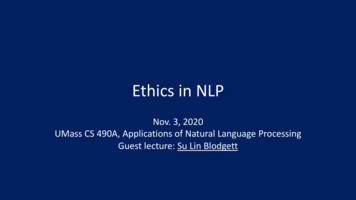
Transcription
Ethics in NLPNov. 3, 2020UMass CS 490A, Applications of Natural Language ProcessingGuest lecture: Su Lin Blodgett
Outline some examples of ethical issuesin NLP systems current state of ethics in NLP thinking through the NLPpipeline open questions discussion!
Occupational gender stereotypes:word embeddingsMany examples of ethicalissues in NLP systems:biased representationsBolukbasi et al. 2016
Occupational stereotypes:coreference resolutionMany examples of ethicalissues in NLP systems:biased outputsRudinger et al. 2018
Occupational stereotypes:machine translationMany examples of ethicalissues in NLP systems:biased outputsPrates et al. 2019
Many examples of ethicalissues in NLP systems:biased outputsQuartz
Toxicity detectionMany examples of ethicalissues in NLP systems:biased outputsHutchinson et al. 2020
Toxicity detectionMany examples of ethicalissues in NLP systems:biased outputsSap et al. 2019
Many examples of ethicalissues in NLP systems:discriminatory decisionsThe Verge
Many examples of ethicalissues in NLP systems:discriminatory decisionsThe Verge
Many examples of ethicalissues in NLP systems:privacyPCMag
demographic attribute predictionMany examples of ethicalissues in NLP systems:privacyHuang and Paul 2019
very new area: 2016 –The state ofethics in NLP ethics in NLP workshop 2017,2018 150 papers since then ACL 2020, NAACL and ACL 2021:ethics in NLP track primary focus: bias in NLP most focus on embeddings but also a wide range of tasks additional focuses/connections: privacy interpretability human-centered evaluation
Predicting mental health online benefits?Let’s speculate!(speculative harm analysis) better understand different experiencespossible interventionsmeasure population-level healthbetter design community spacesbetter design treatments risks?consentde-identificationdata sharinginferences used for some other purposeviolating community norms / diminishingaccess to community spaces bad predictions à bad interventions! incorrect population estimates risk to researchers’ own health
Belmont Report (1979)Reasoning aboutharms Respect for persons: protecting theautonomy of all people; allowing forinformed consent Beneficence: maximize benefits forthe research project and minimizerisks to the research subjects Justice: ensuring procedures areadministered fairly and equally NLP systems: not experiments inthe usual sense! scale broader sets of stakeholders lack of awareness of systems as theyare operating integration into larger pipelines indirect path to harm
Thinking through the NLP pipelineDeploy andevaluatemodelDefineproblemDefineand trainmodelCollectdataLabeldata
What counts as toxicity online?Define problem:Toxicity detection slurs and insultsphysical threatsdoxxingmicroaggressionsinciting violence or self-harmand other things that may breakcommunity norms
Collect data:Toxicity detection What are the effects of differentdata gathering approaches? keyword searches self-reports moderator-deleted content
Label data:Toxicity detection What kinds of things affectannotator decisions? differences of opiniononline cultural contextwider cultural contextagelanguage varietymembership in a minoritized groupdiscourse context availablespecific question asked
Label data:Toxicity detection What kinds of things affectannotator decisions? differences of opiniononline cultural contextwider cultural contextagelanguage varietymembership in a minoritized groupdiscourse context availablespecific question asked
Identifying and measuring harmsOpen questionsand directions Integrating social, historical, andpolitical context to understand whomay be harmed and how e.g., linguistic stigmatization Fairness and privacy tradeoffs Understanding systems in theirdeployed context e.g., hiring Measuring representational harms
Identifying and measuring harmsOpen questionsand directions Integrating social, historical, andpolitical context to understand whomay be harmed and how e.g., linguistic stigmatization Fairness and privacy tradeoffs Understanding systems in theirdeployed context e.g., hiring Measuring representational harms Understanding users’ livedexperiences
Designing betterOpen questionsand directions What ideas about language speakers affect design? Human-centered problemformulation, annotation, evaluation User awareness and recourse Meaningful co-participation ofstakeholders participatory design? Meaningful shifts in decisionmaking When not to build?
Open questionsand directions Exciting interdisciplinaryopportunities! Fairness, justice, and ethics inmachine learning and AI Sociolinguistics, linguisticanthropology, social psychology,education Human-computer interaction andsocial computing
ethics in NLP very new area: 2016 - ethics in NLP workshop 2017, 2018 150 papers since then ACL 2020, NAACL and ACL 2021: ethics in NLP track primary focus: bias in NLP most focus on embeddings but also a wide range of tasks additional focuses/connections: privacy interpretability human-centered evaluation