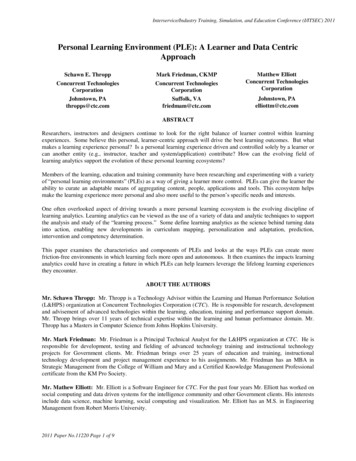
Transcription
Interservice/Industry Training, Simulation, and Education Conference (I/ITSEC) 2011Personal Learning Environment (PLE): A Learner and Data CentricApproachSchawn E. ThroppMark Friedman, CKMPConcurrent TechnologiesCorporationJohnstown, PAthropps@ctc.comConcurrent TechnologiesCorporationSuffolk, VAfriedmam@ctc.comMatthew ElliottConcurrent TechnologiesCorporationJohnstown, PAelliottm@ctc.comABSTRACTResearchers, instructors and designers continue to look for the right balance of learner control within learningexperiences. Some believe this personal, learner-centric approach will drive the best learning outcomes. But whatmakes a learning experience personal? Is a personal learning experience driven and controlled solely by a learner orcan another entity (e.g., instructor, teacher and system/application) contribute? How can the evolving field oflearning analytics support the evolution of these personal learning ecosystems?Members of the learning, education and training community have been researching and experimenting with a varietyof ―personal learning environments‖ (PLEs) as a way of giving a learner more control. PLEs can give the learner theability to curate an adaptable means of aggregating content, people, applications and tools. This ecosystem helpsmake the learning experience more personal and also more useful to the person’s specific needs and interests.One often overlooked aspect of driving towards a more personal learning ecosystem is the evolving discipline oflearning analytics. Learning analytics can be viewed as the use of a variety of data and analytic techniques to supportthe analysis and study of the ―learning process.‖ Some define learning analytics as the science behind turning datainto action, enabling new developments in curriculum mapping, personalization and adaptation, prediction,intervention and competency determination.This paper examines the characteristics and components of PLEs and looks at the ways PLEs can create morefriction-free environments in which learning feels more open and autonomous. It then examines the impacts learninganalytics could have in creating a future in which PLEs can help learners leverage the lifelong learning experiencesthey encounter.ABOUT THE AUTHORSMr. Schawn Thropp: Mr. Thropp is a Technology Advisor within the Learning and Human Performance Solution(L&HPS) organization at Concurrent Technologies Corporation (CTC). He is responsible for research, developmentand advisement of advanced technologies within the learning, education, training and performance support domain.Mr. Thropp brings over 11 years of technical expertise within the learning and human performance domain. Mr.Thropp has a Masters in Computer Science from Johns Hopkins University.Mr. Mark Friedman: Mr. Friedman is a Principal Technical Analyst for the L&HPS organization at CTC. He isresponsible for development, testing and fielding of advanced technology training and instructional technologyprojects for Government clients. Mr. Friedman brings over 25 years of education and training, instructionaltechnology development and project management experience to his assignments. Mr. Friedman has an MBA inStrategic Management from the College of William and Mary and a Certified Knowledge Management Professionalcertificate from the KM Pro Society.Mr. Mathew Elliott: Mr. Elliott is a Software Engineer for CTC. For the past four years Mr. Elliott has worked onsocial computing and data driven systems for the intelligence community and other Government clients. His interestsinclude data science, machine learning, social computing and visualization. Mr. Elliott has an M.S. in EngineeringManagement from Robert Morris University.2011 Paper No.11220 Page 1 of 9
Interservice/Industry Training, Simulation, and Education Conference (I/ITSEC) 2011Personal Learning Environment (PLE): A Learner and Data CentricApproachSchawn E. ThroppMark Friedman, CKMPConcurrent TechnologiesCorporationJohnstown, PAthropps@ctc.comConcurrent TechnologiesCorporationSuffolk, VAfriedmam@ctc.comMatthew ElliottConcurrent TechnologiesCorporationJohnstown, PAelliottm@ctc.comINTRODUCTIONOne highly significant question/challenge we face in thee-learning industry is how to make the learningexperience more personal and engaging—so personaland engaging that maybe the learners do not even knowthey are taking part in a learning experience. The goalis learning that ―just happens‖—the learning experienceis integrated within our daily lives and our personalday-to-day workflow. For example, one aspect of beingpersonal and engaging is the loneliness factor, whichlearning theorists sometimes explore as a question ofsocial presence (Picciano, 2002) (Anderson, Rourke,Garrison, Archer 2001). How many times have youexperienced a typical ―online learning activity‖ whereyou felt lonely—no one to talk to, no one to askquestions of, no one to praise you when you dosomething well or tap you on the shoulder and offerhelp when you are not doing so well? Learningmanagement systems and other niche learning solutionsprovide capabilities such as discussion forums, wikis,and blogs to assist with the feeling of less isolationduring a learning experience. But do such tools reallyhelp? Or are these just advancements in technology thatare finally being used and/or making their way into alearning experience?Advancements in intelligent agents, intelligent tutoringsystems, semantics and learning analytics are promisingto bring a set of solutions that could be tailored togetherto make a true personal learning environment. Aconceptual environment:1.2.3.in which the learner is in control,that is personalized based on a variety of facetsto stimulate the learning process; anddriven by the intelligent analysis of a variety ofdata sources.The intersection of these concepts begins to define thetraits, characteristics, requirements and needs to build asustainable framework for a personal learningecosystem.2011 Paper No.11220 Page 2 of 9A key sustainable and adaptable feature of thisecosystem is the personal learning assistant. Thesepersonal learning assistants are transparent to thelearner and work on behalf of the learner within theecosystem. They gather bits of information left behindby the learner in everyday activities – regardless ofwhether those activities are traditionally considered tobe learning activities. This would include informationabout usage of tools and systems such as, but notlimited to: discussion forum participationsocial media activityapplication or operational system plicationssearch history.In order to define a personal learning ecosystem, aspictured in Figure 1, in which facets of personallearning, personalized learning and learning analyticsare integrated to form a series of thriving, adaptable andintelligent personal learning assistant(s), we believe twovery important topics have to be researched and studied:the learner (learner-centric) and learning analytics (datacentric). This paper focuses on these two topics tobegin to lay a foundation for a flexible and adaptablepersonal learning ecosystem managed and maintainedby a series of transparent personal learning assistants.
Interservice/Industry Training, Simulation, and Education Conference (I/ITSEC) 2011 Can communicate their knowledge in realsettings.A learner-centric approach ultimately leads to a morepersonal or personalized learning experience. Howeverwhich one is it? Personal or personalized, or is it both?Personal vs. PersonalizedWhat is the difference between personal learning andpersonalized learning? One of the best distinctions ourresearch found was by University of Florida professorWendy Drexler. Drexler basically argues that thedistinction is as follows: Figure 1: Conceptual Personal Learning AssistantTraits Intersection THE LEARNER―We are by nature observers, and thereby learners. Thatis our permanent state.‖ – Ralph Waldo EmersonWhat is a learner-centric approach? Researchers andtheorists have described a learner-centric approach asone in which: The focus is on student learning rather thanwhat the teacher is doing (Blumberg, 2008).The role of the trainer changes from being thesource of all knowledge, instead the trainer isseen as a facilitator or guide to the learningprocess (McDaniel, Brown, 2001).Why put the learner first? There are obvious answers tothat question. If we put the learner first–or in thecenter–they will be more engaged with the material,understand the meaning of the material vs. justmemorizing the material and apply concepts learnt moreeffectively (Blumberg, 2008).Blumberg (2004) goes on to describe that when using alearner-centric approach, students tend to: Know why they need to learn and how to learnHave self awareness of their learning abilitiesand their processes of gaining itAre responsible and are prepared to becomeinformed citizensCan retrieve and evaluate informationUse knowledge to solve problems2011 Paper No.11220 Page 3 of 9Personal learning is learning in which thelearner controls the learning process,environment, resource and people they interactwith (Drexler, 2010).Personalized learning is learning in which thelearning process, environment, resources andpeople the learner interacts with are controlled(or makes decisions for the learner based onhis/her past choices or experiences) by someother entity (teacher, tutor, softwareapplication) for the learner (Drexler, 2010).Taking a deeper look at each of these terms begins toreveal some differences. Personalized learning isprobably the most often used of the terms. Personalizedcan mean many things to many people and in most casesthese distinct meanings are correct – that is what makessomething personalized. For example: During the learning process, a system orapplication determines that content should betailored to meet the learner’s progress. If atopic is determined to be challenging to thelearner, perhaps additional ancillary content isprovided (e.g., based on a pre-test,introductory knowledge check, or even anadvanced learning analytics capability).Options are presented to the learner to changethe modality in which the content is presented(e.g., video, all text, immersive, game play,etc), enabling the learner to choose how theywould prefer to see the content.In a personal learning model, the learner is in the centerand controls the process, environment, resources andpeople they interact with. In these cases there are stillinstructional aspects (e.g., learning objectives, teacher)to the process; however, these tend to be more in afacilitation or guiding manner. For example:
Interservice/Industry Training, Simulation, and Education Conference (I/ITSEC) 2011 During the learning process, the learner ispresented with a set of suggested readings toreview around a given topic. The learnerchooses which resource or resources they willreview.The learner is introduced to a set of peers andthey elect which peers they gravitate towards.For example, the learner may decided tointeract with another learner (or peer) becauseof a common research interest or arecommendation by their personal learningassistant.In both cases, personalized and personal, each hasfacets that are important in building a richer learnerexperience. More importantly, these facets can bemodeled within a set of personal learning assistants.Current Research and ApplicabilityOne form of a personal learning environment that israpidly gaining traction (both in the research communityand in operational use) is the concept of a massivelyopen online course (MOOC). In MOOCs studentschoose their preferred mode of learning. Some preferusing a traditional approach based on readingassignments, while others utilize RSS readers and othersstill prefer synchronous dialogue time with theinstructors (usually entering their own commentary intothe dialogue via text chat). Most often, MOOCs areoperated without cost to the student, so as to remain asopen as possible; however now there are least a halfdozen of these well publicized experiments (see Table1) and in the future, there could easily be moretraditional, pay-to-participate, graded-type MOOCsavailable.Allowing each learner to utilize their preferred channelof communication is very interesting and appears to bethe most open and collaborative example of an onlinecourse.This focus on the learner’s preferredcommunication channel places a personal touch on thelearning experience, enabling the learner to choosemany aspects of the experience.In conclusion, based on our research and findings, webelieve that both aspects of personalized learning andpersonal learning need to be utilized in a personallearning ecosystem.These facets will form thefoundation of making the learning experience personal,which then can be designed and modeled into a personallearning assistant. More research is needed in definingthe characteristics and facets of both personalized andpersonal learning experiences. As researchers we needto understand what works and is effective so that we canbuild a better understanding of a richer personalexperience for the learner.LEARNING ANALYTICSLearning analytics is a fast and growing field of study.Interest continues to rise in how to measure and accountfor training and knowledge acquisition. We believe thatlearning analytics is the key enabler of a truly personallearning ecosystem in which facets of personal andpersonalized learning come together and create a varietyof personal learning assistants. A learning ecosystemthat uses the data and information ―bread crumbs‖ thatpeople leave behind in their everyday activities can helpthem take advantage of the variety of learningexperiences they encounter throughout their lifetimes.So what is learning analytics?definitions we could find are:Table 1: Sample MOOCs1.MOOCConnectivism and Connective Knowledge WZPersonal Learning Environments Networks andKnowledge 2010http://bit.ly/mH2S4tPersonal Learning Environments for Inquiry in K12http://bit.ly/k2xXJBeduMOOC: Online Learning Today and Tomorrowhttp://bit.ly/itKUd7Learning and Knowledge Analyticshttp://bit.ly/li7dil2011 Paper No.11220 Page 4 of 92.Two of the best―Learning analytics refers to the interpretationof a wide range of data produced by andgathered on behalf of students in order toassess academic progress, predict futureperformance and spot potential issues‖(Johnson, Smith, Willis, Levine, Haywood,2011).―Learning analytics is the use of intelligentdata, learner-produced data, and analysismodels to discover information and socialconnects, and to predict and advise onlearning‖ (Siemens, 2010).As you can see, these two definitions are quite similar(so were others that we found during our research).Most definitions focus on these key characteristics:
Interservice/Industry Training, Simulation, and Education Conference (I/ITSEC) 2011 Use of a wide set of data (known andunknown)Use of new and emerging analysis modelsPrimarily used for predictions, course of .In the years past, arguably most of the attention on anyform of learning analytics has been focused onassessment data (e.g., end-of-course surveys, knowledgechecks, pre-test/post test). However, there are endlessother sources that are not being tapped and analyzed.Before we take a look at these sources, let us first goback to the roots and take a look at data analytics ingeneral.These are just a few large-scale examples; there havebeen lots of smaller scale data mash ups as well. One tonote was performed by a blogger from the SanFrancisco Bay area (Singh, 2011). The blogger wasinterested in ranking San Francisco Bay area highschools by measuring outcomes such as job titles andcolleges their students attended, instead of how theircurrent rankings are calculated (standardized testscores, class size, student teacher ratio, etc.). Withoutthis information publically available from the schooldistricts, the blogger turned to LinkedIn. UsingLinkedIn data the blogger looked at the top eight highschools in the Bay area and accumulated statistics oncolleges they graduated from, companies they workedfor, their job titles and what industries they worked in.Results of the analysis can be found here:http://bit.ly/kJgBGO.Data Analytics & Data Science – Brief IntroductionThe Data Analysis Process―Data is the next Intel Inside‖ – Tim O’Reilly.Data is ubiquitous; it resides on servers, computers,mobile devices, in data warehouses, databases,fileservers and on paper. Because of computingconstraints, data access and other issues, a wealth ofactionable data has been overlooked in the past. DataAnalytics and Data Science is the science behindturning this data into useful and actionable data.Data driven applications are everywhere and wildlypopular. Here are some examples. Netflix – Netflix uses data collected from theirusers to make recommendations on whatmovies a user might like to watch. Movies arecategorized, and users vote on movies theywatch (1 to 5 stars). The Netflixrecommendation engine learns and is able topredict what movie a subscriber might want towatch next.Google – PageRank is probably one of themost successful uses of data ever. PageRankuses the number of links pointing to aparticular web page to determine its rank inGoogle’s search results.Pandora – The Music Genome Project,similar to Netflix, uses user-generated input torefine recommendations for their users. Similarto Netflix, Pandora uses categorized music anduser input (likes and dislikes) to select whattype of song to play for that user’s selectedstation.2011 Paper No.11220 Page 5 of 9Now that we have looked at some examples of dataanalytics, we now shift our focus onto a briefexplanation of the data analysis process. The dataanalysis process typically follows these steps: Obtain –Scrub – Explore – Model – Interpret (Mason, Wiggins,2010).Obtain – The first part of any data analysis effort isobtaining the data. As mentioned before, data iseverywhere, you just have to find it. Recent Open Datainitiatives have opened the door to previouslyunobtainable data sets. Data marketplaces likeInfoChimps are providing infrastructure wherepeople/companies can buy, share and sell formatteddata.Scrub – Scrubbing is the most important step of the dataanalysis process. Scrubbing or cleaning data will paydividends in the long haul. It is much easier and moreproductive to work with clean, formatted data thansomething that looks like a series of poorly formedHTML tags. Information comes in a variety of formsthat need to be sorted out. For example, consider howyou might address the presence of the following term inyour data: tank (water container) vs. tank (M1 Abrams).Each of these terms might all appear in a dataset. It willsave time and money to differentiate, combine andconsolidate the data. Semantic technologies haveadvanced to a point where they can provide a great dealof assistance in making this distinction and classifyingdata. Regular expressions are your friend during thiscritical step.
Interservice/Industry Training, Simulation, and Education Conference (I/ITSEC) 2011Explore – Once the data is clean it is time to explore thepossibilities. During this step, data can be visualizedusing a variety of techniques. This visualization processwill help drive the creation of hypotheses that can betested in future steps. Data can be visualized on maps,charted in histograms, or clustered together in adendrogram using K-means or hierarchical clustering.Open source tools such as Google Maps and Tableauhave made it easy to start visualizing data with littlelearning curve.Model – This is the hypothesis step, used to help predictand interpret. Selecting the right model will directlyimpact the outcome of the analysis.Interpret – Let us go back to our Netflix example, theinterpretation step in Netflix’s recommendation engineis the recommendation itself. The algorithm or modelhas done what it is meant to do, predict an outcome. Atthis point, knowledge of a domain can help to determineif the model is accurate.Learning Analytics – What the Future HoldsThe 2011 Horizon Report predicts that the time foradoption of learning analytics is four to five years out.The report concludes that ―learning analytics promisesto harness the power of advances in data mining,interpretation, and modeling to improve understandingsof teaching and learning and to tailor education toindividual students more effectively.‖ Although thereport focuses in on the education market (K-12, highereducation), the fundamental concepts described in thereport apply across all learning domains.Current Research & Development EffortsThere are several examples of research anddevelopment efforts underway that are looking atleveraging data and data analytics in their solutions.Most of these cases deal with a single data source in thesense that it is data collected within their ownenvironments. These examples are only provided hereto get an understanding of what some in the industry arebuilding in regards to learning analytics.Purdue University has developed a program calledSignals (Purdue University, 2011). The Signals programis a campus-wide program that is integrated within itsBlackboard Vista environment. The Signals program isused to detect early warning signs and identify the needfor potential intervention actions for those students whomay not be performing to the best of their abilities. Theprogram utilizes common data analysis techniques, such2011 Paper No.11220 Page 6 of 9as data mining and predictive modeling to assess a risklevel and signal the learners at the appropriate time.The University of Wollongong has developed anapplication called Social Networks AdaptingPedagogical Practice (SNAPP). SNAPP is anapplication that allows users to visualize the network ofinteractions resulting from discussion forum posts andreplies (University of Wollongong, 2009). SNAPP canbe used to identify patterns of behaviors such as: Who is posting to the forums more frequently– key information brokersWho is replying to postsWhich learners are interacting with whichlearners the mostWhich learners are ―disconnected.‖Knewton is an organization that is building a learningengine that is described as a continuous adaptivelearning platform that assists students in preparing forGraduate Management Admission Test (GMAT), LawSchool Admission Test (LSAT) and Scholastic AptitudeTest (SAT) tests. Knewton advertises that it is―developing the most powerful adaptive learningengine. It is the only platform that offers continuedadaptivity – the ability to customize educational contentto meet the needs of each student on a daily basis(Knewton, 2011).‖ Knewton captures data within itsown environment. This data is used to customizecontent and paths the student may take within theapplication.The Kahn Academy has produced a website that hostsover 2100 educational videos in the fields of math,science, humanities and test preparation (KahnAcademy, 2011). Part of the solution set that the KahnAcademy offers is a rich set of data based on self-pacedexercises performed by the learner. This data is thenmaintained and exploited to help a learner through aspecific knowledge map.Information and datacollected is available to show proficiencies in aparticular subject, time spent viewing videos, time spenton exercises and achievements obtains. The KahnAcademy is another example of a solution that isfocused on data that is collected within its ownenvironment.BRINGING IT ALL TOGETHEROn one hand we have a shift of focus happeningtowards learner-centric approaches, personalization andenabling a more personal approach to learning. On theother hand we have the emergence and maturation of
Interservice/Industry Training, Simulation, and Education Conference (I/ITSEC) 2011data analytics within the learning, education andtraining domains. We believe there is a perfect stormbrewing at the intersection of these fields of study;something that will truly put the learner front and centerand enable the provision of solutions in which thelearners: Are more engaged,Experience an increase in long term retention,Have a self-awareness of their learningcapability, andHave the ability to understand their processesfor obtaining knowledge and skills.So, what are the next steps? What has to be done tocontrol this perfect storm in order to obtain value out ofthe emergence of these fields of study? Based on ourresearch, we believe that the study and development ofa personal learning ecosystem along with the definitionand understanding of personal learning assistants areneeded. Personal Learning Ecosystem FrameworkBecause of the variety of ―learning environments‖ thatexist today, it is hard to define a single learningframework. Arguments can be made that we learn invariety of ways and in a variety of contexts (peer-topeer conversations, LMSs, gaming environments, use ofsocial media applications, etc). Each of these contexts,however, fit into an overall ecosystem.In thisecosystem there is: A mixture of media and content that can beleveraged,A significant number of applications thatlearners can be engaged with,A rich set of untapped data sources to assistwith personalizing and engaging the learner,andA fundamental infrastructure to deal withcommunication, security and data storage.This ecosystem has to define a set of parameters,conditions of use, policies and practices forcontent/media, learners, solutions providers, data and arich set of applications.We believe that the following components of theecosystem will need to continue to be studied,researched and developed: Open Application Programming Interfaces(APIs) – continued development of open APIs2011 Paper No.11220 Page 7 of 9enablingopencommunicationacrossapplications and accessing the rich data thatexists in these applicationsData Storage – techniques for easily storing,maintaining and accessing portable datastorage (e.g., private data clouds)Anytime Access – ability to gain access todata, applications and information in theecosystem anytime through any means (e.g.,PC, mobile device, Internet of Things)Communication Protocols – with theidentification and definition of open APIs, onealso needs to utilize communication protocolsthat are transparent and easy to useData Framework – with the rich set of databeing left behind, do we need to look atdefining data sets or data definitions? Orshould we look at more self-describing datasets where the entity accessing the dataunderstands ahead of time the types ofinformation they are gaining access to?Variability of Content and Context –support for the variability of content and mediain use today and the future needs to besupported along with the variety of contexts inwhich this content is used.One thing that must be done within this ecosystem is toutilize advancements in these components that aretaking place in other domains. There is no need toreinvent the wheel and focus should be on leveragingthe advancements already made.Personal Learning AssistantsThe concept of a personal learning assistant is not new.Even the activities that one might imagine a personallearning assistant would perform are not new. Solutionsthat utilize personalization techniques and intelligenttutors exist today. So what is different? What haschanged? We believe the driving factor for what iscausing this change is the abundance of data and, moreimportantly, the use of this data.In its simplest form a typical intelligent tutoring system(ITS) contains three primary components: an expertmodel, a student model and a tutor model. Thesemodels interact with each other to help guide or tutorthe learner throughout a learning experience. Thechallenge in any ITS solution is building these modelsup front. What if the models were built on the fly, usingthe information and data gathered through the lifetimeof the learner used to assist the learner through any andall experiences? We believe that is the difference.
Interservice/Industry Training, Simulation, and Education Conference (I/ITSEC) 2011Then what is a personal learning assistant? Whatcharacteristics does one have? What are its roles andresponsibilities? These are the types of questions thatneed to continue to be researched and developed. Webelieve that ultimately a set of characteristics or traitscan be identified. These characteristics and traits willthen lead to the development of a set of personallearning assistants, eventually leading to a taxonomy ofpersonal learning assistants that can be intermixed andused collaboratively within a personal learningecosystem.In order to be successful and ultimately usable, thesepersonal learning assistants should focus on one andonly one role or responsibility. This is the keep it smalland focused philosophy – do not define monolithic andhuge assistants that do everything. In this manner thepersonal learning assistants then can be easily reused inother contexts. Roles and responsibilities might includethings like: Monitor and gather data from a variety ofsourcesClean data that has been gathered and providethe ability to supply that data in a variety offormatsModel data that is gathered to assist in thedevelopment of prediction models, coursecorrection models, recommendation models,etc.Assist in answering questions throughout thelearning processProvide alternative paths or adapt the pathduring a learning experience to challenge andengage the learner’s sense making skillsCoach, mentor and provide positive feedbackto the learner throughout learning experience.The vision here is that your personal learning ecosystemwould have multiple personal learning assistants allworking in harmony with each other to deliver a trueengaging and personal experience.CONCLUSIONSAs seen throughout this paper, the learning, educationand training domain continues to make strides towardlearner-centric approaches.In the mean time,instructors and designe
Johnstown, PA Suffolk, VA Johnstown, PA thropps@ctc.com friedmam@ctc.com elliottm@ctc.com ABSTRACT Researchers, instructors and designers continue to look for the right balance of learner control within learning experiences. Some believe this personal, learner-centric approach will drive the best learning outcomes. But what