. (2012) developed a model ofbusiness intelligence systems (BIS) success thatincluded the business intelligence dimensions ofBIS maturity, information content quality,information access quality, analytical decisionmaking culture, and use of information fordecision-making. BIS maturity refers to the stateof the development of BIS within theorganization. Information content quality, in theBIS context, refers to information relevance oroutput quality. Information access quality refersto the bandwidth, customization capabilities, andinteractivity offered by the BIS.Analyticaldecision-making culture refers to the attitudetowards the use of information in decision-makingprocesses.Use of information for decisionmaking refers to the application of acquired making (Leonard-Barton & Deschamps,1988).Popovič et al. (2012) tested their model on datacollected from 181 organizations and found thatBIS maturity has a strong impact on informationaccess quality. Their results also showed thatinformation content quality, and not informationaccess quality, was relevant for the use ofinformation for decision-making, and thatanalytical decision-making culture improved theuse of information for decision-making whilesuppressing the direct impact of informationcontent quality.Ariyachandra & Watson (2006) analyzed thecritical success factors for BI implementation andfound that information quality, system quality,individual impacts, and organizational impactsare the four factors which determine whether anorganization’s BI efforts are successful. Theirinformation quality dimension included subfactorssuchasinformationaccuracy,completeness of information, and consistency ofinformation (Ariyachandra & Watson, 2006). Thesystem quality dimension included sub-factorssuch as BI system flexibility, scalability, andintegration (Ariyachandra & Watson, 2006).Individual impacts included quick access to data,ease of data access, and improved decisionmaking capabilities while organizational impactsinclude BI use, accomplishment of ments, improved ROI, and enhancedcommunication and collaboration across businessunits (Ariyachandra & Watson, 2006).Yeoh & Koronios (2010) classified businessanalytics success determinants into , process related success factors, andtechnology-related success eterminants such as a clear organizationalvision, and a well-established business case(Yeoh & Koronios, 2010). Their process-relatedsuccess factors included determinants such asbalanced team composition, well-establishedproject management methodologies, and useroriented change management procedures (Yeoh& Koronios, 2010).Their technology-relatedsuccess factors included determinants such as ascalable and flexible architecture, and sustainabledata quality and data integrity (Yeoh & Koronios,2010).Eckerson (2005) identified critical success factorsfor enterprise business intelligence (BI). Thosecritical success factors included support for allusers via integrated BI suites, conformity of BItools to the way users work rather than the otherway around, ability of the BI tools to integratewith desktop and operational applications, abilityof the BI tools to deliver actionable information,ability of the analytics team to rapidly developtools and reports to meet fast changing userrequirement, and an underlying BI platform thatis robust and extensible (Eckerson, 2005).Imhoff (2004) identified five success factors thatare critically important to any business wishing to 2017 ISCAP (Information Systems & Computing Academic Professionals)http://iscap.info; http://isedj.orgPage 45
Information Systems Education Journal (ISEDJ)ISSN: 1545-679X15 (6)November 2017develop a BI environment. Those success factorsincluded a dependable architecture, strongpartnership between the business community andIT, an agile/prototyping methodology, welldefined business problems, and a willingness toaccept change (Imhoff, 2004).Howson (2008) identified four critical successfactors while exploring the characteristics of akiller BI app. Those BI success determinantsincluded culture, people’s views of the value ofinformation, exploratory and predictive models,and fact-based management (Howson, 2008).Consequences of Business AnalyticsSuccessJim Goodnight, CEO of SAS Institute Inc., statesthat business analytics has a tremendous impacton organizational performance and profitabilityadding that the “ability to predict future businesstrends with reasonable accuracy will be one of thecrucial competitive advantages of this newdecade. And you won’t be able to do that withoutanalytics.” (Goodnight, 2015, p.3).A Computerworld survey (Computerworld, 2009)
Business analytics is believed to be a huge boon for organizations since it helps offer timely insights over the competition, helps optimize business processes, and helps generate growth and innovation opportunities. As organizations embark on their business analytics initiatives, many strategic questions,