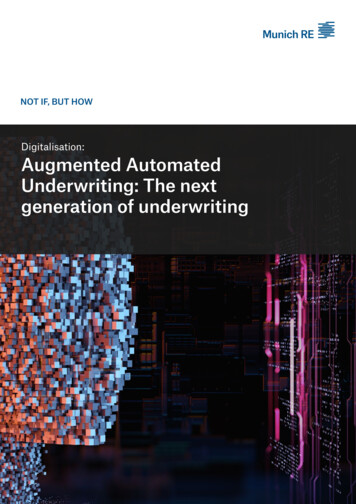
Transcription
Digitalisation:Augmented AutomatedUnderwriting: The nextgeneration of underwriting7Augmenting Reality: The new generation of automated underwritingIntroduction
IntroductionIn many ways, life insurance is likeany other business. Success is largelydependent on efficient processes,satisfied customers and sustainableprofit margins. However, there areimportant caveats: the customerexperience is inherently problematicand the on-boarding process iscomplex and can involve multipleindependent parties, making itinefficient by design. Moreover, thedesire for decent margins co-existswith sensitivity around the societalimpacts of profit-at-all-cost and the‘social good’ of this kind of insurance.These challenges have existed for aslong as life insurance itself. Degreesof automation have been introducedover time to incrementally addresssome of these issues, but thefundamentals have not changedsignificantly – until now.Currently, the life insurance industryexists in a world where customers’expectations have been transformedby technology in both their personaland professional lives. Convenienceand speed are now of supreme valueand place traditional life insurancepractices in an unflattering spotlight.Attracting, engaging and retainingcustomers through the entire onboarding process is becoming evermore challenging, putting marginsat risk.Artificial Intelligence (AI), which willbe discussed in greater depth in thenext paper in this series, can often beused as an all-encompassing termfor advanced analytics or to suggesta more advanced technique than isactually being used.In addition, different regional historiesand regulatory priorities havediversified language: what we callaugmented automated underwriting(AAU) may also be described asaugmented underwriting with AI,accelerated underwriting, or predictiveunderwriting, among other terms.For the sake of simplicity, we use AAUin this paper, in which we examine: How AAU builds on and evolvesprevious technology investmentsand implementations How advanced analytics, predictivemodelling, and machine learningchange underwriting processes andtransform the customer experience Whether AAU is the inevitabledestination for life insuranceunderwritingNew technological capabilitiesallow us to directly confront thesechallenges. However, before we furtherexplore this topic, there are questionsof terminology to address.1Augmented Automated Underwriting: The next generation of underwriting
Part I:Digital customers,digital technology2Augmented Automated Underwriting: The next generation of underwriting
A newcustomercohortAAU is all about revolutionising thecustomer experience for the better. Ageneration of consumers, well-versedin researching, buying and reviewingproducts online, has entered themarket for life insurance – but theyare facing a buying experience thatdoes not align to the expectationsof ‘digital natives’. As a result, ourglobal customer base faces a broadlyconsistent set of challenges, despiteregional differences.This generation includesmillennials, who are oftendismissed as experience-driven,spendthrift consumers who areactively harming their own financialwell-being. This stereotype is neitherhelpful nor accurate. The oldestmembers of this net-native cohortare fast-approaching 40, and havethe same financial concerns andcore needs as earlier customers.Millennials are already acquiring andaccessing bank accounts, payments,foreign exchange, mortgages andother insurance products throughmobile apps, aggregators and pricecomparison sites. The contrast withthe inconvenience, time and typeof questions being asked for lifeinsurance could not be more stark.Those questions and tests requiredetails that people rarely share withanyone outside their closest family.Equally important, traditional salestechniques are losing their appeal,which is deeply problematic forwhat is traditionally a ‘sold’ product.Millennial customers, who are oftenmore focused on near-term, tangibleneeds, are less likely to see brokers oragents that do not offer a digital andspeedy process as an essential partof the sales process. This is a fairlysubstantial shift from GenerationXers – those born between themid-1960s and late 1970s – whoremain comfortable with moretraditional approaches and slowturn-around times.This shift is taking place against abackdrop of increasing pressure topush down the cost of onboardingcustomers as part of broader efficiencymeasures. Our own experience tellsus that this is often coupled with ashortage of underwriting experience incertain regions, which places furtherconstraints upon the business.Finally, overlooking all of this areregulators and ombudsmen whoare increasingly taking a punitiveview of poor decision-making, riskmanagement and asymmetricinformation between the consumerand the insurer.Even those who have no intention offalling out of the process are oftenleft by the wayside, particularly whendoctors’ appointments, medical tests,blood-work and requests for furtherdetails frustrate the process. Evidencefrom aggregator sites shows us thatspeed to decision is key: prospectivebuyers are 50 per cent more likely topurchase the offers that appear mostquickly. Traditional life insurancecomes with too many barriers to entry,and too many decelerating factors.3Augmented Automated Underwriting: The next generation of underwriting
A new customercohortIn order to enhance customer experience, the challengesfacing the life insurance industry are broadly recognised.However, so are the desired improvements. The industry’scommon goals include:1. Greater efficiency Enabling firms to usefewer resources to deliverappropriate outcomes2. Greater effectiveness Allowing resources to be allocatedwhere they are most effective andcan reach ‘better’ outcomes faster3. Greater consistency Producing consistent outcomesand reducing monitoring throughrepeatable processes4. Greater transparency Simplifying the decision processto produce a clear path to outcomesand ensuring actions are auditable5. Greater knowledge To unlock better insights andimprove opportunities foriterating successAs the next evolutionary stage in the history of technologyand underwriting, AAU can deliver on all of these goals.4Augmented Automated Underwriting: The next generation of underwriting
Fromautomated toaugmentedTechnology has rightly been seenas a major part of the solutionto the challenges outlined abovefor some time. The emergentclass of ‘insurtech’ is the latestiteration of what has beena fairly lengthy evolution towardsgreater digitisation, as humanjudgement has been replacedby a more mathematical andscientific approach.This digital evolution can bebroken down into five distinctivephases, of which the first threewill be familiar to most in the lifeinsurance business.First Generation:Black-box systemsInsurers digitised their systemsand implemented jet-casing orclear-casing systems for online andback-office modes – the predecessorsof the underwriting rules engine.These systems were often developedin house and intended to introducesome automated capabilities to theunderwriting process. Typically, these‘engines’ would allow applicationforms that had no disclosures to beexpedited through the system andchecked for some basic businessvalidation rules. By speeding up theonboarding process, they provided adegree of efficiency. But they did not‘show their workings’, which meantthey gave no insight into how best toimprove the underlying underwritingphilosophy; and because theywere mainly hard-coded rules, theyoffered only limited ability to adjustboundaries or parameters.Second Generation:Electronic underwritingThe next iteration of systems gaveunderwriters the opportunity to reviewand amend their own underwritingphilosophy. These systems allowedbehavioural economics and externaldata to be introduced, but in mostcases, they still required IT andprogramming expertise. Changerequests had to be sent to IT and/orthe reinsurer, leading to the build-up oflengthy queues. This became a strongconstraining factor and did little to aidcompetitive differentiation.5Augmented Automated Underwriting: The next generation of underwriting
From automatedto augmentedThird Generation:Descriptive analyticsThis stage of system evolutionoffered descriptive analytic power viainsights from dashboards and reports,better visualisation techniques andgraphical user interfaces (GUIs).These improvements gave greaterflexibility and power to individualunderwriters, placing them in thedriving seat and enabling them tomanage and implement new businessprocesses and change rules withoutIT or programming support. On thecompliance front, reinsurers withconduct post-hoc auditing, eitheron-site or remotely, ultimately puttingdecision-making power in the handsof underwriters.The majority of life insurancebusinesses use second or thirdgeneration systems. However, theevolution is continuing. The fourthstage is where insurers are nowable to obtain deeper insights fromtheir data, using advanced analyticstechniques, and developing predictivemodels that can further improve thecustomer experience, increase salesand enhance business performance.Digital Roadmap from rules engines to AI/augmented underwritingOutcomesPaper DigitalDigitiseInsurer DigitalEvolution(1) STP rate (60% ) TATs SalesSTP initiation(2)Integrated analyticsDeep insightsDescriptive (3)AnalyticsAuto-UWE-ApplicationPOS underwritingBack officeunderwritingChange UWphilosophyPredictive (4)AnalyticsDashboards for allstakeholdersOptimise rulesIntegrate internal /external dataOptimise processperformanceTransformed Cxoptimised operations(5)ModelDeploymentAnalyse historical dataIntegrate into POSDevelop predictivemodelsModel decisions,augment UW decisionsQuantify the riskE.g. Streamline UW,reduce questions,optimise STPCustomerSatisfactionURERules DesignerTechnologyeAPPConsultingConsulting: POS underwriting philosophy, rules development & ongoing optimisation6Analytics EngineCloudData ExtractionAI ModellingModel Integration,Deployment, Monitoring,MaintenanceConsulting: Predictive model development, risk review& quantification, AI explanation, exec sign-offRI risk transfer: On business through AI modelsAugmented Automated Underwriting: The next generation of underwritingClaims
The FourthGeneration:PredictiveanalyticsFourth generation solutionsintroduce advanced analytics.This is a giant leap forward. All riskbased decisions hinge on makingconnections between various datapoints and identifying patterns withinthem; however, these patterns aren’talways immediately discernibleby humans. Advanced analyticscan reveal these previously hiddenconnections and relationships with anexceptional degree of accuracy.By using AI and machine learningtechniques, insurers can, for example,identify which data-points in theapplication process are irrelevantor simply redundant, and whichapplications are now suitablefor immediate straight-throughprocessing (STP). The system canassess risk profiles and, in certaincircumstances and with appropriaterisk management, the number ofquestions asked can be significantlyreduced with little to no impact on risktaken on by the life insurer. Certainparts of the application process maysafely be eliminated altogether.To illustrate the impact that analyticscan have, consider the case of apotential customer aged 25-35,with an early form of diabetes. It’san unusual condition, but commonenough that there is plenty of dataabout other individuals with the samecondition in the same age group.That data shows this group is still alow-risk category with an average lifeexpectancy.The data also shows that this categoryof customer is usually approved forstandard life cover once all personalevidence has been reviewed. In otherwords, insurers require intrusivetests, ask multiple questions, and addroadblocks to the process when thecustomer will be accepted anyway.Diabetes is an obvious flag for lifeinsurers, and the request for medicaltests and evidence feels correct.Human judgements have been builtinto the system. However, whatappears to be a common-sensedecision is not necessarily the correctone. With AI and machine learningtechniques, insurers gain a deeperunderstanding of these kind ofcounter-intuitive realities as well asthe effects – past and present – of theirchosen approach.“ Use predictive modelsto eliminate questions,reduce variables, changerules and secure anoptimal result.”7Augmented Automated Underwriting: The next generation of underwriting
The FifthGeneration:AAUbecomesbusinessas-usualMany insurers have embarked onadvanced analytics initiatives, but facechallenges in effectively deploying themodels developed. Fifth-generationsystems leverage advanced analyticalmodelling by fully integrating predictivemodels into existing underwriting andnew-business processes. This is whatwe mean by augmented automatedunderwriting (AAU).To enable underwriters and datascientists to quickly and effectivelydeploy, integrate, monitor and evolvemodels over time, such that modelmanagement becomes business asusual, is a major challenge.Technology that can predict anapplicant’s risk with an acceptablelevel of accuracy significantly improvesthe consumer experience and reducesonboarding barriers. AAU therefore hasthe potential to draw more people intothe life insurance application process,and then keep them in that process forlonger, to the benefit of customersand insurers alike.Importantly, the adoption of this newtechnology does not require a ‘rip andreplace’ approach. Instead, insurerscan choose their preferred models,use existing rules-developmentcapability to define interactionswith those models, and incorporatethose models by reconfiguring theirexisting underwriting processes. Thefinal underwriting decision for lifeinsurance applications may thereforeuse a model as an input to support andaugment the rules-engine decision.Models can be selected and deployedto achieve one of a number of goals,including but not limited to: Streamlining theunderwriting process Transforming the consumerexperience Reducing manual underwriting andmedical evidence costsThe degree to which the modelresponse is used to determine thefinal underwriting decision will, ofcourse, vary by insurer, use case, dataavailability and model confidence,as well as geographic locationand regulation.For example, one insurance firm inNorth America is in the process oftransitioning to predictive modellingrather than opting for an overnightswitch. Its use of predictive modelsallows individual underwriters tocompare their decision-makingwith the model, and highlightwhere human and model are insync to build confidence in themodel itself as it increasinglyinfluences decision-making.On the other hand, two life insurersin Southeast Asia transitioned fullyto predictive underwriting withmodels and technology developedby Munich Re. Improving turn-around times Increasing STP rates Decreasing the number ofquestions asked Identifying fraudulent behaviour Implementing up- and crossselling campaigns8Augmented Automated Underwriting: The next generation of underwriting
The FifthGeneration:AAU becomesbusinessas-usualThis generation is not just aboutmodel development, however.Creating a product from the modelhas knock-on implications, including:understanding and quantifying therisk it may imply; quantifying anypricing implications; consideringthe explainability of the model; andobtaining executive sign-off before itgoes into production.It also requires that underwriters,operations staff and technologistswork closely with actuaries, datascientists and data engineers in thedevelopment and sign-off of the modeland its intended deployment. In manyinstances, insurers will want to sharethe risk of the model as it is eventuallydeployed, because it is such adeparture from traditional approaches.By deploying AAU, insurance firmshave already achieved significantimprovements to their STP ratesand costs, but with only negligibleincreases to their own risk. Someexamples from Munich Re’sexperience: Company A reduced the amountof medical evidence required ofits potential customers, shortenedits questionnaire significantly,and decreased the amount ofmanual processing undertaken byits underwriters, which increasedits STP rate from 30% to 70% Company B pursues a stringentunderwriting philosophy tosafeguard profits but stillincreased its STP rate from 35%to 60%, decreasing its manualunderwriting requirements andthe associated costs, as wellas minimising the amount ofmedical evidence demanded ofapplicants Company C increased its STPrate from an already impressive60% to 80%, again decreasingits manual underwritingrequirements and the associatedcosts, as well as minimising theamount of medical evidencedemanded of applicants Despite eliminating 80% of itsprevious underwriting questions,Company D was able to offerstandard rates to 50% of itsapplicants with only a minimalprice increase“ Model selection andseamless integration forstreamlined applicationsand accurate offers.”9Augmented Automated Underwriting: The next generation of underwriting
Part II:Questionsand answers10Augmented Automated Underwriting: The next generation of underwriting
Regions andregulationsThe fifth generation can thereforebe seen as the next stage in theevolution of insurance technology,streamlining the customer experiencewhile maintaining or even enhancingthe life insurer’s confidence in its riskevaluation processes.While all paths may lead to AAU, thejourneys are not identical. So far, wehave discussed AAU as a generalconcept. However, there are regionaldifferences which will determine howunderwriters will effectively transitionto AAU.If we consider regulatory jurisdictionsas a spectrum, the European model isat one end, while the general US model– allowing for variations within the 50states – is at the other.In general, the US market is fact-basedand relies on extensive proof to validatethe information that is provided. Thiscreates a complex, parallel and multistage underwriting process, whichrequires detailed questionnaires andmultiple data sources and leads tocomplex outcomes: preferred plus;preferred; standard; rated; declined;or postponed.11Automation is therefore also complex,with plenty of manual processes stillrequired. For example, the demandfrom regulators in New York andCalifornia that underwriting rules beregistered, places serious constraintson an underwriter’s ability to updatethose rules in response to changingdata patterns. In addition, thecontestability period of 24 monthsmeans the burden of proof remains onthe underwriter when decisions arechallenged. The primary goal for USunderwriters, therefore, is to accelerateand simplify this process.In contrast, most countries in Europeapply the principle of utmost goodfaith. Customers provide informationto insurers, who take it on trust whenassessing risk. The process is typicallysimpler and more linear: interview,decision, additional evidence, andfinal decision. The complexity of theunderwriting questionnaire dependson a number of factors, includingproduct, distribution channel,engagement model, pricing, andmarketing practice, but commonlyaccepted outcomes are standard;rated; declined; or postponed.The level of automation alreadyin place also depends on a numberof factors, ranging from marketmaturity and availability of structureddata, to the nature of the underwritingprocess itself.Augmented Automated Underwriting: The next generation of underwriting
Regions andregulationsIn Europe, the burden of proof liesmore with the customer than theunderwriter; as a result, contestabilityis usually shorter and more swiftlyaddressed. The goal here is to usedata models in a way that improvesunderwriting accuracy, minimises falsepositives and reduces ‘spikes’ in risk.The APAC region is different again.Japan and other countries in SouthEast Asia have similarly high levels ofautomation as their peers in Europe,while the level of automation inAustralia is still comparatively low.The journeys to greater automationand augmented automation withinthe region will therefore take slightlydifferent routes.Meanwhile, there are plenty ofcountries that do not have readilyavailable, coherent records.However, fifth-generationtechnology is effectively agnostic.It can incorporate an underwriter’sfundamental philosophy, bring inmodels that support that, and pulldata in from other sources if necessary.As a neutral data hub, it allows fordisparate data sources and formatsto be incorporated, from which AAUcan be achieved.The nature of medical records in aparticular region also has an impact onthe adoption of AAU. As an example,UK health files are digitised throughoutthe National Health Service (NHS). Thechallenge is that family doctors havetraditionally acted as a gatekeeperof those records and were paid byreinsurers to provide them. As a result,there is some resistance to change,which can slow progress.12Augmented Automated Underwriting: The next generation of underwriting
ExplainabilityandtransparencyDespite regional differences, thereare two universally recognisedrequirements for AAU: explainabilityand transparency. These key factorsoften differentiate life insurance fromother financial products.AAU places underwriting in the realmof complex models that leveragemachine-learning techniques.Insurers will therefore be increasinglyrequired to use expert judgementwhen selecting models for use inAAU, since they are the means bywhich they achieve their fundamentalunderwriting philosophy. However,insurers will still need to prove toregulators and ombudsmen that thechosen approach is sound and thedecision-making is valid. This raisestwo key questions:1. Philosophically, how does humanintelligence understand anddescribe even the earliest formsof artificial intelligence?2. Practically, how does an underwriterexplain in a court of law thata machine algorithm made thecorrect decision?13Transparency and explainability haveto be built into both the model andthe model-selection process. GUIs,easily configurable rules, transparency,reporting, and now advanced analyticsare not unique to life insurance, andwe have seen similar moves towardsgreater automation and machinelearning in areas like fraud preventionand detection. Yet the life sectorhas rejected some of the automateddecision-making seen in otherareas precisely because of its‘explainability’ requirements.Explainability is therefore one of thekey barriers to adoption. However, itis worth noting that the demand forexplainability has not always been metby first-, second- or third-generationtechnologies. Explaining the neteffect of thousands of rules is noeasy feat, and regulators have oftenexpressed interest in avoiding unfairdiscrimination against customers by‘black-box’ models.Fortunately, many of the latestmachine-learning models havebecome more ‘interpretable’ thanearlier iterations. As a result, it ispossible to explain decision-makingat an aggregate level and to drilldown into what drives a singleprediction for a specific customer.The gap between an explainablemodel and explaining the impactof 1,000 variables may not be asinsurmountable as many believe.Augmented Automated Underwriting: The next generation of underwriting
Models andcomponentsAs indicated above, achievingAAU is not about implementing asingle, monolithic system. Multiplecomponents, or modules, areinvolved. Claims engines, machinelearning algorithms, monitoringdashboards, underwriting rulesets, data integration, and naturallanguage processing (NLP) all formpart of the picture, as does a scalablearchitecture enabled by cloudcapabilities and APIs.Individually, each of these componentparts offers very little value. It is onlywhen they are integrated and overlaidwith in-depth knowledge of the lifeinsurance sector – its history, itsregulations, its requirements and itsbroader societal role – that they canbe transformed into sources ofbusiness value such as point-of-salepredictive models.The process of selecting the modelsthemselves must also be considered.Companies have to take into accountthe trade-offs between modeleffectiveness and performance,complexity and explainability, and findthe right balance for their organisationin its market and regulatory context.All model developers – whetherinternal or external – must buildtransparency into their own processes,from data accessibility and dataanalysis, to model integration,deployment and monitoring.Developers should also strive to avoidthe syntax and jargon of data science,so that statistical methodology canbe adequately communicated tonon-experts.Currently, independent companiesand AI consultancies are developingmodels for insurance and otherpurposes. Some are based on publiclyavailable data, while others are basedon insurer-specific data. However,models that are built using only publicdata generally cover only one pieceof the puzzle that is a human life tobe insured.As a result, models from within theinsurance business offer an advantage.Many insurers therefore may chooseto work with models developed byproviders with insurance, underwriting,data and actuarial experts who havethe ability to explain the implicationsto risk, compliance and actuarialcolleagues and get the modelssigned off.This is a big leap to make for therisk-averse insurance sector andrepresents a profound change in theway insurance works. That change willbe required at almost every level.Capital, modelling andtechnology expertiseAs one of the world’s largestreinsurers, Munich Re takes a risksharing approach when developingmodels for clients with the capitalto support it. It has business unitsthat are dedicated to developingmodels, which can be integrated intoour underwriting engine alongsidemodels from third parties. However,when Munich Re models are used,tested and validated, and all partiesare convinced that the model willmake the right decisions, it isprepared to take a significant portionof the risk through a reinsurancearrangement. This ‘skin in thegame’ is a significant advantagereinsurers have in the development of14Augmented Automated Underwriting: The next generation of underwritingmodels, unlike, for example, pure AIconsultancies. It requires reinsurersto provide utmost diligence in themodel development as any modeldeployment has a significant financialimpact if its risks implications arenot properly assessed by actuarial,claims, underwriting and data scienceexperts.
Managingthe datadelugeAlthough there are challengesin moving to AAU, there are alsopowerful reasons for overcomingthose challenges. There is also asense of inevitability surroundingits adoption. The onward march oftechnological capability, coupled withthe rapid evolution of data science,has had a significant impact on theinsurance industry as a whole, andis likely to do the same for the lifeinsurance sector. The choice to adoptAAU may, in the end, not be much of achoice at all.For example, new sources ofpertinent health data are becomingreadily available. Electronic healthrecords (EHRs), for example, canprovide structured information thatcould be readily integrated intomodel development, all withoutinconveniencing customers orphysicians. In addition, today’swearable fitness trackers are compilingcomplementary data sets that mayprove valuable to underwriters. Theinternal data that insurance companiesalready hold can also be integratedinto models and mined for previouslyhidden insights.These additional sources ofinformation raise questions about whatdata could be used in the future. Whenconsidering potential data sources, wemust ask: Will the regulators allow it? Will it truly add value?Of course, the answers are notalways easy to come by. Considerthe following: The technology behind facialrecognition, although not sufficientlyaccurate at present, could eventuallybe used to identify a smoker who isreluctant to admit their habit. Is thisadmissible? Genetic data, increasingly availableas DIY DNA and ancestry kits growin popularity, could provide helpfulrisk information. No regulatorcurrently allows them to be usedextensively, and they are unlikely todo so in the near future. But couldregulators at some point overcomethis reluctance? Personal lifestyle informationabout hobbies, education, diet andother factors is readily availablethrough social media accounts. Thisinformation could be incorporatedinto models, but will it proveproblematic when it comes tojustifying decision-making? On arelated note, should models minesocial media as a whole for usefultrend analysis and broader societalcontext, or should this too beinadmissible?These examples provide insight intosome of the questions that will beasked in the near future. Similarly, theasymmetry of information betweenthe applicant and the insurer will haveto be addressed by regulators and theinsurance industry. Will it undermine principles of eitherconsent or explainability?15Augmented Automated Underwriting: The next generation of underwriting
Data scienceand itstechnologyIn addition to the ethical questionsaround data use, there is the morepractical issue of whether data isreadily usable. Structured formatsare needed for model analysis anddetails tend to be gathered in formalprocesses, which necessarily excludesa vast amount of information. Theprocess of gathering structureddata is also prone to distortion, ascustomers interpret and filter theirown personal information to suit theirself-perception.Data science is also in the process offinding better ways to convert moreinformal or analogue informationinto structured digital formats. NLPis one method that converts thecomplexity of normal conversationinto more standardised, readableand analysis-friendly data. Equally,the insight gained from data analysiscan itself help to structure questionsmore effectively and to pre-populateforms in a way that provides the mostuseful information. Analytics can alsoidentify where data could and shoul
augmented automated underwriting (AAU) may also be described as augmented underwriting with AI, accelerated underwriting, or predictive underwriting, among other terms. For the sake of simplicity, we use AAU in this paper, in which we examine: How AAU builds on and evolves previous technology investments and implementations