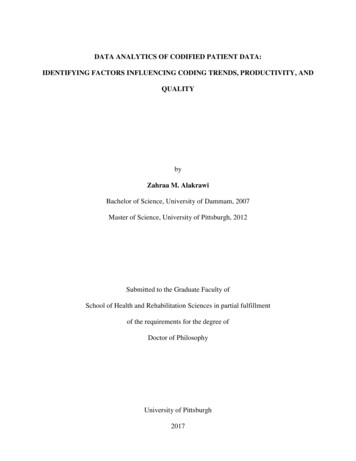
Transcription
TITLE PAGEDATA ANALYTICS OF CODIFIED PATIENT DATA:IDENTIFYING FACTORS INFLUENCING CODING TRENDS, PRODUCTIVITY, ANDQUALITYbyZahraa M. AlakrawiBachelor of Science, University of Dammam, 2007Master of Science, University of Pittsburgh, 2012Submitted to the Graduate Faculty ofSchool of Health and Rehabilitation Sciences in partial fulfillmentof the requirements for the degree ofDoctor of PhilosophyUniversity of Pittsburgh2017
COMMITTEEUNIVERSITY OF PITTSBURGHSCHOOL OF HEALTH AND REHABILITATION SCIENCESThis dissertation was presentedbyZahraa M. AlakrawiIt was defended onMarch 31, 2017and approved byMervat Abdelhak, Ph.D., RHIA, FAHIMAChair and Associate Professor, Health Information ManagementMelanie M. Hughes, Ph.D.Associate Professor, SociologyPatricia Anania Firouzan, MSIS, RHIAAssistant Professor, Health Information ManagementPatty Sheridan, MBA, RHIA, FAHIMA,Ciox Health, Senior Vice President, HIM Business DevelopmentDissertation Advisor: Valerie Watzlaf, Ph.D., RHIA, FAHIMA,Associate Professor, Health Information ManagementiiDissertation Advisor: Valerie Watzlaf,Ph.D., RHIA, FAHIMA,Associate Professor, Health Information Management
COPYRIGHTCopyright by Zahraa M. Alakrawi2017iii
ABSTRACTDATA ANALYTICS OF CODIFIED PATIENT DATA:IDENTIFYING FACTORS INFLUENCING CODING TRENDS, PRODUCTIVITY,AND QUALITYZahraa M. Alakrawi, Ph.D.University of Pittsburgh, 2017Cost containment and quality of care have always been major challenges to the health care deliverysystem in the United States. Health care organizations utilize coded clinical data for health caremonitoring, and reporting that includes a wide range of diseases and clinical conditions along withadverse events that could occur to patients during hospitalization. Furthermore, coded clinical datais utilized for patient safety and quality of care assessment in addition to research, education,resource allocation, and health service planning.Thus, it is critical to maintain high quality standards of clinical data and promote fundingof health care research that addresses clinical data quality due to its direct impact on individualhealth outcomes as well as population health. This dissertation research is aimed at identifyingcurrent coding trends and other factors that could influence coding quality and productivitythrough two major emphases: (1) quality of coded clinical data; and (2) productivity of clinicalcoding. It has adopted a mix-method approach utilizing varied quantitative and qualitative dataanalysis techniques. Data analysis includes a wide range of univariate, bivariate, and multivariateanalyses.Results of this study have shown that length of stay (LOS), case mix index (CMI) and DRGrelative weight were not found to be significant predictors of coding quality. Based on thequalitative analysis, history and physical (H&P), discharge summary, and progress notes wereiv
identified as the three most common resources cited by Ciox auditors for coding changes. Also,results have shown that coding productivity in ICD-10 is improving over time. Length of stay, casemix index, DRG weight, and bed size were found to have a significant impact on codingproductivity. Data related to coder’s demographics could not be secured for this analysis.However, factors related to coders such as education, credentials, and years of experience arebelieved to have a significant impact on coding quality as well as productivity. Linking coder’sdemographics to coding quality and productivity data represents a promising area for futureresearch.v
TABLE OF CONTENTSPREFACE . XVIII1.0INTRODUCTION . 12.0HEALTHCARE VOCABULARY, TERMINOLOGY, AND CLASSIFICATION . 82.1.1HealthCare Terminology . 122.1.2Healthcare Classifications . 152.1.2.1 Reference Classifications .162.1.2.2 Derived Classifications .162.1.2.3 Related Classifications .162.1.3WHO Family of International Classification (FIC) . 172.1.3.1 Purpose of the WHO-FIC .172.1.3.2 UN definition of the WHO-FIC .172.1.3.3 Scope of the WHO Family.182.1.4Use of Vocabulary, Terminology, and Classification Systems . 182.1.5Clinical Terminology and Clinical Classification Systems: A Critique UsingAHIMA’s Data Quality Management DQM Model . 232.1.5.1 AHIMA’s DQM Model .242.1.5.2 Users and Applications .332.1.5.3 Clinical Documentation into the EHR .353.0CLINICAL CODING PROCESSES . 373.1CLINICAL CODING . 37vi
3.2CODING CLINICAL EXPRESSIONS USING SNOMED CT AND ICD-10-CM/PCS .393.3INPATIENT V. OUTPATIENT CODING . 423.4INPATIENT CODING CLASSIFICATION SYSTEMS; ICD-9-CM, ICD-10, ICD-10-CM/PCS, AND ICD-11 . 444.0REIMBURSEMENT METHODS IN HEALTHCARE . 514.1INPATIENT PROSPECTIVE PAYMENT SYSTEM (IPPS) . 514.2OUTPATIENT PROSPECTIVE PAYMENT SYSTEM (OPPS) . 574.3FEDERAL LAWS AND REGULATIONS . 585.0CLINICAL CODING VALIDATION, CODED DATA QUALITY, ANDPRODUCTIVITY-DRIVEN PRACTICES . 605.1INTERNAL AUDIT PROGRAMS . 605.1.1Outsourcing approach . 605.1.2Merging approach . 615.1.3Coordination approach . 615.2THE RECOVERY AUDIT PROGRAM AND MEDICARE . 635.2.1Background on Medicare . 635.2.2RAC Audit Program . 645.3ADMINISTRATIVE DATA QUALITY & PRODUCTIVITY. 675.4COMPUTER-ASSISTED CODING (CAC). 776.0STUDY SIGNIFICANCE. 817.0SPECIFIC AIMS AND RESEARCH QUESTIONS . 85vii
7.1.1Specific Aim I: Identifying factors that could influence coding accuracy: .867.1.1.1 Length of Stay (LOS) .867.1.1.2 Case Mix Index (CMI) .867.1.1.3 DRG Relative Weight .867.1.1.4 MS DRG categories .877.1.1.5 Digit Level .877.1.2Specific Aim II: Identifying documentation discrepancies that couldinfluence coding quality . 887.1.3Specific Aim III: Identifying impact of coding errors on CMI and hospital’spayment . 897.1.4Specific Aim IV: Identifying individual and facility-related factors thatcould influence coding productivity . 897.1.4.1 Length of Stay (LOS) .907.1.4.2 Case Mix Index (CMI) .907.1.4.3 DRG Relative Weight .907.1.4.4 Bed Size .907.1.4.5 Teaching Status .917.1.4.6 Trauma Status .917.1.5Specific Aim V: Explore the relationship between coding productivity andcoding quality . 937.1.6Specific Aim VI: Develop predictive models to predict coding productivityand coding quality based on the individual and facility-related factors. . 93viii
8.0METHODOLOGY. 948.1STUDY DESIGN . 948.2SAMPLING DESIGN . 958.3SAMPLE SIZE. 958.4DATA COLLECTION . 958.4.1Accuracy Data Set . 968.4.2Productivity Data Set . 978.59.0DATA ANALYSIS . 98RESULTS . 999.1IDENTIFYING FACTORS THAT COULD INFLUENCE CODINGACCURACY . 999.1.1Descriptive Statistics. 1009.1.2Bivariate Analyses . 1129.2IDENTIFYING DOCUMENTATION DISCREPANCIES THAT COULDINFLUENCE CODING QUALITY . 1149.39.2.1Unspecified codes rate . 1149.2.2Physician Query Rate . 1159.2.3Most Frequent Errors By ICD-10 Chapters . 1159.2.4Source Documents Used to Identify Coding Errors . 1189.2.5Most Frequent Errors Related to Coding Guidelines . 120IDENTIFYING IMPACT OF CODING ERRORS ON CMI ANDHOSPITAL’S PAYMENT . 121ix
9.4IDENTIFYING INDIVIDUAL AND FACILITY-RELATED FACTORSTHAT COULD INFLUENCE CODING PRODUCTIVITY . 1249.4.1Descriptive Analyses . 1249.4.2Bivariate Analyses . 1469.4.3Multiple Linear Regression . 1509.4.4Hierarchical Linear Modeling . 1539.5IDENTIFYING IMPACT OF CODING TIME ON PRODUCTIVITY . 1559.6DEVELOPING A PREDICTIVE MODEL TO PREDICT CODINGQUALITY AND PRODUCTIVITY . 1569.6.1Conceptual Framework: . 1569.6.2Prediction of Coding Productivity . 1579.6.3Prediction of Coding Quality . 1579.6.4Case Studies. 1599.6.4.1 Case Study 1: Circulatory System .1599.6.4.2 Case Study 2: Musculoskeletal System and Connective Tissues .1639.6.4.3 Case Study 3: Oncology .16710.0DISCUSSION . 17211.0LIMITATIONS . 17612.0FUTURE WORK . 17713.0CONCLUSION. 178APPENDIX A . 179GLOSSARY . 179x
EXAMPLES I: SOURCE DOCUMENTS USED TO IDENTIFY CODING ERRORS . .182EXAMPLES II: ERRORS RELATED TO CODING GUIDELINES . 186EXAMPLES III: RECOMMENDED CHANGES PER DOCUMENTATION . 189BIBLIOGRAPHY . 191xi
LIST OF TABLESTable 1: Reasons for needing a vocabulary, terminology, or classification system . 10Table 2. Differences between vocabulary, terminology and classification systems based on chosengoal and users . 21Table 3. DQM Domains and Characteristics with Definitions . 25Table 4. Example on Comprehensiveness of Both Systems . 28Table 5. Examples on the Different Language Used by Both Systems (Data Definition) . 30Table 6. Examples of Natural Language Expressions Coded in SNOMED-CT and ICD-10-CM 41Table 7. Difference between inpatient and outpatient coding . 43Table 8. Comparison between ICD-9-CM and ICD-10-CM/PCS . 46Table 9. Examples of MS-DRGs with different weights (FY 2016) . 53Table 10. Total payment for each case based on hospital rate. 54Table 11. States Covered by Each RAC Contractor . 66Table 12. Summary of Specific Aim I . 87Table 13. Summary of Specific Aim III . 89Table 14. Summary of Specific Aim IV . 91Table 15. Summary of Specific Aim V . 93Table 16. Descriptive Statistics (Accuracy Data) . 100Table 17. Most Frequent Principle Diagnoses . 101Table 18. Most Frequent Secondary Diagnoses . 102Table 19. Most Frequent Principal Procedures . 103xii
Table 20. Most Frequent DRGs . 104Table 21. Number of different digits between coders & reviewers . 106Table 22. Accuracy Statistics . 109Table 23. Accuracy Rate based on the Most Frequent DRGs . 110Table 24. Most Frequent DRG Changes Due to Principle Diagnosis. 111Table 25. Zero-order correlations among the variables (Accuracy) . 113Table 26. Distribution of Errors by ICD-10 Chapters . 116Table 27. Most Frequent Errors: Top Five ICD-10 Chapters . 117Table 28. Source Documents used to Change the Assigned Codes (Nvivo) . 119Table 29. Most Frequent Errors Related to Coding Guidelines (Nvivo) . 120Table 30: Most Frequent Sequencing Errors and Their Impact on Payment . 123Table 31. Descriptive Statistics of Productivity Data . 126Table 32. Coding time by teaching and trauma status. . 131Table 33. Coding time by bed size. 132Table 34. Average Coding Time by MDC. 134Table 35. Average Coding Time by DRGs . 138Table 36. Zero-order correlations among the variables (productivity data) . 146Table 37. Predicting Coding Productivity Based on LOS & CMI . 150Table 38. Predicting Coding Productivity Based on LOS, CMI, DRG Weight, Bed Size, TraumaStatus, and Teaching Status . 151Table 39. Hierarchical Linear Model to Predict Coding Time based on LOS and CMI bed size, andDRG weight . 154Table 40: Prediction of Coding Productivity (Final Models) . 158xiii
Table 41. Descriptive Statistics (Circulatory System) . 159Table 42. Average Coding Time by DRG Type (Circulatory System) . 160Table 43. Average Coding Time by DRG (Circulatory System). 161Table 44: Predicting Mean Coding Time for Circulatory System . 163Table 45. Descriptive Statistics (Musculoskeletal System and Connective Tissues) . 164Table 46. Average Coding Time by DRG Type (Musculoskeletal System and Connective Tissues). 164Table 47. Average Coding Time by DRGs (Musculoskeletal System and Connective Tissues) 165Table 48. Predicting Mean Coding Time for Musculoskeletal System and Connective Tissues 167Table 49. Descriptive Statistics (Oncology) . 168Table 50. Average Coding Time by DRG Type (Oncology) . 168Table 51. Average Coding Time by DRGs (Oncology) . 169Table 52. Predicting Mean Coding Time for Oncology . 171xiv
LIST OF FIGURESFigure 1: Conceptual Framework to Literature Review . 6Figure 2: The level of detail given by a vocabulary, classification system, and terminology . 8Figure 3: History of SNOMED . 13Figure 4: WHO-FIC with Examples . 19Figure 5: AHIMA’s DQM Model; Comparing Data Quality of SNOMED-CT and ICD-10-CM 32Figure 6: Coding Natural Language Clinical Phrases Using SNOMED CT and ICD 10-CM . 40Figure 7: Revisions of ICD . 44Figure 8: A coding Scenario Using ICD-10-CM/PCS. 49Figure 9: ICD-11 Timeline . 50Figure 10: Top 10 Principal Diagnoses . 102Figure 11: Top 10 Secondary Diagnoses . 103Figure 12: Top 5 principal procedures . 104Figure 13: Most frequent DRGs . 105Figure 14: Distribution of Coding Errors (%). 106Figure 15: Distribution of Coding Errors Based on 3rd, 4th, and 5th Digits . 107Figure 16: Distribution of ICD-10 Coding Errors Based on GEMs . 117Figure 17: Source Documents Used for Coding Changes . 119Figure 18: Distribution of Errors by Coding Guidelines . 121Figure 19: Average Coding Time for 10-month period (October 2015-July 2016) . 127Figure 20: Comparing Standard Coding Productivity Times . 128xv
Figure 21: Average Coding Time by LOS . 128Figure 22: Average Coding Time by CMI . 129Figure 23: CMI and Coding Time by Month . 130Figure 24: Top Three DRGs by Month (March - July 2016) . 130Figure 25: Coding time by bed size . 133Figure 26: Coding Time by MDCs . 137Figure 27: Coding Time by Length of Stay . 147Figure 28: Coding Time by Bed Size . 148Figure 29: Coding Time by CMI . 148Figure 30: Coding Time by DRG Relative Weight . 149Figure 31: Correlation Between CMI and LOS . 149Figure 32: Relationship Between Coding Accuracy and Productivity (Coding Time) . 155Figure 33: Conceptual Framework of Factors Influencing Coding Quality & Productivity . 156Figure 34: Coding Time by DRGs (Circulatory System) . 162Figure 35: Coding Time by DRGs (Musculoskeletal System & Connective Tissues) . 166Figure 36: Coding Time by DRG (Oncology) . 170Figure 37. Cases in Which H&P Was Used for Coding Change . 182Figure 38. Cases in Which Discharge Summary Was Used for Coding Change . 183Figure 39. Cases in Which Progress Notes Were Used for Coding Change . 183Figure 40. Cases in Which Anesthesia and Operative Report Were Used for Coding Change . 184Figure 41. Cases in Which Consultation Notes Were Used for Coding Change . 184Figure 42. Cases in Which Psychiatric Evaluation Was Used for Coding Change . 184Figure 43. Cases in Which Different Sources Were Used for Coding Change . 185xvi
Figure 44: Changes Related to Principle Diagnosis . 187Figure 45: Changes Related to Secondary Diagnoses . 187Figure 46: Changes Related to Combination Codes . 187Figure 47: Changes Related to Symptoms & Signs . 187Figure 48: Changes Related to V Codes . 188Figure 49: Changes Related to CC/MCC/POA . 188Figure 50: Changes Related to Place of Occurrence . 188Figure 51: Lack of Supporting Documentation . 189Figure 52: Addition and Deletion Per Documentation . 190Figure 53: Recommended Query . 190xvii
PREFACEI have always been longing to this moment to arrive, to fill my heart with joy, to finally keep it asa precious memory in my mind and inside my heart. It has been such a long yet a pleasant journey.Through this journey, I have met so many people who made me the person I am today, includingthe nice strangers who I ran into at Starbucks.I have been longing to this moment, to write the “preface” of my dissertation, toacknowledge all the great people who believed in me and brought this dissertation to life. First, Iwould like to express my sincerest thanks and deepest gratitude to my dissertation advisor andcommittee chair, Dr. Valerie Watzlaf, for her dedicated mentoring, never-ending support, andwonderful guidance in the last five years. Thank you for teaching me how to accept myweaknesses, learn from my mistakes, and grow and cherish every opportunity to be a better versionof me. I would like to thank my dissertation committee for their guidance and support throughoutthis journey: Dr. Mervat Abdelhak, Dr. Melanie Hughes, Patricia Anania Firouzan, and PattySheridan.I would like to express my heartfelt thanks, gratefulness, and gratitude for Batool Al Yousif& Moosa Alakrawi, my beloved parents, for their endless love, support, encouragement, andprayers. Also, I would like to thank my lovely sisters, Mariam and Khatoon, for always being bymy side. Last, but not least, I am especially thankful to my beloved husband, Raid Al Jomaia.Thank you for your love, your care, and unlimited support. Thank you for all meals, for all theflowers, for lifting me up when I am down, and for all the jokes you tell to make me smile.From the bottom of my heart, thank you to everyone who contributed to this dissertation.xviii
1.0INTRODUCTIONCoding constitutes one of the fundamental functions in the field of Health InformationManagement (HIM) (AHIMA, 2016). Coding can be defined as “the process of translatingdescriptions of diseases, injuries, and procedures into numeric or alphanumeric designations”(AHIMA, 2013). In this era of electronic health records (EHRs) and based on the need forelectronic transactions, coders need not only to be familiar with the code assignment process butalso with mapping among different clinical nomenclature and terminology (DeAlmeida, 2012;Giannangelo, 2011; Alakrawi, 2016).Clinical coders, at least, should have the knowledge and skills that are needed to deal withthe Health Insurance Portability and Accountability Act (HIPAA) code sets. HIPAA standard codesets include the following: Inte
of health care research that addresses clinical data quality due to its direct impact on individual health outcomes as well as population health. This dissertation research is aimed at identifying . AHIMA's Data Quality Management DQM Model. 23 2.1.5.1 AHIMA's DQM Model .