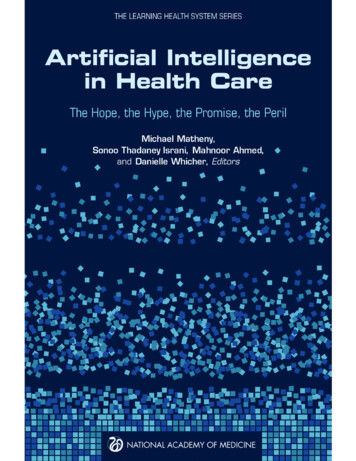
Transcription
Artificial Intelligence in Health Care:The Hope, the Hype,the Promise, the PerilMichael Matheny, Sonoo Thadaney Israni, Mahnoor Ahmed,and Danielle Whicher, EditorsWASHINGTON, DCNAM.EDUPREPUBLICATION COPY - Uncorrected Proofs
NATIONAL ACADEMY OF MEDICINE 500 Fifth Street, NW WASHINGTON, DC 20001NOTICE: This publication has undergone peer review according to proceduresestablished by the National Academy of Medicine (NAM). Publication by the NAMsignifies that it is the product of a carefully considered process and is a contributionworthy of public attention, but does not constitute endorsement of conclusions andrecommendations by the NAM. The views presented in this publication are those ofindividual contributors and do not represent formal consensus positions of the authors’organizations; the NAM; or the National Academies of Sciences, Engineering, andMedicine.Library of Congress Cataloging-in-Publication Data to ComeCopyright 2019 by the National Academy of Sciences. All rights reserved.Printed in the United States of America.Suggested citation: Matheny, M., S. Thadaney Israni, M. Ahmed, and D. Whicher, Editors.2019. Artificial Intelligence in Health Care: The Hope, the Hype, the Promise, the Peril.NAM Special Publication. Washington, DC: National Academy of Medicine.PREPUBLICATION COPY - Uncorrected Proofs
“Knowing is not enough; we must apply.Willing is not enough; we must do.”--GOETHEPREPUBLICATION COPY - Uncorrected Proofs
ABOUT THE NATIONAL ACADEMY OF MEDICINEThe National Academy of Medicine is one of three Academies constituting the National Academies of Sciences, Engineering, and Medicine (the National Academies). The National Academies provide independent, objective analysis and advice to the nation andconduct other activities to solve complex problems and inform public policy decisions.The National Academies also encourage education and research, recognize outstandingcontributions to knowledge, and increase public understanding in matters of science,engineering, and medicine.The National Academy of Sciences was established in 1863 by an Act of Congress,signed by President Lincoln, as a private, nongovernmental institution to advise the nation on issues related to science and technology. Members are elected by their peers foroutstanding contributions to research. Dr. Marcia McNutt is president.The National Academy of Engineering was established in 1964 under the charter ofthe National Academy of Sciences to bring the practices of engineering to advising thenation. Members are elected by their peers for extraordinary contributions to engineering. Dr. John L. Anderson is president.The National Academy of Medicine (formerly the Institute of Medicine) was established in 1970 under the charter of the National Academy of Sciences to advise the nation on issues of health, health care, and biomedical science and technology. Membersare elected by their peers for distinguished contributions to medicine and health. Dr.Victor J. Dzau is president.Learn more about the National Academy of Medicine at NAM.edu.PREPUBLICATION COPY - Uncorrected Proofs
AUTHORSMICHAEL MATHENY (Co-Chair), Vanderbilt University Medical Center and theDepartment of Veterans AffairsSONOO THADANEY ISRANI (Co-Chair), Stanford UniversityANDREW AUERBACH, University of California, San FranciscoANDREW BEAM, Harvard UniversityPAUL BLEICHER, OptumLabsWENDY CHAPMAN, University of MelbourneJONATHAN CHEN, Stanford UniversityGUILHERME DEL FIOL, University of UtahHOSSEIN ESTIRI, Harvard Medical SchoolJAMES FACKLER, Johns Hopkins School of MedicineSTEPHAN FIHN, University of WashingtonANNA GOLDENBERG, University of TorontoSETH HAIN, EpicJAIMEE HEFFNER, Fred Hutchinson Cancer Research CenterEDMUND JACKSON, Hospital Corporation of AmericaJEFFREY KLANN, Harvard Medical School and Massachusetts General HospitalRITA KUKAFKA, Columbia UniversityHONGFANG LIU, Mayo ClinicDOUGLAS MCNAIR, Bill & Melinda Gates FoundationENEIDA MENDONÇA, Regenstrief InstituteJONI PIERCE, University of UtahW. NICHOLSON PRICE II, University of MichiganJOACHIM ROSKI, Booz Allen HamiltonSUCHI SARIA, Johns Hopkins UniversityNIGAM SHAH, Stanford UniversityRANAK TRIVEDI, Stanford UniversityJENNA WIENS, University of MichiganixPREPUBLICATION COPY - Uncorrected Proofs
NAM StaffDevelopment of this publication was facilitated by contributions of the followingNAM staff, under the guidance of J. Michael McGinnis, Leonard D. Schaeffer ExecutiveOfficer and Executive Director of the Leadership Consortium for a Value & Science-DrivenHealth System:DANIELLE WHICHER, Senior Program Officer (until September 2019)MAHNOOR AHMED, Associate Program OfficerJESSICA BROWN, Executive Assistant to the Executive Officer(until September 2019)FASIKA GEBRU, Senior Program AssistantJENNA OGILVIE, Deputy Director of CommunicationsxPREPUBLICATION COPY - Uncorrected Proofs
REVIEWERSThis special publication was reviewed in draft form by individuals chosen for theirdiverse perspectives and technical expertise, in accordance with review proceduresestablished by the National Academy of Medicine. We wish to thank the followingindividuals for their contributions:SANJAY BASU, Harvard Medical SchoolPAT BAIRD, PhilipsSARA BURROUS, Washington University, St. LouisKEVIN JOHNSON, Vanderbilt University Medical CenterSALLY OKUN, PatientsLikeMeJ. MARC OVERHAGE, Cerner CorporationJACK RESNECK, JR., American Medical AssociationSARA ROSENBAUM, George Washington UniversityPAUL TANG, IBM Watson HealthTIMOTHY M. PERSONS, Chief Scientist and Managing Director, Science, TechnologyAssessment, and Analytics, United States Government Accountability Office(NOTE: Dr. Persons only provided editorial comments and technical advice on thedescription of the artificial intelligence technology described in the publication. Dr.Persons did not comment on any policy related recommendations and did not reviewor comment on any of the legal content in the publication.)The reviewers listed above provided many constructive comments and suggestions,but they were not asked to endorse the content of the publication, and did not see thefinal draft before it was published. Review of this publication was overseen by DanielleWhicher, Senior Program Officer, NAM; Mahnoor Ahmed, Associate Program Officer,and J. Michael McGinnis, Leonard D. Schaeffer Executive Officer, NAM. Responsibility forthe final content of this publication rests with the editors and the NAM.xiiiPREPUBLICATION COPY - Uncorrected Proofs
FOREWORDIn 2006, the National Academy of Medicine established the Roundtable on Evidence-Based Medicinefor the purpose of providing a trusted venue for national leaders in health and health care to work cooperatively toward their common commitment to effective, innovative care that consistently generatesvalue for patients and society. The goal of advancing a “Learning Health System” quickly emerged andwas defined as “a system in which science, informatics, incentives, and culture are aligned for continuous improvement and innovation, with best practices seamlessly embedded in the delivery process andnew knowledge captured as an integral by-product of the delivery experience”1.To advance this goal, and in recognition of the increasingly essential role that digital health innovations in data and analytics contribute to achieving this goal, the Digital Health Learning Collaborativewas established. Over the life of the collaborative, the extraordinary preventive and clinical medicalcare implications of rapid innovations in artificial intelligence (AI) and machine learning emerged asessential considerations for the consortium. The publication you are now reading responds to the needfor physicians, nurses and other clinicians, data scientists, health care administrators, public health officials, policy makers, regulators, purchasers of health care services, and patients to understand the basicconcepts, current state of the art, and future implications of the revolution in AI and machine learning.We believe that this publication will be relevant to those seeking practical, relevant, understandableand useful information about key definitions, concepts, applicability, pitfalls, rate-limiting steps, andfuture trends in this increasingly important area.Michael Matheny, MD, MS, MPH and Sonoo Thadaney Israni, MBA have assembled a stellar team ofcontributors, all of whom enjoy wide respect in their fields. Together, in this well-edited volume that hasbenefitted from the thorough review process ingrained in the National Academy of Medicine’s culture,they present expert, understandable, comprehensive, and practical insights on topic areas that includethe historical development of the field; lessons learned from other industries; how massive amounts ofdata from a variety of sources can be appropriately analyzed and integrated into clinical care; how innovations can be used to facilitate population health models and social determinants of health interventions; the opportunities to equitably and inclusively advance precision medicine; the applicability forhealth care organizations and businesses to reduce the cost of care delivery; opportunities to /LearningHealthSystem 28jul15.pdfxvPREPUBLICATION COPY - Uncorrected Proofs
xviFOREWORDinteractions between health care professionals and patients, families, and caregivers; and the role oflegal statutes that inform the uptake of AI in health care.As the co-chairs of the Digital Health Learning Collaborative, we are excited by the progress being demonstrated in realizing a virtuous cycle in which the data inevitably produced by every patient encountermight be captured into a “collective memory” of health services to be used to inform and improve thesubsequent care of the individual patient and the health system more generally. Enormous datasets areincreasingly generated, not only in the formal health care setting, but also emanating from data streamsfrom medical and consumer devices, wearables, patient-reported outcomes, as well as environmental,community and public health sources. They include structured (or mathematically operable) data aswell as text, images and sounds. The landscape also includes data “mash-ups” from commercial, legal,and online social records.AI has been the tool envisioned to offer the most promise in harvesting knowledge from that collective memory, and as this volume demonstrates, some of that promise is being realized. Among the mostimportant of these promises in the near term is the opportunity to assuage the frustration of health careproviders who have been clicking away on electronic records with modest benefit beyond increaseddata transportability and legibility. Our hope is that AI will be the “payback” for the investment in boththe implementation of electronic health records and the cumbersomeness of their use by facilitatingtasks that every clinician, patient, and family would want, but are impossible without electronic assistance—such as monitoring a patient for emergent sepsis 24 7 365 and providing timelier therapyfor a condition in which diagnostic delay correlates with increased risk of death.However, we also appreciate that AI alone cannot cure health care’s ills and that new technologiesbring novel and potentially under-appreciated challenges. For example, if a machine learning algorithmis trained with data containing a systematic bias, then that bias may be interpreted as normative, exacerbating rather than resolving disparities and inequities in care. Similarly, association of data doesnot prove causality, and it may not even be explanatory, suggesting that a simultaneous revolution inresearch methods is also necessary. Finally, the mere existence of substantial and sensitive data assetsraises concerns about privacy and security. Aspiring to the promise of AI requires both continuing innovation and attention to the potential perils.In our opinion, this publication presents a sober and balanced celebration of accomplishments, possibilities, and pitfalls. We commend Drs. Michael McGinnis and Danielle Whicher for their thoughtfulsponsorship of the NAM Consortium and Digital Health Learning Collaborative, Dr. Matheny and Mrs.Thadaney Israni for their leadership in producing this volume, and to all the contributors who haveproduced an exceptional resource with practical relevance to a wide array of key stakeholders.Jonathan B. Perlin, MD, PhD, MACPReed V. Tuckson, MD, FACPCo-Chairs, Digital Learning Collaborative,Consortium on Value and Science-Driven Health Care,National Academy of MedicinePREPUBLICATION COPY - Uncorrected Proofs
CONTENTSACRONYMS AND ABBREVIATIONSxixFIGURES, BOXES, AND TABLESxxiiiSUMMARY11ARTIFICIAL INTELLIGENCE IN HEALTH CARE:THE HOPE, THE HYPE, THE PROMISE, THE PERIL2OVERVIEW OF CURRENT ARTIFICIAL INTELLIGENCE3HOW ARTIFICIAL INTELLIGENCE IS CHANGING HEALTHAND HEALTH CARE4POTENTIAL TRADE-OFFS AND UNINTENDED CONSEQUENCESOF AI5AI MODEL DEVELOPMENT AND VALIDATION6DEPLOYING AI IN CLINICAL SETTINGS7HEALTH CARE AI: LAW, REGULATION, AND POLICY8ARTIFICIAL INTELLIGENCE IN HEALTH CARE:HOPE NOT HYPE, PROMISE NOT PERILAPPENDICESAADDITIONAL KEY REFERENCE MATERIALSBWORKSHOP AGENDA AND PARTICIPANT LISTCAUTHOR BIOGRAPHIESPREPUBLICATION COPY - Uncorrected Proofs7355989119145181214229231237
Acronyms and AbbreviationsACMAssociation of Computing MachineryAIArtificial IntelligenceAMA American Medical AssociationAPI application programming interfaceATM automated teller machine(s)AUROCarea under the ROC curveBBC British Broadcasting CorporationCDCCenters for Disease Control and PreventionCDM common data modelCDS clinical decision supportCGMPCurrent Good Manufacturing ProcessCLIAClinical Laboratory Improvement AmendmentsCMSCenters for Medicare & Medicaid ServicesCONSORTConsolidated Standards of Reporting TrialsCPICClinical Pharmacogenetics Implementation ConsortiumCPU central processing unit(s)DARPADefense Advanced Research Projects AgencyDHHSDepartment of Health and Human ServicesDHLCDigital Health Learning CollaborativeDOJ Department of JusticeECA embodied conversational agentsECG electrocardiogramEFF Electronic Frontier FoundationEHR electronic health record(s)EU European Unionxix
xxACRONYMS AND ABBREVIATIONSFAIRfindability, accessibility, interoperability, and reusabilityFDAFood and Drug AdministrationFDCAFederal Food, Drug, and Cosmetic ActFHIRFast Healthcare Interoperability ResourcefRamilyfriends and family unpaid caregiversFTC Federal Trade CommissionFTCAFederal Trade Commission ActGDPRGeneral Data Protection RegulationGPS global positioning systemGPU graphics processing unit(s)GWAS genome-wide association studiesHAZOPhazard and operability studyHIE health information exchangeHIPAAHealth Insurance Portability and Accountability ActHITECH ActHealth Information Technology for Economic and Clinical Health ActHIVhuman immunodeficiency virusi2b2Informatics for Integrating Biology & the BedsideICD-9International Classification of Diseases, 9th RevisionICD-10International Classification of Diseases, 10th RevisionIEEEInstitute of Electrical and Electronics EngineersIOM Institute of MedicineIoT Internet of ThingsIMDRFInternational Medical Device Regulators ForumIP intellectual propertyIT information technologyIVDin vitro diagnostic deviceIVDMIAin vitro diagnostic multivariate index assay(s)JITAI just-in-time adaptive interventionsLeadership ConsortiumThe National Academy of Medicine Leadership Consortium:Collaboration for a Value & Science-Driven Learning Health SystemLDT laboratory-developed test(s)LHS learning health systemLOINCLogical Observational Identifiers Names and CodesMITMassachusetts Institute of Technology
ACRONYMS AND ABBREVIATIONSxxiNAMNational Academy of MedicineNASNational Academy of SciencesNeurIPSConference on Neural Information Processing SystemsNHTSANational Highway Traffic Safety AdministrationNIHNational Institutes of HealthNITRCNeuroimaging Informatics Tools and Resources ClearinghouseNLP natural language processingNNHnumber needed to harmNNTnumber needed to treatNPV negative predictive valueNRC National Research CouncilNSTCNational Science and Technology CouncilOHDSIOHRPOMOPONCObservational Health Data Sciences and InformaticsOffice for Human Research ProtectionsObservational Medical Outcomes PartnershipThe Office of the National Coordinator for Health Information TechnologyPARiHSPromoting Action on Research Implementation in Health ServicesPCORnetPatient-Centered Clinical Research NetworkPDSA plan-do-study-actPFS physician fee schedulePHI protected health informationPPV positive predictive valuePR precision-recallPre-CertDigital Health Software Precertification ProgramQI quality improvementQMS quality management systemR&D research and developmentROC receiver operating characteristicRWD real-world dataRWE real-world evidence
xxiiACRONYMS AND ABBREVIATIONSSaMDsoftware as a medical deviceSDoHsocial determinants of healthSDLC software development life-cycleSMARTSubstitutable Medical Apps, Reusable TechnologySTARDStandards for Reporting of Diagnostic Accuracy StudiesTPR true positive rateTPU tensor processing unit(s)WEIRDWestern, educated, industrialized, rich, and democraticUDN Undiagnosed Diseases Network
FiguresFigures, Boxes, and TablesS-1S-2Advancing to the Quintuple Aim, 2Appropriately regulating AI technologies will require balancing a number of importantvariables, including intellectual property (IP), concerns around privacy and consent, risksand liability associated with the use of the technologies, and developmental processes, 54-14-24-34-4Gartner Hype Cycle, 90Construction of an “adversarial example”, 98The Future of Employment, 100Projected Total Physician Shortfall Range, 2015-2030, 1011-11-21-31-41-51-65-15-26-16-26-37-18-1Life expectancy gains and increased health spending, selected high-incomecountries, 1995-2015, 8A summary of the domains of artificial intelligence, 13A summary of the most common methods and applications for training machinelearning algorithms, 14Growth in facts affecting provider decisions versus human cognitive capacity, 17Framework for implementing AI through the lens of human rights values, 22Chapter relationship, 26What is a model?, 129Patient timeline and associated data gathering opportunities, 130Determinants of population health, 149Relationship of population health to public health and standard clinical care, 150Developmental life cycle of artificial intelligence applications, 163Different forms of transparency, 192Recommended data standardization framework to promote AI system developmentfrom high-quality, transparent, and interoperable data, 216xxiii
xxivFIGURES, BOXES, AND TABLESBoxes5-15-2Key Considerations in Model Development, 122Key Definitions in Model Development, 1288-28-38-4The Quintuple Aim to ensure equity & inclusion are stated and measured goalswhen designing and deploying health care interventions, 219Summary of relationships between requirements for transparency and the three axesof patient risk, user trust, and algorithm performance within three key domains:data transparency, algorithmic transparency, and product/output transparency, 221Relationship between regulation and risk, 2257-1Federal Food, Drug, and Cosmetic Act (21 U.S.C. § 360j) Medical Device Definition, 185Tables1-11-23-13-2Practical challenges to the advancement and application of AI tools in clinical settingsidentified during the November 2017 Digital Health Learning Collaborative Meeting, 11Relevant Ethical Codes, Frameworks, and Guidelines, 21Examples of AI applications for stakeholder groups, 60Illustrative examples of AI solutions to aid in health care administration processes, 735-1Example of artificial intelligence applications by the primary task and mainstakeholder, 1216-16-26-37-1Leveraging AI tools int
essential considerations for the consortium. The publication you are now reading responds to the need for physicians, nurses and other clinicians, data scientists, health care administrators, public health offi- . MPH and Sonoo Thadaney Israni, MBA have assembled a stellar team of contributors, all of whom enjoy wide respect in their fields .