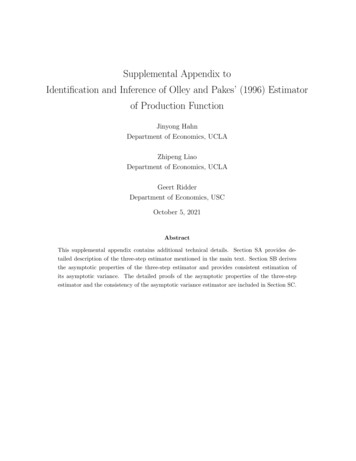
Transcription
Supplemental Appendix toIdentification and Inference of Olley and Pakes’ (1996) Estimatorof Production FunctionJinyong HahnDepartment of Economics, UCLAZhipeng LiaoDepartment of Economics, UCLAGeert RidderDepartment of Economics, USCOctober 5, 2021AbstractThis supplemental appendix contains additional technical details. Section SA provides detailed description of the three-step estimator mentioned in the main text. Section SB derivesthe asymptotic properties of the three-step estimator and provides consistent estimation ofits asymptotic variance. The detailed proofs of the asymptotic properties of the three-stepestimator and the consistency of the asymptotic variance estimator are included in Section SC.
SAThe Three-step Series EstimatorIn this section, we describe the three-step procedure on estimating βk,0 . The model can berewritten asy1,i l1,i βl,0 φ (i1,i , k1,i ) η1,i , y2,i k2,i βk,0 g(ω1,i ) u2,i , y l βwhere y2,i2,i2,i l,0 and ω1,i φ (i1,i , k1,i ) k1,i βk,0 . The following restrictions are maintainedthroughout the appendixE [ η1,i i1,i , k1,i ] 0andE [ u2,i i1,i , k1,i ] 0.(SA.1)For any βk , letω1,i (βk ) φ (i1,i , k1,i ) k1,i βkand g(ω1,i (βk ); βk ) E[y2,i βk k2,i ω1,i (βk )].(SA.2)Then ω1,i ω1,i (βk,0 ) and g(ω1,i ) g(ω1,i (βk,0 ); βk,0 ) by definition. The unknown parameters areβl,0 , βk,0 , φ(·) and g(·; βk ) for any βk in Θk , where Θk is a compact subset of R which contains βk,0as an interior point.Suppose that we have data {(yt,i , it,i , kt,i , lt,i )t 1,2 }ni 1 and a preliminary estimator β̂ l of βl,0 .The asymptotic theory established here allows for a generic estimator of βl,0 , as long as certainregularity conditions (i.e., Assumptions SC1(iii) and SC4(i) in Section SC) hold. For example, β̂ lmay be obtained from the partially linear regression proposed in Olley and Pakes (1996), or fromthe GMM estimation proposed in Ackerberg, Caves, and Frazer (2015). The unknown parametersβk,0 , φ(·) and g(·; βk ) for any βk Θk are estimated through the following three-step estimationprocedure.Step 1. Estimating φ(·). Let P1 (x1,i ) (p1,1 (x1,i ), . . . , p1,m1 (x1,i ))0 be an m1 -dimensionalapproximating functions of x1,i where x1,i (i1,i , k1,i ). Define ŷ1,i y1,i l1,i β̂ l . Then theunknown function φ(·) is estimated byφ̂ (·) P1 (·)0 (P01 P1 ) 1 (P01 Ŷ1 )(SA.3)where P1 (P1 (x1,1 ), . . . , P1 (x1,n ))0 and Ŷ1 (ŷ1,1 , . . . , ŷ1,n )0 .Step 2. Estimating g(·; βk ) for any βk Θk . With β̂ l and φ̂(·) obtained in the first step, one by ŷ ycan estimate y2,i2,i β̂ l l2,i and estimate ω1,i (βk ) by ω̂ 1,i (βk ) φ̂(x1,i ) βk k1,i . Let2,iP2 (ω) (p2,1 (ω), . . . , p2,m2 (ω))0 be an m2 -dimensional approximating functions. Then g(·; βk ) is2
estimated byĝ(·; βk ) P2 (·)0 β̂ g (βk ), where β̂ g (βk ) (P̂2 (βk )0 P̂2 (βk )) 1 P̂2 (βk )0 Ŷ2 (βk )(SA.4) β k , . . . , ŷ β k0where P̂2 (βk ) (P2 (ω̂ 1,1 (βk )), . . . , P2 (ω̂ 1,n (βk )))0 and Ŷ2 (βk ) (ŷ2,1k 2,1k 2,n ) .2,nStep 3. Estimating βk,0 . The finite dimensional parameter βk,0 is estimated by β̂ k throughthe following semiparametric nonlinear regressionβ̂ k arg min nβk Θk 1nX τ̂ i (βk )2 , where τ̂ i (βk ) ŷ2,i k2,i βk ĝ(ω̂ 1,i (βk ); βk ).(SA.5)i 1We shall derive the root-n normality of β̂ k and provide asymptotically valid inference for βk,0 .SBAsymptotic Properties of β̂ kIn this section, we derive the asymptotic properties of β̂ k . The consistency and the asymptoticdistribution of β̂ k are presented in Subsection SB.1. In Subsection SB.2, we provide a consistentestimator of the asymptotic variance of β̂ k which can be used to construct confidence interval forβk,0 . Proofs of the consistency and the asymptotic normality of β̂ k , and the consistency of thestandard deviation estimator are included in Subsection SB.3.SB.1Consistency and asymptotic normalityTo show the consistency of β̂ k , we use the standard arguments for showing the consistency ofthe extremum estimator which requires two primitive conditions: (i) the identification uniquenesscondition of the unknown parameter βk,0 ; and (ii) the convergence of the estimation criterionPfunction n 1 ni 1 τ̂ i (βk )2 to the population criterion function uniformly over βk Θk . We imposethe identification uniqueness condition of βk,0 in condition (SB.6) below, which can be verifiedunder low-level sufficient conditions. The uniform convergence of the estimation criterion functionis proved in Lemma SB1 in Subsection SB.3.Lemma SB1. Let τi (βk ) y2,i l2,i βl,0 βk k2,i g(ω1,i (βk ); βk ) for any βk Θk . Suppose thatfor any ε 0, there exists a constant δε 0 such thatinf{βk Θk : βk βk,0 ε} E τi (βk )2 τi (βk,0 )2 δε .Then under Assumptions SC1 and SC2 in Section SC, we have β̂ k βk,0 op (1).3(SB.6)
The asymptotic normality of β̂ k can be derived from its first-order condition:n 1nXi 1 ĝ(ω̂ 1,i (β̂ k ); β̂ k )τ̂ i (β̂ k ) k2,i βk! 0(SB.7)where for any βk Θk β̂ g (βk ) ĝ(ω̂ 1,i (βk ); βk ) P2 (ω̂ 1,i (βk )) β̂ g (βk )0 P2 (ω̂ 1,i (βk ))0. βk βk βk(SB.8)By the definition of ĝ(ω̂ 1,i (β̂ k ); β̂ k ) in (SA.4), we can writen 1nXnXP2 (ω̂ 1,i (β̂ k ))ĝ(ω̂ 1,i (β̂ k ); β̂ k ) n 1i 1 P2 (ω̂ 1,i (β̂ k ))(ŷ2,i k2,i β̂ k )i 1which implies thatn 1nXτ̂ i (β̂ k )P2 (ω̂ 1,i (β̂ k )) 0.i 1Therefore, the first-order condition (SB.7) can be reduced ton 1nX0 P2 (ω̂ 1,i (β̂ k ))τ̂ i (β̂ k ) k2,i k1,i β̂ g (β̂ k )i 1 ω! 0(SB.9)which slightly simplifies the derivation of the asymptotic normality of β̂ k .Theorem SB1. Let g1 (ω) g(ω)/ ω. Suppose thathiΥ E (v2,i v1,i g1 (ω1,i ))2 0(SB.10)where vj,i kj,i E[kj,i ω1,i ] for j 1, 2. Then under (SB.6) in Lemma SB1, and AssumptionsSC1, SC2 and SC3 in Section SCn1/2 (β̂ k βk,0 ) Υ 1 n 1/2nXu2,i (v2,i v1,i g1 (ω1,i ))i 1nX Υ 1 n 1/2η1,i g1 (ω1,i ) v2,i v1,i g1 (ω1,i )i 1 11/2 Υ Γn (β̂ l βl,0 ) op (1),(SB.11)h i v g (ω ) E[k x ] E[k ω ]. Moreoverwhere Γ E (l2,i l1,i g1 (ω1,i )) v2,iand v2,i1,i 1 1,i2,i 1,i2,i 1,in1/2 (β̂ k βk,0 ) d N (0, Υ 1 ΩΥ 1 )4(SB.12)
where Ω E 2 v g (ω ) Γεu2,i (v2,i v1,i g1 (ω1,i )) η1,i g1 (ω1,i ) v2,i.1,i 1 1,i1,iRemark. The local identification condition of βk,0 is imposed in (SB.10) which is important toensure the root-n consistency of β̂ k . Remark. The random variable ε1,i in the definition of Ω is from the linear representation of theestimator errorβ̂ l βl,0 n 1nXε1,i op (n 1/2 )i 1which is maintained in Assumption SC1(iii) in Section SC. Different estimation procedures of β̂ lmay give different forms for ε1,i . Therefore, the specific form of ε1,i has to be derived case by case. Remark. Since E[vj,i ω1,i ] 0 for j 1, 2,E [l2,i (v2,i v1,i g1 (ω1,i ))] E [(l2,i E[l2,i ω1,i ]) (v2,i v1,i g1 (ω1,i ))] .Therefore we can writeΓ E [(l2,i E[l2,i ω1,i ] h(x1,i )g1 (ω1,i )) (v2,i v1,i g1 (ω1,i ))] .(SB.13)which is the form used in the main text of the paper. Moreover when the perpetual inventorymethod (PIM) i.e., k2,i (1 δ) k1,i i1,i holds, v1,i , v2,i and ω1,i are functions of x1,i . ThereforeE [h(x1,i )g1 (ω1,i ) (v2,i v1,i g1 (ω1,i ))] E [l1,i g1 (ω1,i ) (v2,i v1,i g1 (ω1,i ))]by the law of iterated expectation. Hence we deduce thatΓ E [(l2,i l1,i g1 (ω1,i )) (v2,i v1,i g1 (ω1,i ))]under PIM.(SB.14) Remark. From the asymptotic expansion in (SB.11), we see that the asymptotic variance of β̂ k isPdetermined by three components. The first component, n 1/2 ni 1 u2,i (v2,i v1,i g1 (ω1,i )) comesfrom the third-step estimation with known ω1,i . The second and the third components are fromPthe first-step estimation. Specifically, the second one, n 1/2 ni 1 η1,i g1 (ω1,i ) (v2,i v1,i g1 (ω1,i )) isfrom estimating φ(·) in the first step, while the third component Γn1/2 (β̂ l βl,0 ) is due to theestimation error in β̂ l . 5
Remark. The estimator β̂ k depends on the numbers of approximating functions, i.e., m1 and m2used in estimating φ(·) and g(·, ). In practice, one may select m1 and m2 in a data-dependent way,such as through the cross-validation. The cross-validated series nonparametric regression estimatoris shown to be asymptotically optimal in the literature (see, e.g., Li (1987) and Andrews (1991a)).Therefore, we expect that the estimator β̂ k based on the cross-validated m1 and m2 enjoys goodasymptotic properties such as the root-n asymptotic normality in (SB1). A formal justification ofthis conjecture will be an interesting research topic but is beyond the scope of this paper.SB.2 Consistent variance estimationThe asymptotic variance of β̂ k can be estimated using its explicit form and the estimators , h(x ) and g (ω ). The unknown functions in v , v , ε , η ,of v1,i , v2,i , ε1,i , η1,i , u2,i , v2,i1,i1 1,i1,i2,i1,i1,i , h(x ) and g (ω ) can be estimated by the kernel or the series method. Since β̂ isu2,i , v2,i1,i1 1,ikconstructed using the series method and its asymptotic properties have been established in theprevious subsection, we next provide the asymptotic variance estimator of β̂ k using the seriesmethod.First, it is clear that g1 (ω1,i ) can be estimated by ĝ1 (ω̂ 1,i (β̂ k ); β̂ k ) whereĝ1 (ω̂ 1,i (βk ); βk ) β̂ g (βk )0 P2 (ω̂ 1,i (βk ))for any βk Θk . ω(SB.15)Second, the residual ςi v2,i v1,i g1 (ω1,i ) can be estimated byς̂ i k̂2,i P2 (ω̂ 1,i (β̂ k ))0 (P̂2 (β̂ k )0 P̂2 (β̂ k )) 1nXP2 (ω̂ 1,i (β̂ k )) k̂2,ii 1where k̂2,i k2,i k1,i ĝ1 (ω̂ 1,i (β̂ k ); β̂ k ).Given the estimated residual ς̂ i , the Hessian term Υ in the asymptotic variance of β̂ k can beestimated by 1nXς̂ 2i .(SB.16)(l2,i ĥi ĝ1 (ω̂ 1,i (β̂ k ); β̂ k ))ς̂ i(SB.17)Υ̂n ni 1Moreover the Jacobian term Γ can be estimated byΓ̂n n 1nXi 1where ĥi P1 (x1,i )0 (P01 P1 ) 1Pni 1 P1 (x1,i )l1,i .Defineû2,i ŷ2,i l2,i β̂ l k2,i β̂ k ĝ(ω̂ 1,i (β̂ k ); β̂ k )6andη̂ 1,i y1,i l1,i β̂ l φ̂(x1,i ).
Then Ω is estimated byΩ̂n n 1n X(û2,i η̂ 1,i ĝ1 (ω̂ 1,i (β̂ k ); β̂ k ))ς̂ i Γ̂n ε̂1,i 2(SB.18)i 1where ε̂1,i denotes the estimator of ε1,i for i 1, . . . , n.Theorem SB2. Under Assumptions SC1, SC2, SC3 and SC4 in Section SC, we haveΥ̂n Υ op (1)and moreoverandn1/2 (β̂ k βk,0 ) 1 1/2(Υ̂ 1n Ω̂n Υ̂n )Ω̂n Ω op (1) d N (0, 1)(SB.19)(SB.20)where Ω̂n is defined in (SB.18).SB.3Proof of the asymptotic propertiesIn this subsection, we prove the main results presented in the previous subsection. Throughoutthis subsection, we use C 1 to denote a generic finite constant which does not depend on n, m1or m2 but whose value may change in different places.Proof of Lemma SB1. By (SC.72) in the proof of Lemma SC8 and Assumption SC2(i) sup E τi (βk )2 C(SB.21)βk Θkwhich together with Lemma SC8 implies thatsup n 1βk ΘknXτi (βk )2 Op (1).(SB.22)i 1By the Markov inequality, Assumptions SC1(i, iii) and SC2(i), we obtainn 1nX 2(ŷ2,i y2,i) (β̂ l βl )2 n 1i 1nXi 172l2,i Op (n 1 ).(SB.23)
By the definition of τ̂ i (βk ) and τi (βk ), we can writenXn 1 τ̂ i (βk )2 E τi (βk )2i 1nX n 1nX τi (βk )2 E τi (βk )2 2n 1τi (βk )(ŷ2,i y2,i)i 1 2n 1i 1nXτi (βk )(ĝ(ω̂ 1,i (βk ); βk ) g(ω1,i (βk ); βk ))i 1 2n 1nX (ŷ2,i y2,i)(ĝ(ω̂ 1,i (βk ); βk ) g(ω1,i (βk ); βk ))i 1 n 1nX 2(ŷ2,i y2,i) n 1i 1nX(ĝ(ω̂ 1,i (βk ); βk ) g(ω1,i (βk ); βk ))2 ,i 1which together with Assumption SC2(vi), Lemma SC7, Lemma SC8, (SB.22), (SB.23) and theCauchy-Schwarz inequality implies thatsup n 1βk ΘknX τ̂ i (βk )2 E τi (βk )2 op (1).(SB.24)i 1The consistency of β̂ k follows from its definition in (SA.5), (SB.24), the identification uniquenesscondition of βk,0 assumed in (SB.6) and the standard arguments of showing the consistency of theextremum estimator.Q.E.D.Lemma SB2. Let g1,i g1 (ω1,i ) and Jˆi (βk ) τ̂ i (βk ) (k2,i k1,i ĝ1 (ω̂ 1,i (βk ); βk )) for any βk Θk ,where ĝ1 (ω̂ 1,i (βk ); βk ) is defined in (SB.15). Then under Assumptions SC1, SC2 and SC3, we haven 1nXJˆi (βk,0 ) n 1i 1nX(u2,i η1,i g1,i )(v2,i v1,i g1,i ) Γ(β̂ l βl,0 ) op (n 1/2 ).(SB.25)i 1Proof of Lemma SB2. By the definition of τ̂ i (βk,0 ) and Lemma SC10,n 1nXτ̂ i (βk,0 ) (k2,i k1,i ĝ1 (ω̂ 1,i (βk,0 ); βk,0 ))i 1 n 1nX (ŷ2,i(βk,0 ) g(ω1,i ))(k2,i k1,i g1,i )i 1 n 1nX(ĝ(ω̂ 1,i (βk,0 ); βk,0 ) g(ω1,i ))(k2,i k1,i g1,i ) op (n 1/2 )i 18(SB.26)
(β ) ywhere ŷ2,i2,i l2,i β̂ l k2,i βk,0 , and by Lemma SC12k,0n 1nX(ĝ(ω̂ 1,i (βk,0 ); βk,0 ) g(ω1,i ))(k2,i k1,i g1,i )i 1 n 1nXu2,i ϕ(ω1,i ) E[l2,i ϕ(ω1,i )](β̂ l βl,0 )i 1nX 1 ng1,i (φ̂(x1,i ) φ(x1,i ))(v2,i v1,i g1,i )i 1 op (n 1/2 ),(SB.27) (β ), we getwhere ϕ(ω1,i ) E[k2,i ω1,i ] E[k1,i ω1,i ]g1,i . By the definition of ŷ2,ik,0n 1nX (ŷ2,i(βk,0 ) g(ω1,i ))(k2,i k1,i g1,i )i 1 n 1nXu2,i (k2,i k1,i g1,i ) (β̂ l βl,0 )n 1i 1 n 1nXnXl2,i (k2,i k1,i g1,i )i 1u2,i (k2,i k1,i g1,i ) (β̂ l βl,0 )E[l2,i (k2,i k1,i g1,i )] op (n 1/2 )(SB.28)i 1where the second equality is by Assumption SC1(iii) andn 1nXl2,i (k2,i k1,i g1,i ) E[l2,i (k2,i k1,i g1,i )] Op (n 1/2 )i 1which holds by the Markov inequality, Assumptions SC1(i) and SC2(i, ii). Therefore by (SB.26),(SB.27) and (SB.28), we obtainn 1 n 1nXτ̂ i (βk,0 ) (k2,i k1,i ĝ1 (ω̂ 1,i (βk,0 ); βk,0 ))i 1nXu2,i (v2,i v1,i g1,i ) (β̂ l βl,0 )E[l2,i (v2,i v1,i g1,i )]i 1nX n 1g1,i (φ̂(x1,i ) φ(x1,i ))(v2,i v1,i g1,i ) op (n 1/2 ).i 1The claim of the lemma follows from (SB.29) and Lemma SC13.Lemma SB3. Under Assumptions SC1, SC2 and SC3, we haven 1nX (Jˆi (β̂ k ) Jˆi (βk,0 )) (β̂ k βk,0 ) E[(v2,i v1,i g1,i )2 ] op (1) op (n 1/2 ).i 19(SB.29)Q.E.D.
Proof of Lemma SB3. First note that by the definition of Jˆi (βk ) and τ̂ i (βk ), we can writen 1nX(Jˆi (β̂ k ) Jˆi (βk,0 ))i 1 (β̂ k βk,0 )n 1nXk2,i (k2,i k1,i ĝ1 (ω̂ 1,i (β̂ k ); β̂ k ))i 1 n 1nX(ĝ(ω̂ 1,i (β̂ k ); β̂ k ) ĝ(ω̂ 1,i (βk,0 ); βk,0 ))(k2,i k1,i ĝ1 (ω̂ 1,i (βk,0 ); βk,0 ))i 1 n 1nXu2,i k1,i (ĝ1 (ω̂ 1,i (β̂ k ); β̂ k ) ĝ1 (ω̂ 1,i (βk,0 ); βk,0 ))i 1 (β̂ l βl,0 )n 1nXl2,i k1,i (ĝ1 (ω̂ 1,i (β̂ k ); β̂ k ) ĝ1 (ω̂ 1,i (βk,0 ); βk,0 ))(SB.30)i 1which together with Assumption SC1(iii), Lemma SC17, Lemma SC21 and Lemma SC23 impliesthatn 1nX(Jˆi (β̂ k ) Jˆi (βk,0 )) (β̂ k βk,0 )E[k2,i (k2,i k1,i g1,i )]i 1 (β̂ k βk,0 ) [E[k1,i g1,i (v2,i v1,i g1,i )] E[k2,i ϕ(ω1,i )]] (β̂ k βk,0 )op (1) op (n 1/2 ) (β̂ k βk,0 ) E[(v2,i v1,i g1,i )2 ] op (1) op (n 1/2 )which finishes the proof.Q.E.D.Proof of Theorem SB1. By Assumptions SC1(ii, iii) and SC2(i, ii), and Hölder’s inequalityΓ E [(l2,i hi g1 (ω1,i )) (v2,i v1,i g1 (ω1,i ))] C(SB.31)andΩ E[((u2,i η1,i g1 (ω1,i ))(v2,i v1,i g1 (ω1,i )) Γε1,i )2 ]444 CE[u42,i η1,i v1,i v2,i ε21,i ] C.(SB.32)By Assumption SC1(i), (SB.32) and the Lindeberg–Lévy central limit theorem,n 1/2nX((u2,i η1,i g1 (ω1,i ))(v2,i v1,i g1 (ω1,i )) Γε1,i ) d N (0, Ω).i 110(SB.33)
By (SB.9), Assumption SC1(iii), Lemma SB2 and Lemma SB3, we can write0 n 1 n 1nXi 1nXJˆi (βk,0 ) n 1nX(Jˆi (β̂ k ) Jˆi (βk,0 ))i 1(u2,i η1,i g1 (ω1,i ))(v2,i v1,i g1 (ω1,i )) Γn1/2 (β̂ l βl,0 )i 1 (β̂ k βk,0 ) E[(v2,i v1,i g1 (ω1,i ))2 ] op (1) op (n 1/2 )(SB.34)which together with (SB.10) and (SB.33) implies thatn1/2 (β̂ k βk,0 ) Υ 1 n 1/2nX(u2,i η1,i g1 (ω1,i ))(v2,i v1,i g1 (ω1,i )) Υ 1 Γn1/2 (β̂ l βl,0 ) op (1).i 1(SB.35)This proves (SB.11). The claim in (SB.12) follows from Assumption SC1(iii), (SB.33) and (SB.35).Q.E.D.Proof of Theorem SB2. The results in (SB.19) are proved in Lemma SC25(i, iii), whichtogether with Theorem SB1, Assumption SC4(iii) and the Slutsky Theorem proves the claim in(SB.20).SCQ.E.D.Auxiliary ResultsIn this section, we provide the auxiliary results which are used to show Lemma SB1, TheoremSB1 and Theorem SB2. The following notations are used throughout this section. We use k·k2to denote the L2 -norm under the joint distribution of (yt,i , it,i , kt,i , lt,i )t 1,2 , k·k to denote the Euclidean norm and k·kS to denote the matrix operator norm. For any real symmetric square matrixA, we use λmin (A) and λmax (A) to denote the smallest and largest eigenvalues of A respectively.Throughout this appendix, we use C 1 to denote a generic finite constant which does not dependon n, m1 or m2 but whose value may change in different places.SC.1The asymptotic properties of the first-step estimatorsLet Qm1 E [P1 (x1,i )P1 (x1,i )0 ]. The following assumptions are needed for studying the firststep estimator φ̂(·).Assumption SC1. (i) The data {(yt,i , it,i , kt,i , lt,i )t 1,2 }ni 1 are i.i.d.; (ii) E[ η1,i x1,i ] 0 and2 η 4 x ] C; (iii) there exist i.i.d. random variables ε4E[ l1,i1,i1,i with E[ε1,i ] C such that1,iβ̂ l βl,0 n 1nXi 111ε1,i op (n 1/2 );
(iv) there exist rφ 0 and βφ,m Rm such that supx X φm (x) φ(x) O(m rφ ) whereφm (x) P1 (x)0 βφ,m and X denotes the support of x1,i which is compact; (v) C 1 λmin (Qm1 ) rφλmax (Qm1 ) C uniformly over m1 ; (vi) m21 n 1 n1/2 m12 O(1) and log(m1 )ξ0,mn 1 o(1)1where ξ0,m1 is a nondecreasing sequence such that supx X kP1 (x)k ξ0,m1 .Assumption SC1(iii) assumes that there exists a root-n consistent estimator β̂ l of βl,0 . Differentestimation procedures of β̂ l may give different forms for ε1,i . For example, β̂ l may be obtainedtogether with the nonparametric estimator of φ(·) in the partially linear regression proposed inOlley and Pakes (1996), or from the GMM estimation proposed in Ackerberg, Caves, and Frazer(2015). Therefore, the specific form of ε1,i has to be derived case by case. The rest conditions inAssumption SC1 are fairly standard in series estimation; see, for example, Andrews (1991b), Newey(1997) and Chen (2007).1 In particular, condition (iv) specifies the precision for approximating theunknown function φ (·) via approximating functions, for which comprehensive results are availablefrom numerical approximation theory.The properties of the first-step estimator φ̂(·) are presented in the following lemma.Lemma SC4. Under Assumption SC1, we haven 1nX φ̂(x1,i ) φ(x1,i ) 2 Op (m1 n 1 )(SC.36)i 1and moreover1/2sup φ̂(x1 ) φ(x1 ) Op (ξ0,m1 m1 n 1/2 ).(SC.37)x1 XProof of Lemma SC4. Under Assumption SC1(i, v, vi), we can invoke Lemma 6.2 in Belloni,Chernozhukov, Chetverikov, and Kato (2015) to obtainn 1 P01 P1 Qm1S Op ((log m1 )1/2 ξ0,m1 n 1/2 ) op (1)(SC.38)which together with Assumption SC1(v) implies thatC 1 λmin (n 1 P01 P1 ) λmax (n 1 P01 P1 ) C(SC.39)uniformly over m1 with probability approaching 1 (wpa1). Since ŷ1,i y1,i l1,i β̂ l φ(x1,i ) 1For some approximating functions such as power series, Assumptions SC1(v, vi) hold under certain nonsingulartransformation on the vector approximating functions P1 (·), i.e., BP1 (·), where B is some non-singular constantmatrix. Since the nonparametric series estimator is invariant to any nonsingular transformation of P1 (·), we do notdistinguish between BP1 (·) and P1 (·) throughout this appendix.12
η1,i l1,i (β̂ l βl,0 ), we can writeβ̂ φ βφ,m1 (P01 P1 ) 1nXP1 (x1,i )η1,ii 1nX 1 (P01 P1 )P1 (x1,i )(φ(x1,i ) φm1 (x1,i ))i 1 (β̂ l βl,0 )(P01 P1 ) 1nXP1 (x1,i )l1,i .(SC.40)i 1By Assumption SC1(i, ii, v) and the Markov inequalityn 1nX1/2P1 (x1,i )η1,i Op (m1 n 1/2 )(SC.41)i 1which together with Assumption SC1(vi), (SC.38) and (SC.39) implies thatn 1 0 1 X1/2 1 1P1 (x1,i )η1,i Op ((log m1 )1/2 ξ0,m1 m1 n 1 ) op (n 1/2 ). (SC.42)(n P1 P1 ) Qm1 ni 1By Assumption SC1(iv, vi) and (SC.39)(P01 P1 ) 1nXP1 (x1,i )(φ(x1,i ) φm1 (x1,i )) Op (m rφ ) Op (n 1/2 ).(SC.43)i 1Under Assumption SC1(i, ii, v, vi), we can use similar arguments in showing (SC.41) to getn 1nX1/2P1 (x1,i )l1,i E[P1 (x1,i )l1,i ] Op (m1 n 1/2 ) op (1).(SC.44)i 1By Assumption SC1(i, ii, v),2kE[l1,i P1 (x1,i )]k2 λmax (Qm1 )E[l1,i P1 (x1,i )0 ]Q 1m1 E[P1 (x1,i )l1,i ] CE[l1,i ] C(SC.45)which combined with (SC.44) implies thatn 1nXP1 (x1,i )l1,i Op (1).i 113(SC.46)
By Assumption SC1(iii, v, vi), (SC.38), (SC.44), (SC.45) and (SC.46),(β̂ l βl,0 )(P01 P1 ) 1nX 1/2P1 (x1,i )l1,i Q 1)m1 E[P1 (x1,i )l1,i ](β̂ l βl,0 ) Op (ni 1which combined with Assumption SC1(vi), (SC.40), (SC.42) and (SC.43) shows thatβ̂ φ βφ,m1 Q 1m1nX!1/2 Op (n 1/2 ) Op (m1 n 1/2 )P1 (x1,i )η1,i E[P1 (x1,i )l1,i ](β̂ l βl,0 )i 1(SC.47)where the second equality follows from Assumptions SC1(iii, v), (SC.41) and (SC.45). By theCauchy-Schwarz inequalityn 1nX2 φ̂(x1,i ) φ(x1,i ) 2n 1i 1 2λmax (n 1 P01 P1 ) β̂ φ βφ,m1nX2 φ̂(x1,i ) φm1 (x1,i ) 2ni 12 1nX φm1 (x1,i ) φ(x1,i ) 2i 11/2 2 sup φm1 (x) φ(x) Op (m1 n 1/2 )(SC.48)x X1where the equality is by Assumptions SC1(iv, vi), (SC.39) and (SC.47), which proves (SC.36). Bythe triangle inequality, the Cauchy-Schwarz inequality, Assumption SC1(iv, vi) and (SC.47)sup φ̂(x1 ) φ(x1 ) x1 Xsup φ̂(x1 ) φm1 (x1 ) sup φm1 (x1 ) φ(x1 ) x1 Xx1 X rφ ξ0,m1 β̂ φ βφ,m1 O(m1which proves the claim in (SC.36).SC.21/2) Op (ξ0,m1 m1 n 1/2 ) (SC.49)Q.E.D.Auxiliary results for the consistency of β̂ k β k ω (β ) ω]. For anyRecall that ω1,i (βk ) φ(x1,i ) βk k1,i and g(ω; βk ) E[y2,ik 2,i 1,ikβk Θk , let Ω(βk ) [aβk , bβk ] denote the support of ω1,i (βk ) with cω aβk bβk Cω , wherecω and Cω are finite constants. Define Ωε (βk ) [aβk ε, bβk ε] for any constant ε 0. For aninteger d 0, let g(βk ) d max0 j d supω Ω(βk ) j g(ω; βk )/ ω j . )4 l4 k 4 x ] C; (ii) g(ω; β ) is continuously differentiable withAssumption SC2. (i) E[(y2,ik2,i2,i 1,iuniformly bounded derivatives; (iii) for some d 1 there exist βg,m2 (βk ) Rm2 and rg 0 such rgthat supβk Θk g(βk ) gm2 (βk ) d O(m2) where gm2 (ω; βk ) P2 (ω)0 βg,m2 (βk ); (iv) for anyβk Θk there exists a nonsingular matrix B(βk ) such that for P̃2 (ω1 (βk ); βk ) B(βk )P2 (ω1 (βk )),C 1 λmin (Qm2 (βk )) λmax (Qm2 (βk )) C14
uniformly over βk Θk , where Qm2 (βk ) E[P̃2 (ω1 (βk ); βk ) P̃2 (ω1 (βk ); βk )0 ]; (v) for j 0, 1, 2, 3,there exist sequences ξj,m2 such that supβk Θk supω Ωε (βk ) j P̃2 (ω; βk ) / ω j1 βkj j1 ξj,m2 where rg1/2j 1and ξ0,m1 (m1 m32 (log(n))1/2 )n 1/2 n1/2 m2j1 j and ε m 22 ; (vi) ξj,m2 Cm2 o(1). , lAssumption SC2(i) imposes upper bound on the conditional moments of y2,i2,i and k2,i givenx1,i . Assumptions SC2(ii, iii) require that the conditional moment function g(ω; βk ) is smoothand can be well approximated by linear combinations of P2 (ω). Assumption SC2(iv) imposesnormalization on the approximating functions P2 (ω), and uniform lower and upper bounds onthe eigenvalues of Qm2 (βk ). Assumption SC2(v, vi) restrict the magnitudes of the normalizedapproximating functions and their derivatives, and the convergence rate of the series approximationerror.Since the series estimator ĝ(ω̂ 1,i (βk ); βk ) P2 (ω̂ 1,i (βk ))0 β̂ g (βk ) is invariant to any non-singulartransformation on P2 (ω), throughout the rest of the Appendix we letP̃2 (βk ) (P̃2,1 (βk ), . . . , P̃2,n (βk ))0and P̂2 (βk ) (P̂2,1 (βk ), . . . , P̂2,n (βk ))0where P̃2,i (βk ) B(βk )P2 (ω1,i (βk )), P̂2,i (βk ) B(βk )P2 (ω̂ 1,i (βk )) and ω̂ 1,i (βk ) φ̂(x1,i ) k1,i βk .2Define j P̃2 (ω; βk ) j P̃2 (ω; βk ) ω jand j P̃2,i (βk ) j P̃2 (ω1,i (βk ); βk )for j 1, 2, 3 and i 1, . . . , n.Lemma SC5. Under Assumptions SC1 and SC2, we havesupn 1 P̂2 (βk )0 P̂2 (βk ) n 1 P̃2 (βk )0 P̃2 (βk )βk Θk1/2S Op (ξ1,m2 m1 n 1/2 ).Proof of Lemma SC5. Since ω̂ 1,i (βk ) φ̂(x1,i ) βk k1,i , by Lemma SC41/2sup max ω̂ 1,i (βk ) ω1,i (βk ) max φ̂(x1,i ) φ(x1,i ) Op (ξ0,m1 m1 n 1/2 ) op (1) (SC.50)βk Θki ni nwhich together with Assumption SC2(vi) implies thatω̂ 1,i (βk ) Ωε (βk ) wpa1(SC.51)for any i n and uniformly over βk Θk . By the mean value expansion, we have for any υ2 Rm2υ20 (P̃2,i (βk ) P̂2,i (βk )) υ20 1 P̃2 (ω̃ 1,i (βk ); βk ) (ω̂ 1,i (βk ) ω1,i (βk ))2(SC.52)Note that we define P̂2,i (βk ) P2 (ω̂ 1,i (βk )) in Section SA. We change its definition here since the asymptoticproperties of the sereis estimator ĝ(ω̂ 1,i (βk ); βk ) P2 (ω̂ 1,i (βk ))0 β̂ g (βk ) shall be investigated under the new definitionP̂2,i (βk ) B(βk )P2 (ω̂ 1,i (βk )).15
where ω̃ 1,i (βk ) lies between ω1,i (βk ) and ω̂ 1,i (βk ). Since ω1,i (βk ) and ω̂ 1,i (βk ) are in Ωε (βk ) uniformly over βk Θk and for any i 1, . . . , n wpa1, the same property holds for ω̃ 1,i (βk ). By theCauchy-Schwarz inequality, Assumption SC2(v) and (SC.52)υ20 (P̃2,i (βk ) P̂2,i (βk )) kυ2 k ξ1,m2 φ̂(x1,i ) φ(x1,i ) wpa1.Therefore,υ20 (P̂2 (βk ) P̃2 (βk ))0 (P̂2 (βk ) P̃2 (βk ))υ2 nnXX2(υ20 (P̃2,i (βk ) P̂2,i (βk )))2 kυ2 k2 ξ1,m φ̂(x1,i ) φ(x1,i ) 22i 1i 1wpa1, which together with Lemma SC4 implies that1/2sup P̂2 (βk ) P̃2 (βk ) S Op (ξ1,m2 m1 ).(SC.53)βk ΘkBy Lemma SC27 and Assumption SC2(iv, vi), we have uniformly over βk ΘkC 1 λmin (n 1 P̃2 (βk )0 P̃2 (βk )) λmax (n 1 P̃2 (βk )0 P̃2 (βk )) C wpa1.(SC.54)By the triangle inequality, Assumption SC2(vi), (SC.53) and (SC.54), we getn 1 P̂2 (βk )0 P̂2 (βk ) n 1 P̃2 (βk )0 P̃2 (βk )supβk Θk Ssup n 1 (P̂2 (βk ) P̃2 (βk ))0 (P̂2 (βk ) P̃2 (βk ))βk Θk sup n 1 (P̂2 (βk ) P̃2 (βk ))0 P̃2 (βk )βk Θk sup n 1 P̃2 (βk )0 (P̂2 (βk ) P̃2 (βk ))βk Θkwhich proves the claim of the lemma.SS1/2S Op (ξ1,m2 m1 n 1/2 )Q.E.D.Lemma SC6. Under Assumptions SC1 and SC2, we havesup n 1βk ΘknXP̃2,i (βk )0 β̂ g (βk ) g(ω1,i (βk ); βk )i 1where β̂ g (βk ) (P̂2 (βk )0 P̂2 (βk )) 1 P̂2 (βk )0 Ŷ2 (βk ).1622 Op ((m22 ξ1,mm1 )n 1 ) op (1)2
Proof of Lemma SC6. By the Cauchy-Schwarz inequality and Assumption SC2(iii)n 1 2nnXP̃2,i (βk )0 β̂ g (βk ) g(ω1,i (βk ); βk )i 1nX 1 2nP̃2,i (βk )0 β̂ g (βk ) gm2 (ω1,i (βk ); βk )i 1nX 1i 1 1 2λmax (n22 gm2 (ω1,i (βk ); βk ) g(ω1,i (βk ); βk ) 2 2rgP̃2 (βk )0 P̃2 (βk )) β̂ g (βk ) β̃ g,m2 (βk ) 2 Cm2(SC.55)for any βk Θk , where β̃ g,m2 (βk ) (B(βk )0 ) 1 βg,m2 (βk ) and βg,m2 (βk ) is defined in AssumptionSC2(iii). We next show thatsupβk Θkβ̂ g (βk ) β̃ g,m2 (βk )22 Op ((m22 ξ1,mm1 )n 1 ) op (1)2(SC.56)which together with (SC.54) and (SC.55) proves the claim of the lemma. k β g(ω (β ), β ). Then we can writeLet u2,i (βk ) y2,i2,i k1,i kkβ̂ g (βk ) β̃ g,m2 (βk ) (P̂2 (βk )0 P̂2 (βk )) 1 P̂2 (βk )0 (Ŷ2 (βk ) P̂2 (βk )0 β̃ g,m2 (βk ))nX0 1P̂2,i (βk )(g(ω1,i (βk ), βk ) gm2 (ω̂ 1,i (βk ), βk )) (P̂2 (βk ) P̂2 (βk ))i 10 1 (β̂ l βl,0 )(P̂2 (βk ) P̂2 (βk ))nXP̂2,i (βk )l2,ii 10 1 (P̂2 (βk ) P̂2 (βk ))nXP̂2,i (βk )u2,i (βk )(SC.57)i 1where gm2 (ω̂ 1,i (βk ), βk ) P̂2,i (βk )0 β̃ g,m2 (βk ). By Assumption SC2(vi), Lemma SC5 and (SC.54),we have uniformly over βk ΘkC 1 λmin (n 1 P̂2 (βk )0 P̂2 (βk )) λmax (n 1 P̂2 (βk )0 P̂2 (βk )) C wpa1(SC.58)which implies that P̂2 (βk )(P̂2 (βk )0 P̂2 (βk )) 1 P̂2 (βk )0 is an idempotent matrix uniformly over βk 17
Θk wpa1. Therefore,0 1(P̂2 (βk ) P̂2 (βk ))nX2P̂2,i (βk )(g(ω1,i (βk ), βk ) gm2 (ω̂ 1,i (βk ), βk ))i 1 Op (1)n 1nX(g(ω1,i (βk ), βk ) gm2 (ω̂ 1,i (βk ), βk ))2 .(SC.59)i 1uniformly over βk Θk . Since ω1,i (βk ) φ(x1,i ) k1,i βk , we can use Assumptions SC1(i) andSC2(i) to deducesup g(ω1,i (βk ); βk ) C.(SC.60)βk ΘkTherefore,2supβk Θk β̃ g,m2 (βk )sup (λmin (Qm2 (βk ))) 1 P̃2,i (βk )0 β̃ g,m2 (βk )βk Θk22 C sup kg(ω1,i (βk ); βk ) gm2 (ω1,i (βk ); βk )k22βk Θk C sup kg(ω1,i (βk ); βk )k22 C.(SC.61)βk ΘkBy the second order expansion, Assumption SC2(iii, v, vi), Lemma SC4, (SC.60) and (SC.61), wehave uniformly over βk Θk ,n 1 2nnX(gm2 (ω1,i (βk ), βk ) gm2 (ω̂ 1,i (βk ), βk ))2i 1nX 1 2n( 1 P̃2,i (βk )0 β̃ g,m2 (βk )(φ̂(x1,i ) φ(x1,i ))2i 1nX 1( 2 P̃2 (ω̃ 1,i (βk ); βk )0 β̃ g,m2 (βk )(φ̂(x1,i ) φ(x1,i )2 )2i 12 Op (m1 n 1 ) Op (ξ2,mξ 2 m21 n 2 ) Op (m1 n 1 )2 0,m1where ω̃ 1,i (βk ) is between ω1,i (βk ) and ω̂ 1,i (βk ) and it lies in Ωε (βk ) uniformly over βk Θk wpa118
by (SC.51), which together with Assumption SC2(iii, vi) implies thatn 1nX(g(ω1,i (βk ), βk ) gm2 (ω̂ 1,i (βk ), βk ))2i 1nX 1 Cn(g(ω1,i (βk ), βk ) gm2 (ω1,i (βk ), βk ))2i 1nX 1 Cni 1 1 Op (m1 n(gm2 (ω1,i (βk ), βk ) gm2 (ω̂ 1,i (βk ), βk ))2 2rg m2) Op (m1 n 1 ).(SC.62)From (SC.59) and (SC.62), we get uniformly over βk Θk(P̂2 (βk )0 P̂2 (βk )) 1nX1/2P̂2,i (βk )(g(ω1,i (βk ), βk ) gm2 (ω̂ 1,i (βk ), βk )) Op (m1 n 1/2 ).(SC.63)i 1By Assumptions SC1(i) and SC2(i), and the Markov inequality,n 1nX2l2,i Op (1)(SC.64)i 1which together with Assumption SC1(iii) and (SC.58) implies that(β̂ l βl,0 )(P̂2 (βk )0 P̂2 (βk )) 1nXP̂2,i (βk )l2,i Op (n 1/2 )(SC.65)i 1uniformly over βk Θk . By the mean value expansion, the Cauchy-Schwarz inequality and thetriangle inequality, we have for any υ2 Rm2n 1n 1nXi 1nXυ20 (P̂2,i (βk ) P̃2,i (βk ))u2,i (βk )υ20 1 P̃2 (ω̃ 1,i (βk ); βk ) (ω̂ 1,i (βk ) ω1,i (βk ))u2,i (βk )i 1 kυ2 k ξ1,m2 n 1nX(φ̂(x1,i ) φ(x1,i ))u2,i (βk ) .(SC.66)i 1By the definition of u2,i (βk ), we can use Assumptions SC1(i) and SC2(i), (SC.60) and the Markovinequality to deducesup n 1βk ΘknX(u2,i (βk ))2 Op (1).i 119(SC.67)
Thus by the Cauchy-Schwarz inequality, Lemma SC4 and (SC.67),sup n 1βk ΘknX1/2(φ̂(x1,i ) φ(x1,i ))u2,i (βk ) Op (m1 n 1/2 )i 1which together with (SC.58) and (SC.66) implies that(P̂2 (βk )0 P̂2 (βk )) 1nX1/2(P̂2,i (βk ) P̃2,i (βk ))u2,i (βk ) Op (ξ1,m2 m1 n 1/2 )(SC.68)i 1uniformly over βk Θk . Applying Lemma SC28 and (SC.58) yields(P̂2 (βk )0 P̂2 (βk )) 1nXP̃2,i (βk )u2,i (βk ) Op (m2 n 1/2 )(SC.69)i 1uniformly over βk Θk . The claim in (SC.56) then follows from Assumption SC2(vi), (SC.57),(SC.63), (SC.65), (SC.68) and (SC.69).Q.E.D.Lemma SC7. Under Assumptions SC1 and SC2, we havesup n 1βk ΘknX2 ĝ(ω̂ 1,i (βk ); βk ) g(ω1,i (βk ); βk ) 2 Op ((m22 ξ1,mm1 )n 1 ) op (1).2i 1Proof of Lemma SC7. By the triangle inequality, (SC.56) and (SC.61)supβk
Remark. The estimator k depends on the numbers of approximating functions, i.e., m 1 and m 2 used in estimating () and g(;). In practice, one may select m 1 and m 2 in a data-dependent way, such as through the cross-validation. The cross-validated series nonparametric regression estimator