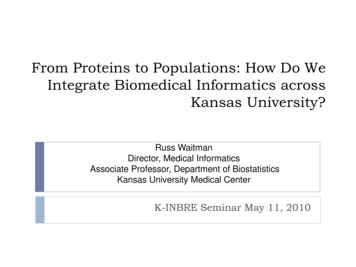
Transcription
From Proteins to Populations: How Do WeIntegrate Biomedical Informatics acrossKansas University?Russ WaitmanDirector, Medical InformaticsAssociate Professor, Department of BiostatisticsKansas University Medical CenterK-INBRE Seminar May 11, 2010
Disclaimer Warning: this talk draws extensively from the workof esteemed informaticians and should not be seenas the novel thought of the presenter. Any proposals are based on a preliminary threemonth assessment and are designed to promotediscussion. The presenter does not have any conflicts of interestregarding the information presented.
PreliminaryGameSelectionPopulationSurveyTrade Study:Mammoth vs.Tiger vs. RabbitFinal ementsSite PreparationSite SelectionGameSightingsPreliminaryHunting PlanPreliminaryHunterSelectionFinal HuntingPlanHunt LeaderChosenWeaponSelectionTrade Study:Club vs. SpearWeaponsPractice and inaryHuntingReview(PHR)WeaponDevelopmentObtain Blessingof Great GodThagFinal dinessReview(HRR)Site SurveyExamineEntrailsHunterInspectionTransport toCaveCatch GameGet CaughtBy GameChase GameLose GameDistributeMeatKILL GameChoose NewHunt LeaderI don't know.It seemedeasier when we justwent hunting.Yes, but Ogassures me thatthis will improveour efficiency andkeep us ahead ofthoseCro-Magnons inthe valley.Why the Neanderthals Became Extinct
Outline Perspectives on biomedical informaticsNIH objectives regarding translational research?Strawman for KU and filling medical informatics gapsDiscussion: What is the vision for bioinformatics in Kansas?What are the strongest stories and linkages we can tell orrelationships we can build across campuses?What projects should we pursue to make contributions toinformatics as a discipline versus providing clinicaltranslational research support?
Background: Charles Friedman The Fundamental Theorem of BiomedicalInformatics: A person working with an information resource is betterthan that same person unassisted. NOT!!Charles P. Friedman: http://www.jamia.org/cgi/reprint/16/2/169.pdf
Background: Randolph Miller ON THE NEED FOR DECISION SUPPORT:1. Life is short, the art long, opportunity fleeting, experiencetreacherous,judgment difficult. Hippocrates. Aphorisms, 460-400 BC ALSO ON THE NEED FOR DECISION SUPPORT:2. Men are men; the best sometimes forget. Shakespeare. Othello,1604-5 ON THE NEED TO EVALUATE DECISION SUPPORT SYSTEMS:(also interpreted as avoidance of medical informatics vaporware)3. The proof of the pudding is in the eating.Miguel de Cervantes. Don Quixote, 1605
Background: William SteadThe Individual ExpertClinicianSynthesis &DecisionEvidencePatientRecordWilliam Stead: http://courses.mbl.edu/mi/2009/presentations fall/SteadV1.ppt
The demise of expert-basedpractice is inevitableFacts per Decision1000Proteomics and othereffector molecules100Functional Genetics:Gene expressionprofiles10Structural Genetics:e.g. SNPs, haplotypesHumanCognitive 5CapacityDecisions by ClinicalPhenotype1990200020102020William Stead: http://courses.mbl.edu/mi/2009/presentations fall/SteadV1.ppt
Background: Edward ShortliffeBasic ResearchMethods, Techniques, and TheoriesPublic HealthApplied entistryVisualizationMolecular BiologyEdward Shortliffe: ence presentations/shortliffe.ppt
Background: Edward ShortliffeBasic ResearchApplied ResearchBiomedical Informatics Methods,Techniques, and TheoriesBioinformaticsImagingClinicalInformatics InformaticsMolecular andTissues lic HealthInformaticsPopulationsAnd SocietyEdward Shortliffe: ence presentations/shortliffe.ppt
Background: Edward ShortliffeBiomedical Informatics Research AreasBiomedicalKnowledgeMachine learningText interpretationKnowledge evelopmentBiomedicalDataBiomedicalResearchPlanning &Data AnalysisInferencingSystemDataAcquisitionReal-time acquisitionImagingSpeech/language/textSpecialized input ward Shortliffe: ence presentations/shortliffe.ppt
Background: Dan MasysNIH Goal to Reduce Barriers to Research Administrative bottlenecks Poor integration of translational resources Delay in the completion of clinical studies Difficulties in human subject recruitment Little investment in methodologic research Insufficient bi-directional information flow Increasingly complex resources needed Inadequate models of human disease Reduced financial margins Difficulty recruiting, training, mentoring scientists
Clinical and Translational Science AwardsA NIH Roadmap Initiative“It is the responsibility of those of usinvolved in today’s biomedical researchenterprise to translate the remarkablescientific innovations we are witnessinginto health gains for the nation.”
CTSA Objectives:The purpose of this initiative is to assist institutions toforge a uniquely transformative, novel, andintegrative academic home for Clinical andTranslational Science that has the consolidatedresources to:1) captivate, advance, and nurture a cadre of welltrained multi- and inter-disciplinary investigators andresearch teams;2) create an incubator for innovative research toolsand information technologies; and3) synergize multi-disciplinary and inter-disciplinaryclinical and translational research and researchers tocatalyze the application of new knowledge andtechniques to clinical practice at the front lines ofpatient care.
NIH CTSAs: Home for Clinical andTranslational ticsClinicalResourcesBiostatisticsTrial rticipant& InstitutionsDan Masys: http://courses.mbl.edu/mi/2009/presentations fall/masys.ppt
Reengineering Clinical ResearchInterdisciplinaryResearchInnovator Award Public-Private PartnershipsBenchBuilding Blocksand PathwaysMolecular ceIntegrated Research NetworksClinical Research InformaticsNIH Clinical Research AssociatesClinical outcomesHarmonizationTrainingDan Masys: http://courses.mbl.edu/mi/2009/presentations fall/masys.ppt
Role of Informaticsin Clinical and Translational Research Structured observation and record keeping are theessence of scienceInformatics Centric Efforts: Clinical Trial enrollmentClinical Trial softwareReuse, integration, and sharing of electronic health datato support translational researchBioinformatics and Biospecimen managementMethods Applicable to other large infrastructureneeds: NCI Cancer Center designation.CTSA Informatics cross institutional goals?
IKFC Ops Committee; Dec, 2008CTSA ConsortiumSteering Committee (CCSC)Informatics Key Function Committee and Operations subcommitteeIKFC Prioritized Projects,Special Interest Groups (SIGS),ProjectsCTSA PI Priorities National Clinical andTranslational Research Capability Clinical Research Management Research Infrastructure Phenotyping-human and pre-clinicalmodelsCTSA Strategic GoalCommitteesCTSA PI Liaisons Training & Career Developmentof Clinical/Translational Scientists Enhancing Consortium-WideCollaborations Members Social Networking Inventory of Resources Data sharing Enhancing the Health of OurCommunities and the Nation Community Engagement Public Health Policy Human Study DatabaseProject group (Sim and Team) Data Repositories (Kamerick) Standards & Interoperability (Chute) Education (Klee, Hersh)Other Key FunctionCommitteesIKFCPrioritizationProcess Collaboration Facilitation (Kahlon) Resource Inventory (Becich, Athey &Team) Data Sharing (Silverstein, Anderson) Nat’l Human Subject VolunteerRegistry (Harris)
Building a Vision: EnvironmentalComparison VU/KU VUMC: unified leadership across hospitals, clinics,academics Unified informatics: from network jack and server, tolibrary and bioinformatics coresBuild/buy mix legacy - complexityLarge consolidated academic home for informaticsData sharing for research a non issueKU/KUMC, Rest of the world: not so homogenous What can one do with EPIC or Cerner addedinformatics?Validated solutions more likely to scale.Data sharing involves multiple organizations
KU Opportunities Quality Focused Hospital CTSA goal: Data “Warehouse” Without every solution involving informaticsAdvance research and clinical quality“Green Field” for newer technologiesState and Region KUMC strong in community outreach research Link our data to external information? (Ex: KHPA Medicaid data)Health Information Exchange “window”KU Lawrence Informatics, StowersLong term: Cerner
Medical Informatics: Short Term Approach Data sharing aggrement and data access This is not a one size fits all solutionDevelop terms of agreement and oversightUnderstand current information strategy andtimelines of our partner organizationsEngage research communityEstablish development environmentGain experience with KUH/KUPI/KUMC informationsystems Focus on practical pilot projectsIdeally, benefits to clinical quality and research
Data Sharing roles: entities with justifiable(and variable) rights to medical data First order role definitions: Provider, Patient, Payer, “Society”Second order: Providers: primary vs. consultant provider, ancillarysupport staffPatient: self, family, legally authorized repsPayer: billing staff and subcontractors, clearinghouses,insurersSociety: public health agencies, state medical boards, lawenforcement agenciesDan Masys: http://crypto.stanford.edu/portia/workshops/2004 7 slides/masys.ppt
Data Sharing roles: entities with justifiable(and variable) rights to medical data Third order: Providers: internal and external QA entities (peer review,JCAHO), sponsors of clinical researchPatient: community support groups, personal friendsPayers: fraud detection (Medical Information Bureau),business consultantsSociety: national security, bioterrorism detectionDan Masys: http://crypto.stanford.edu/portia/workshops/2004 7 slides/masys.ppt
Healthcare InformationAccess RolesCommunitySupportInternal lySpecialistsAuthorized yerFraud ProcessorsDetection tionBureauBusinessConsultantsSocietyPublic HealthStateLicensureLawEnforcementNationalBoards SecurityBioterrorismDetectionDan Masys: http://crypto.stanford.edu/portia/workshops/2004 7 slides/masys.ppt
Intermediate CTSA aligned goals Build team, evaluate, and choose appropriateinformatics products and underlying technologiesImplement incremental construction of “warehouse” information strategy Balance retrospective with near real time opportunitiesClinical data foundation, then link to other resourcesand provide research opportunity Potential linkages with biospecimensState data for epidemiology researchNDNQI nursing quality indicators
Strawman: Recall Shortliffe ModelBasic ResearchMethods, Techniques, and TheoriesPublic HealthApplied entistryVisualizationMolecular BiologyEdward Shortliffe: ence presentations/shortliffe.ppt
KU CTSA and Overall StrawmanAcademicHomes KU-LCenter forBioinformaticsAcademicHomes KUMCITTC EECSBioinformaticsBioInformatics(K-INBRE coord)Center for HealthInformaticsMedicalInformaticsUKP: clinicsCores/LabsAcademicStructureDept. HealthInfo Mgt (BS)OperationalService LayerHealthInformaticsPublic: SSDIKUH: hospitalBiospecimenRepositoriesCRIS: trialsPublic: GenBankMolecular andCellularProcessesState/KHPA:Medicaid State: ExchangesExtension CentersTissues andOrgansIndividuals(Patients)PopulationsAnd SocietyOrganizations/Data sources
Medical and Health Informatics Vision By directly engaging in clinical and health informaticsdatabases, we in turn learn about the delivery ofcare and the effectiveness of informatics methods asmechanisms for influencing care.If we can develop strong relationships with ourprovider, state, and clinical research organizations,we will provide a rich environment for clinical andinformatics research The hospital and clinic data is our core resourceEngagement in state wide data in complementary to ourexisting research strength in preventative medicine
Share with me your “Vision” What is the vision for bioinformatics in Kansas? What are the strongest stories and linkages we cantell or relationships we should build acrosscampuses? What projects might we pursue to makecontributions to informatics as a discipline versusproviding clinical translational and other researchsupport?
The presenter does not have any conflicts of interest regarding the information presented. Population Game Survey . Game Sightings Meat Requirements Review (MRR) . captivate, advance, and nurture a cadre of wel