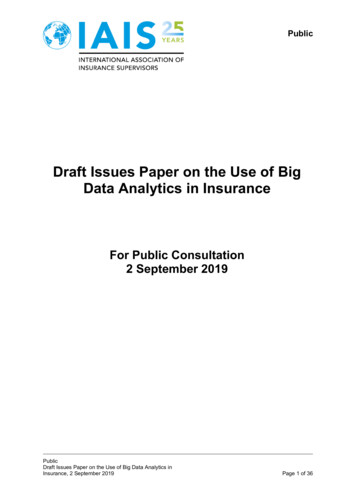
Transcription
PublicDraft Issues Paper on the Use of BigData Analytics in InsuranceFor Public Consultation2 September 2019PublicDraft Issues Paper on the Use of Big Data Analytics inInsurance, 2 September 2019Page 1 of 36
About the IAISThe International Association of Insurance Supervisors (IAIS) is a voluntary membershiporganisation of insurance supervisors and regulators from more than 200 jurisdictions. Themission of the IAIS is to promote effective and globally consistent supervision of the insuranceindustry in order to develop and maintain fair, safe and stable insurance markets for the benefitand protection of policyholders and to contribute to global financial stability.Established in 1994, the IAIS is the international standard setting body responsible fordeveloping principles, standards and other supporting material for the supervision of theinsurance sector and assisting in their implementation. The IAIS also provides a forum forMembers to share their experiences and understanding of insurance supervision andinsurance markets.The IAIS coordinates its work with other international financial policymakers and associationsof supervisors or regulators, and assists in shaping financial systems globally. In particular,the IAIS is a member of the Financial Stability Board (FSB), member of the Standards AdvisoryCouncil of the International Accounting Standards Board (IASB), and partner in the Access toInsurance Initiative (A2ii). In recognition of its collective expertise, the IAIS also is routinelycalled upon by the G20 leaders and other international standard setting bodies for input oninsurance issues as well as on issues related to the regulation and supervision of the globalfinancial sector.Issue Papers provide background on particular topics, describe current practices, actualexamples or case studies pertaining to a particular topic and/or identify related regulatory andsupervisory issues and challenges. Issues Papers are primarily descriptive and not meant tocreate expectations on how supervisors should implement supervisory material. IssuesPapers often form part of the preparatory work for developing standards and may containrecommendations for future work by the IAIS.International Association of Insurance Supervisorsc/o Bank for International SettlementsCH-4002 BaselSwitzerlandTel: 41 61 280 8090 Fax: 41 61 280 9151www.iaisweb.orgThis document was prepared by the Market Conduct Working Group in consultation with IAISMembers.This document is available on the IAIS website (www.iaisweb.org). International Association of Insurance Supervisors (IAIS), 2019.All rights reserved. Brief excerpts may be reproduced or translated provided the source isstated.PublicDraft Issues Paper on the Use of Big Data Analytics inInsurance, 2 September 2019Page 2 of 36
ContentsExecutive summary . 4Acronyms . 5123Introduction . 61.1Background and purpose . 61.2Approach and structure . 7Sources, collection and processing of data in insurance . 92.1Sources and collection of data . 92.2Processing of data . 11The use of big data analytics across the insurance product lifecycle . 143.13.1.1Personalised insurance cover . 143.1.2Targeted marketing . 163.1.3Sales execution . 173.1.4Distribution and advice . 183.2Pricing and underwriting. 193.2.1Increased granularity in risk selection and pricing . 193.2.2Price optimisation . 203.2.3Underwriting . 213.34Product design, marketing, sales and distribution . 14Claims handling . 243.3.1Risk mitigation and loss reduction . 243.3.2Claims processing . 253.3.3Fraud detection . 27Supervisory considerations . 304.1Suitability, affordability and availability of insurance cover . 314.2Governance and oversight of algorithms . 324.3Third party risk management . 354.4Issues around privacy, ownership and sources of data . 35PublicDraft Issues Paper on the Use of Big Data Analytics inInsurance, 2 September 2019Page 3 of 36
Executive summary1.This paper builds on the IAIS Issues Paper on Increasing Digitalisation in Insuranceand its Potential Impact on Consumer Outcomes (November 2018) 1 by focusing morespecifically on issues relating to the use of personal and other data by insurers as a result ofdigitalisation.2.The scope of this paper focuses on the use of algorithms and advanced analyticscapabilities by insurers to make decisions based on patterns, trends and linkages and theavailability to insurers of new alternative data sources, collectively referred to as “big dataanalytics” (BDA).3.To help understand the potential benefits and risks of the use of BDA by insurers, thispaper considers the manner in which insurers are now able to collect, process and use dataacross various stages of the insurance product lifecycle, namely product design, marketing,sales and distribution, pricing and underwriting and claims handling.4.Furthermore, in light of the outcomes for the fair treatment of customers described inInsurance Core Principles (ICPs) 18 and 19, this paper makes certain observations about thepotential implications for supervisors as a result of the use of BDA in insurance.5.The paper observes that the increased availability of data and enhanced processingcapabilities now accessible to insurers can result in a number of benefits. The granularity ofdata from multiple sources can lead to more personalised and affordable insurance productsas well as more efficient servicing for customers. Insurers can also benefit from BDA byexpanding their distribution reach, ensuring more accurate pricing and lowering their costmargins due to claims savings and better fraud detection.6.On the other hand, the paper also highlights that the complexity and opacity ofalgorithm technology and the ability of insurers to customise product offerings to an individuallevel could potentially result in risks to individual customers, as well as to the insurance sectoras a whole, for which supervisors may need to devise appropriate responses. In particularsupervisors may wish to consider whether the use of BDA could adversely impact theavailability and affordability of insurance, potentially resulting in reduced options or nocoverage for certain consumer segments. Additionally the paper suggests that supervisorsthink about whether there is a need to enhance governance, oversight and third party riskmanagement requirements specific to the use of algorithms for BDA purposes.7.While the collection, use and processing of data naturally raises issues about privacyprotection and related matters, the supervision of such matters often falls outside the remit ofinsurance supervisors, and is not covered within the scope of this paper except to the extentaddressed in Standard 19.12 l-impact-on-consumer-outcomes .2 Standard 19.12 states that [t]he supervisor requires insurers and intermediaries to have policies andprocedures for the protection and use of information on customers.PublicDraft Issues Paper on the Use of Big Data Analytics inInsurance, 2 September 2019Page 4 of 36
AcronymsABIAssociation of British InsurersAIDAArtificial Intelligence and Data AnalyticsAPIsApplication Programming InterfacesACCCAustralian Competition and Consumer CommissionAFMAutoriteit Financiële Markten (Netherlands)AIArtificial IntelligenceBaFinBundesanstalt für Finanzdienstleistungsaufsicht (Germany)BDABig Data AnalyticsEIOPAEuropean Insurance and Occupational Pensions AuthorityFACIFederal Advisory Committee on Insurance (USA)FCAFinancial Conduct Authority (UK)FEATFairness, Ethics, Accountability and TransparencyFSCAFinancial Sector Conduct Authority (South Africa)GIAGeneral Insurance Association (Singapore)GINAGenetic Information Nondiscrimination Act of 2008 (USA)HKFIHong Kong Federation of InsurersIAISInternational Association of Insurance SupervisorsICPsInsurance Core PrinciplesIIBInsurance Information Bureau of IndiaIoTInternet of ThingsIRDAInsurance Regulatory and Development Authority (India)MASMonetary Authority of SingaporeNAICNational Association of Insurance Commissioners (USA)SAICBSouth African Insurance Crime BureauTCIPTurkish National Catastrophe Insurance PoolUBIUsage Based InsurancePublicDraft Issues Paper on the Use of Big Data Analytics inInsurance, 2 September 2019Page 5 of 36
11.1IntroductionBackground and purpose9.In November 2018 the IAIS published an Issues Paper on Increasing Digitalisation inInsurance and its Potential Impact on Consumer Outcomes 3 (Digitalisation Paper). That paperconsidered generally the impact of various technological innovations on consumer outcomesand insurance supervision in light of Insurance Core Principles (ICPs) 18 on Intermediariesand 19 on Conduct of Business.10.One of the areas identified in the above paper was the increased availability of, andaccess to, consumer related-data by insurers as a result of many of the innovations describedin that paper. The IAIS signalled that this would be an area for further exploration anddiscussion in a separate paper.11.The purpose of this paper is therefore to consider the use of personal and other databy insurers as a result of digitalisation and to identify the benefits and risks for consumersassociated with such use. Specifically this paper focuses on the increasing use of algorithmsand advanced data analytics by insurers, as well as the greater availability to insurers oftraditional and non-traditional (alternative) data sources. In the paper this will be collectivelyreferred to as “big data analytics” (BDA).12.Where data is processed and held by intermediaries, as may be the case in certaincircumstances depending on their involvement in the insurance value chain, the issuesidentified in this paper will also be applicable as and when appropriate to intermediaries. Inthese circumstances references to “insurer” in the paper would include insuranceintermediaries.13.In this paper “consumer” refers to the “universe of all actual and potential customersfor insurance products” and “customer” refers to the “[p]olicyholder or prospective policyholderwith whom an insurer or insurance intermediary interacts, and includes, where relevant, otherbeneficiaries and claimants with a legitimate interest in the policy”, as defined in the IAISGlossary. 414.This paper does not go into technical detail on the various types of technologies 5available to insurers as a result of digitalisation, but rather discusses various applications ofBDA by insurers across the insurance product lifecycle and considers the potential impact ofthese applications on outcomes for consumers and possible implications for supervisors as aresult.15.Insurers are making greater use of BDA either in direct customer interactions (egautomated advice and chatbot support during sales processes) or more indirectly (eg targetedadvertising, product design, credit assessment, customer identification and verification). l-impact-on-consumer-outcomes .4 glossary .5 Previous IAIS material describing the various types of technological innovations available to clusive-insurance; IAIS Digitalisation Paper .PublicDraft Issues Paper on the Use of Big Data Analytics inInsurance, 2 September 2019Page 6 of 36
addition the diversification of available customer data sources has created opportunities forgreater individualisation of risks and pricing by insurers.16.While such individualisation may help insurers to better understand customer needsand preferences, it could lead to accessibility and affordability challenges and possible marketexclusion for some customers. The opacity of algorithms may lead to decreased comparabilityof products, especially if customers are only able to see or access product offerings that areindividually tailored.17.Customer segmentation on the basis of risk classes is a core feature of privateinsurance. Higher risk customers generally pay higher premiums than lower risk customers.The application of BDA may allow insurers to cluster customers in increasingly refined riskcategories for different treatment in respect of risk selection, marketing, sales execution,underwriting, pricing and claims settlement. However, this could also reduce access toinsurance and the availability and affordability of insurance for certain segments of thepopulation.18.ICPs 18 and 19 contemplate the delivery of fair customer outcomes across theinsurance value chain irrespective of the adoption of technology or the use of more traditionalmeans to design, market, sell, distribute or service insurance policies. A thorough and ongoingunderstanding of the benefits and risks associated with the application of BDA in insurancecan help supervisors to develop more appropriate and proportionate responses to suchapplications by insurers while ensuring that the fair treatment of customers is notcompromised.19.The application of BDA also raises important questions related to the privacy,ownership, transferability and ethical use of data. Since privacy protection issues fall outsidethe remit of insurance supervisors in many jurisdictions, this paper does not cover these issuesin detail except to the extent set out in ICP 19. Standard 19.12 requires supervisors to ensurethat insurers have policies and procedures in place for the protection and use of customerdata.1.2Approach and structure20.In line with the approach adopted in the previous Digitalisation Paper, this paperdiscusses the applications of BDA across various elements of the insurance product lifecycle.The specific elements covered are: product design, marketing, sales and distribution, pricingand underwriting, and claims handling.21.The paper is structured as follows: section 2 provides an overview of traditional and alternative sources, collectionmethods and processing of data by insurers; section 3 discusses potential applications of BDA across certain aspects of theinsurance product lifecycle, and the associated benefits and risks of such applications;andPublicDraft Issues Paper on the Use of Big Data Analytics inInsurance, 2 September 2019Page 7 of 36
6section 4 concludes with key considerations, supplementary to those highlighted in theprevious Digitalisation Paper 6, aimed at assisting supervisors to determine possibleresponses to the issues discussed in section 3.IAIS Digitalisation Paper at page 24.PublicDraft Issues Paper on the Use of Big Data Analytics inInsurance, 2 September 2019Page 8 of 36
2Sources, collection and processing of data in insurance2.1Sources and collection of data22.The insurance sector is heavily reliant on various types of data, used for manypurposes and drawn from multiple sources. Some examples are listed in the following table: 7Type of dataDemographicdataMedical dataExposure dataBehaviouraldataLoss dataExamplesAge, gender, civil andfamily status, profession,addressMedical history, medicalcondition, condition offamily members, genetictestingType of car, value ofbuilding contents, typeand features of nayear,deductible choice, lifeinsurance lapse ratesClaim reports from caraccidents, liability casesPersonal / nonUse 8personalPanel A: Traditional dataData sourcePersonalRisk selectionPolicyholdersPersonalRisk selectionPolicyholdersPersonal/non-personalRisk onexchange within industryindustryPopulationdataMortality rates, morbidityrates, car accidentsAnonymised andaggregatedpersonal dataRisk selectionGovernment,industrystatistics, academiaHazard dataFrequency and severity ofnatural hazardsNon-personalRisk selectionOthertraditional dataCredit reference, claimadjustmentreports,information from the autorepair shopsPersonal/non-personalRiskselection,marketing, claimsmanagementGovernment,industrystatistics, academiaPanel B: New and ‘non-traditional’ tion,activityandmedical illance (smart home)IoT ders, credit agents,partner adjusters or agenciesinvolved in the claimData collection devicesWeb searches, onlinebuying behaviour, socialmedia ycompanies(Internet providers, searchengineproviders,ecommerce providers, socialmedia platforms)Mobile phone operatingsystem, email addressesPersonalRiskselection,marketing, productdevelopmentTechnology companies,PolicyholdersSource: Big Data and Insurance: Implications for Innovation, Competition and Privacy, GenevaAssociation, March 2018. The table does not represent an exhaustive list of data types and sources.Depending on the circumstances insurers may collect other types of data from other sources notspecifically listed in the table.8 Here risk selection includes pricing and underwriting; marketing includes distribution and salesactivities; claims management includes fraud detection.7PublicDraft Issues Paper on the Use of Big Data Analytics inInsurance, 2 September 2019Page 9 of 36
23.Insurers may collect data actively or passively through direct or indirect means.24.Active data collection usually occurs during application and registration processeswhere customers are specifically requested or required to share certain data. With passivecollection, on the other hand, customers may sometimes unknowingly share data (eg byproviding or sharing location data through mobile or telematics devices).25.Insurers may collect data from customers as a result of direct interactions, or indirectlythrough outsourced service providers or other third parties (eg credit scoring, backgroundverification, marketing or advertising companies, or consumer and claims handlingdatabases).26.Offline collection of data may be done in person, in writing, through manual forms orover the phone, while online collection may be done through web browsers, social mediaplatforms, game consoles, tablets, smart devices and other types of electronic communication.27.Digitalisation in insurance has resulted in the availability and use of new data sources,also known as “alternative data”. This type of data is not collected or produced by the insureras result of direct customer interactions but taken from indirect sources such as mobiledevices, sensors, satellite technology and the internet. Since alternative data sets can bevoluminous, they require the application of algorithms and advanced analytics to help derivemeaningful and usable insights, as described in subsection 2.2 below.28.Depending on the source and method of collection, data may be accessible to insurerswithin an insurance context or outside of it, thereby leaving the original context for which thesharing of the specific data was intended. For example, data collected from a telematics devicewould be within an insurance context, whereas data collected from Facebook would be outsidean insurance context.Examples:NetherlandsIn the Netherlands, an important source of data is MijnOverheid, where personal data isstored from different institutions, such as government services and pension funds. Forexample, customers that ask financial planners to develop financial plans, can downloadtheir pension data from MijnOverheid and then upload it to the server of their financialplanners. This way, all their pension entitlements are immediately taken into account fortheir financial plans. Other data in MijnOverheid is an individual’s income data.SingaporeA key component of Singapore’s National Digital Identity system is MyInfo, a digital servicethat enables citizens to authorise third parties to access their personal data that is storedacross different government agencies. With consent, third parties can use MyInfo’sapplication programming interfaces (APIs) to access government-verified data toauthenticate their customers and make business decisions relating to them. Currentlymore than 20 financial institutions are using MyInfo to provide more than 110 digitalfinancial services.European UnionThe European Insurance and Occupational Pensions Authority (EIOPA) recentlyconducted a thematic review on the use of BDA in motor and health insurance. The reviewshowed how traditional data sources such as demographic data or exposure data areincreasingly combined (not replaced) with new sources like online media data or telematicsPublicDraft Issues Paper on the Use of Big Data Analytics inInsurance, 2 September 2019Page 10 of 36
data, providing greater granularity and frequency of information about consumers’characteristics, behaviour and lifestyles. 9 This enables the development of more tailoredproducts and services and more accurate risk assessments.2.2Processing of data29.The processing of customer data, whether done in-house or by an outsourced thirdparty provider, is a critical component of an insurer’s business. It helps inform, for example,the insurer’s business and marketing strategies, service delivery and distribution models,product range, risk selection and pricing structures.30.Digitalisation has resulted in significant changes to the manner in which data can beprocessed and leveraged by insurers as they now have access to greatly enhanced levels ofcomputing power and analytical capabilities.31.The increased availability of customer data, more sophisticated tools for collecting,safeguarding, transferring and analysing this data, and growing appetite for the use of newtechnologies have created increased opportunities for insurers to adopt the use of algorithmsfor various purposes.32.Algorithms can be used by insurers for direct customer servicing (eg to provideautomated advice and pre- and post-sales support) or more indirectly (eg to design targetedadvertising campaigns, obtain insights on consumer preferences, influence consumerbehaviour, inform product design, risk selection and pricing, assess credit worthiness, conduct“know your customer” and other identification and verification checks).33.The increasing reliance on the use of algorithms by insurers for the processing ofcustomer data may create a number of challenges and risks.34.Algorithms can be complex and are often treated as proprietary and highly confidentialin nature. Thus, there can be a lack of transparency and strong asymmetry of understandingbetween those who design and use algorithms and customers and supervisors trying tounderstand the outcomes generated by these algorithms.35.Machine learning algorithms are based on historical data and, therefore, generallyreproduce the past. This can increase the likelihood that algorithms may perpetuateunforeseen biases, which in turn create risks of errors potentially resulting in inequitable orunsuitable customer outcomes.36.Additionally, the effectiveness of an algorithm is dependent on the quality, accuracyand completeness of available data, and can be hampered by possible errors in its initialdesign or programming.37.There is also a risk that customer segments could be differentiated on the basis offalse assumptions or false conclusions drawn by algorithms on the basis of theseassumptions, resulting in unlawful discrimination against certain customers. 10Example:United Kingdom9https://eiopa.europa.eu/Publications/EIOPA BigDataAnalytics ThematicReview April2019.pdf .10https://www.bafin.de/SharedDocs/Downloads/EN/dl bdai studie en.html at page 177.PublicDraft Issues Paper on the Use of Big Data Analytics inInsurance, 2 September 2019Page 11 of 36
In the UK, a BBC investigation compared car insurance quotes from the five leading pricecomparison websites, first using the name of a white British BBC producer, and then adifferent common British name, ‘Muhammad Khan’. All five sites returned higher prices forMuhammad Khan. 1138.Enhancements in machine learning capabilities may also result in the operation ofalgorithms becoming increasingly complex even for those who design them.39.Algorithms may be used alongside, rather than instead of, established techniques suchas generalised linear models, to provide new insights to insurers. Nevertheless thegovernance and oversight of algorithm applications may prove to be challenging and couldrequire specific technical expertise and more robust auditing mechanisms within insurers andsupervisors to adequately mitigate risks that could potentially arise as a result of the abovefactors.Examples:NetherlandsThe Netherlands Autoriteit Financiële Markten (AFM) has published guidance on thedevelopment of algorithms used in robo advice. A key requirement is testing of thealgorithm, both before and after launching the robo advisor. When developing the roboadvice, the insurer must be satisfied that sufficient knowledge of financial products ispresent in order to ensure that the robo advice is of sufficient quality. The developmentand testing of the robo advice must be carried out thoroughly enough so that it can bedemonstrated that the advice is suitable when the system goes live.The supervisor’s approach to supervising robo advice and its underlying algorithms is asfollows:(i) the supervisor will assess the input and output of the robo advisor. This is equivalent tothe supervision of physical advice files where consideration is given to whether all relevantdata has been obtained and used in order to arrive at the appropriate outcome based onthe customer’s needs and circumstances;(ii) the supervisor will assess the conditions under which the algorithm was developed inorder to determine whether it was developed with enough knowledge of the advice processand adequately tested; and(iii) the supervisor is now also considering whether it should directly supervise the designof the algorithm itself.SingaporeIn its Guidelines on Provision of Digital Advisory Services, the Monetary Authority ofSingapore (MAS) requires digital advisers to put in place adequate governance andsupervisory arrangements to effectively mitigate the risks that stem from the use ofalgorithms. 12 These include requiring:(i) board and senior management of digital advisers to maintain effective oversight andensure that there are sufficient resources to monitor and supervise the performance of thealgorithms; GG02.pdf .PublicDraft Issues Paper on the Use of Big Data Analytics inInsurance, 2 September 2019Page 12 of 36
(ii) digital advisers to have in place policies and procedures governing the development,monitoring and testing of algorithms, as well as when changes are made to the algorithms.PublicDraft Issues Paper on the Use of Big Data Analytics inInsurance, 2 September 2019Page 13 of 36
3The use of big data analytics across the insurance product lifecycle40.BDA can be used across various components of the insurance product lifecycle, asshown by the examples in diagram 1 below.41.This paper does not cover all the elements contained in diagram 1, but rather focuseson a few key areas of the lifecycle where the use of BDA has been identified as potentiallyintroducing significant benefits and risks for insurers, consumers and supervis
Draft Issues Paper on the Use of Big Data Analytics in Insurance, 2 September 2019 Page 6 of 36 1 Introduction 1.1 Background and purpose 9. In November 2018 the IAIS published Issues Paper on Increasing Digitalisation in an Insurance and its Potential Impact on Consumer Outcomes 3 (Digitalisation Paper ). That paper