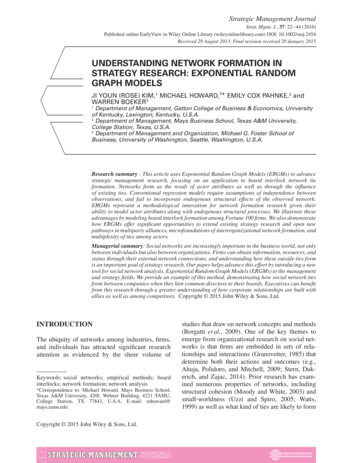
Transcription
Strategic Management JournalStrat. Mgmt. J., 37: 22–44 (2016)Published online EarlyView in Wiley Online Library (wileyonlinelibrary.com) DOI: 10.1002/smj.2454Received 29 August 2013; Final revision received 20 January 2015UNDERSTANDING NETWORK FORMATION INSTRATEGY RESEARCH: EXPONENTIAL RANDOMGRAPH MODELSJI YOUN (ROSE) KIM,1 MICHAEL HOWARD,2* EMILY COX PAHNKE,3 andWARREN BOEKER31Department of Management, Gatton College of Business & Economics, Universityof Kentucky, Lexington, Kentucky, U.S.A.2Department of Management, Mays Business School, Texas A&M University,College Station, Texas, U.S.A.3Department of Management and Organization, Michael G. Foster School ofBusiness, University of Washington, Seattle, Washington, U.S.A.Research summary : This article uses Exponential Random Graph Models (ERGMs) to advancestrategic management research, focusing on an application to board interlock network tieformation. Networks form as the result of actor attributes as well as through the influenceof existing ties. Conventional regression models require assumptions of independence betweenobservations, and fail to incorporate endogenous structural effects of the observed network.ERGMs represent a methodological innovation for network formation research given theirability to model actor attributes along with endogenous structural processes. We illustrate theseadvantages by modeling board interlock formation among Fortune 100 firms. We also demonstratehow ERGMs offer significant opportunities to extend existing strategy research and open newpathways in multiparty alliances, microfoundations of interorganizational network formation, andmultiplexity of ties among actors.Managerial summary: Social networks are increasingly important in the business world, not onlybetween individuals but also between organizations. Firms can obtain information, resources, andstatus through their external network connections, and understanding how these outside ties formis an important goal of strategy research. Our paper helps advance this effort by introducing a newtool for social network analysis, Exponential Random Graph Models (ERGMs) to the managementand strategy fields. We provide an example of this method, demonstrating how social network tiesform between companies when they hire common directors to their boards. Executives can benefitfrom this research through a greater understanding of how corporate relationships are built withallies as well as among competitors. Copyright 2015 John Wiley & Sons, Ltd.INTRODUCTIONThe ubiquity of networks among industries, firms,and individuals has attracted significant researchattention as evidenced by the sheer volume ofKeywords: social networks; empirical methods; boardinterlocks; network formation; network analysis*Correspondence to: Michael Howard, Mays Business School,Texas A&M University, 420L Wehner Building, 4221 TAMU,College Station, TX 77843, U.S.A. E-mail: mhoward@mays.tamu.eduCopyright 2015 John Wiley & Sons, Ltd.studies that draw on network concepts and methods(Borgatti et al., 2009). One of the key themes toemerge from organizational research on social networks is that firms are embedded in sets of relationships and interactions (Granovetter, 1985) thatdetermine both their actions and outcomes (e.g.,Ahuja, Polidoro, and Mitchell, 2009; Stern, Dukerich, and Zajac, 2014). Prior research has examined numerous properties of networks, includingstructural cohesion (Moody and White, 2003) andsmall-worldness (Uzzi and Spiro, 2005; Watts,1999) as well as what kind of ties are likely to form
Understanding Network Formation in Strategy Research: ERGMsbetween actors and which actors will become morecentral (e.g., Burkhardt and Brass, 1990; Shipilovand Li, 2012). At the same time, a better understanding of how and why organizational networksemerge is critically important to determine how different network structures offer distinctive benefitsor constraints to firms embedded in them (Ahuja,Soda, and Zaheer, 2012; Salancik, 1995; Stuart andSorenson, 2007).Network formation may be driven by multipleinterdependent social processes acting simultaneously (Hedström and Swedberg, 1998; Lusher,Koskinen, and Robins, 2013). Networks mayemerge through a process whereby actors seek partners with specific characteristics (e.g., homophily)or in response to opportunities made available bytheir partners’ reciprocal behaviors (Blau, 1964;Park and Luo, 2001), leading to changes in thenetwork structure (Contractor, Wasserman, andFaust, 2006). Alternatively, network ties mayresult from locally emergent structures (Gulatiand Gargiulo, 1999; Lusher et al., 2013; Zaheerand Soda, 2009), in which relationships amongactors are influenced by the presence (or absence)of other ties in the network. For example, in thecontext of the global cellular phone industry,Google’s choice to partner with Samsung and HTCaround the Android operating system and initiallypromote it through the Verizon carrier network inthe United States was strongly influenced by theexisting relationship that Apple had formed withAT&T. Google’s decision illustrates an endogenousnetwork structural effect—the choice to formnetwork ties with Samsung, HTC, and Verizonextends beyond simple considerations of their characteristics and involves a calculated response to theties already formed by AT&T. Observed networkstructure may thus result from the combination ofdistinct, simultaneous processes that may be interdependent and structurally emergent (Lusher et al.,2013) and co-evolve in a complex manner (Stuartand Sorenson, 2007; Zaheer and Soda, 2009).Prior strategy research has rarely examined thecomplex combinations of processes that simultaneously shape the structural characteristics of a givennetwork. Traditional network analysis uses regression methodologies that are based on the assumption that tie formation between two actors is independent of the other ties and actors in the network.However, this assumption can be problematic formany networks, in which new tie formation isan interdependent process influenced by both theCopyright 2015 John Wiley & Sons, Ltd.23characteristics of the actors and of their existingties (Ahuja et al., 2012; Contractor et al., 2006;Provan, Fish, and Sydow, 2007). Strategy networkresearchers often address this problem by usingmatched pair samples, rare events methods, orother advanced techniques in random effect logistic regression models. However, these approachesoffer only a partial solution. Moreover, conventionalstatistical methodologies are unable to account forthe endogenous structural effects that the existingrelationships between two firms have on the formation of ties to a third firm (Contractor et al., 2006).In the example of the global cellular phone industry, existing relationships among Google, HTC, andSamsung are likely to influence Microsoft’s subsequent choice to partner with Nokia. As a result,without modeling such endogenous structural processes, researchers run the risk of inappropriatelyattributing observed network tie formation to firmor dyad-specific characteristics (e.g., characteristicsof Microsoft or Nokia in our previous example),although such effects may be confounded by underlying interdependent structural processes (e.g., theexisting relationships among Google, HTC, andSamsung).The goal of this study is to highlight a relatively new analytical approach that allows scholarsto examine multiple interdependent social processesinvolved in network formation. We focus on a classof social network methodologies called Exponential Random Graph Models (ERGMs) to demonstrate how they permit better specification of theprocesses underlying network formation (Frankand Strauss, 1986; Pattison and Wasserman, 1999;Snijders et al., 2006). Broadly stated, ERGM analysis examines tie formation at the network level,accounting for potential cross-dependencies, emergent network structures (such as consortia or clusters of allied firms within an industry), and othereffects that cannot be addressed through conventional approaches, which focus primarily on dyadicrelationships. Thus, while ERGMs can model manyof the co-variates included in traditional regressions, they provide additional insights that increaseour understanding of how network structures form.In the example of the global cellular industry,ERGMs could provide insight into the likelihoodthat any two firms will form a tie, given the existingties between other firms in the industry.Currently, only a small number of organizationalstudies have utilized ERGMs (e.g., Faraj and Johnson, 2011; Lomi et al., 2014). In this article, weStrat. Mgmt. J., 37: 22–44 (2016)DOI: 10.1002/smj
24J. Y. (Rose) Kim et al.extend this research and demonstrate how ERGMscan be effectively employed for a much broader setof research questions to study strategy network phenomena. We suggest that ERGMs can be used toexplicitly capture the effects of endogenous localstructures, and more generally, to understand theantecedents and mechanisms involved in networkformation. Broadly stated, this category of researchcan be expressed through two questions: (1) How doobserved network structures emerge, and (2) Whatare the underlying social processes that lead to theemergence of these observed structures? With theirdistinctive advantages, ERGMs not only extendprior research, but also open up new research opportunities to enhance our understanding of strategytopics such as multipartner alliances, microfoundations of interorganizational network formation,and multiplexity (multiple types of ties) in socialrelationships.To demonstrate the benefits of ERGMs, we provide an example examining board interlocks amongFortune 100 firms and develop models to illustratethe comparison of traditional logistic regressionmethodologies to ERGM techniques. Prior researchhas examined different firm- and dyad-specific factors of interlock tie formation, yet few studies examine endogenous structural processes underlying theformation of board interlock networks (Harriganand Bond, 2013). Thus, we first examine whetherthere are any structural processes that shape theboard interlock network. We also test whether theinfluence of firm-, dyad-specific factors examinedin prior research persists after explicitly accounting for these structures. Our results show thatendogenous structural processes such as reciprocity(“returning the favor” by exchanging board interlock invitations) and triad closure (the greater likelihood of a tie forming between two actors wheneach is already tied to a third actor) play a significantrole in shaping this network. These results provideinsights not offered by other regression techniquesand indicate that interlock formation may partiallystem from broader social phenomena. At the sametime, when we control for local network structuraleffects using ERGMs, some of the firm-specificcharacteristics that have most often been studied inthe past using conventional techniques prove to beless consequential. For example, prior research hasproduced inconsistent results regarding the relationship between profitability and interlocks (Mizruchi,1996). Our findings help clarify this issue, revealinglittle evidence that directors of large or profitableCopyright 2015 John Wiley & Sons, Ltd.firms are more likely to be invited for a directorshipat other firms when network structural effects aretaken into consideration. Our application shows thatthe use of ERGMs provide not only rigorous empirical evidence for advancing scientific research, butalso opportunities for further theorizing the socialembeddedness view of board interlock formation assuggested in prior research (Withers, Hillman, andCannella, 2012). Furthermore, we offer potentialextensions of existing strategy research that couldbe made using ERGMs in domains such as alliancesamong multiple firms, microfoundations of interorganizational network formation, and different typesof concurrent ties among actors.NETWORK FORMATIONCurrent approaches in strategy researchExisting research in the strategy area has focusedon numerous actor and dyadic characteristics thatinfluence tie formation between organizations. Atthe actor (firm) level, a variety of firm-specific characteristics such as technical capability or organizational reputation have been shown to be importantpredictors of a firm’s propensity to form ties withother firms, for example through alliances (e.g.,Ahuja, 2000; Gu and Lu, 2013). In a directed network, sender’s and receiver’s characteristics mayinfluence the likelihood of a tie being formed.Existing research has also illustrated how theobserved network structure may be driven by characteristics of the dyad, showing how a relationalattribute between two parties can influence the formation of ties. As an illustration, homophily suggests that firms with similar characteristics are morelikely to have ties with one another than withother firms (McPherson, Smith-Lovin, and Cook,2001). However, homophily may sometimes limitthe benefits of connection in terms of informationor resources available in the network; thus, actorsmay partner with others who are different fromthem. For example, past research has shown howdyad-specific characteristics such as differences orsimilarities in firms’ resource endowments or theirrelative position with respect to markets, technologies, or geographic location are important factorsinfluencing tie formation (Diestre and Rajagopalan,2012; Rothaermel and Boeker, 2008). Finally, network ties emerge as a result of other types ofshared ties between actors, that is, multiplexity. ForStrat. Mgmt. J., 37: 22–44 (2016)DOI: 10.1002/smj
Understanding Network Formation in Strategy Research: ERGMs(a) ReciprocityFigure 1.(b) Popularity(c) Activity(d) Triad closure25(e) BrokerageDifferent types of network structures and associated endogenous processesexample, the presence of collaborative R&D relationships between firms may subsequently influence the formation of different kinds of ties such ascustomer-buyer relationships (Powell, Koput, andSmith-Doerr, 1996; Shipilov and Li, 2012). Similarly, characteristics of interlocking ties betweenindividual board members influence subsequentalliance formation between their affiliated organizations (Gulati and Westphal, 1999).Endogenous structural processesWhile network formation is influenced byfirm-specific and dyad-specific characteristicssuch as those highlighted above, networks can alsoemerge through broader social processes such asendogenous effects driven by the internal processesof the focal network (Lusher and Robins, 2013;Robins, 2009). For example, individual actorsmay act separately from each other without anyknowledge of or intention to shape the broadernetwork while still being influenced by each other’ssimultaneous actions (Gulati and Gargiulo, 1999;Uzzi and Spiro, 2005; Watts, 1999). In such cases,network ties are the result of endogenous processesinfluenced by existing network ties rather thanas a result of actor attributes or other exogenousfactors. Scholars have suggested five broad typesof endogenous structural processes that influencenetwork formation (Lusher and Robins, 2013), asillustrated in Figure 1.Reciprocity (a) is the most basic, yet one ofthe most important, tendencies in social interactions (Blau, 1964). It explains tie formation through“returning the favor,” reciprocating an earlier interaction with a network actor. The structure of thenetwork can also be influenced by the number anddirection of an actor’s ties. Popularity (b) illustrates the process by which already-popular actorsmay become even more popular, analogous to thewell-known “Matthew effect” in social scienceCopyright 2015 John Wiley & Sons, Ltd.(Barabási and Albert, 1999; Merton, 1968), oftendescribed as “the rich get richer.” For example,in interorganizational networks, firms with moreextensive alliance ties are more likely to engagein future alliances, subsequently influencing theirvisibility and attractiveness as a potential partner(Podolny, 1993; Podolny and Stuart, 1995). Tiesmay also more likely be generated by actors that aresimply very active in seeking new network connections, represented as Activity (c) in Figure 1. Thenetwork patterns associated with Popularity (b) andActivity (c) are often called “star” terms because oftheir star-like structures; specifically, “in-two-star”describes popularity with two incoming ties towardthe central node, and “out-two-star” refers to activity with two outgoing ties from the central nodein a directed network. In an undirected network,there is no need to differentiate the direction, and wewould refer only to a general “two-star” term. (Notethat there can be multiple ties outgoing/incomingto the central node; we depict only the simplestforms for purpose of illustration.) In network terms,a triad represents the structure of ties between anyset of three actors, with Triad Closure (d) indicating that two actors are likely to form a tie if theyare each tied to a separate common actor, creating atriangle connecting all three actors (Wasserman andFaust, 1994). “A friend of my friend is my friend”is an intuitive example of triad closure, which isalso referred to as the transitive influence on theformation of a tie between unconnected actors.Research suggests that firms may engage in triadclosure to obtain resources from multiple collaboration partners to counter the move of a competitoror to protect their own interests in an interorganizational network (Gomes-Cassares, 1994; Madhavan,Gnyawali, and He, 2004). Finally, ties may occur bythe network process of Brokerage (e) where an actorconnects others who are not directly connected.There are particular advantages that brokers enjoy,as Burt (1992) discusses in terms of the power andStrat. Mgmt. J., 37: 22–44 (2016)DOI: 10.1002/smj
26J. Y. (Rose) Kim et al.influence obtained by actors in a brokerage position,shown as the central node of Figure 1(e).Network formation is the result of numerousprocesses that interact and operate simultaneously(Contractor et al., 2006; Hedström and Swedberg,1998; Lusher et al., 2013). Strategy research onnetworks and ties has largely focused on how afirm’s characteristics or dyadic-specific attributesinfluence tie formation. More complex, endogenousstructural effects such as reciprocity, triad closureand brokerage have rarely been empirically investigated in a systematic fashion, although the notionthat firms’ decisions to create ties are influenced byothers’ behaviors is a central premise of networkresearch. This omission has important implicationsfor research on network formation. Failure toaccount for these endogenous structures may resultin model misspecification, attributing significanteffects to firm- or dyad-specific characteristicsthat may be confounded with structural processesindependently driving the network (Cranmer andDesmarais, 2011; Goodreau, Kitts, and Morris,2009; Harrigan and Bond, 2013). In contrast,properly accounting for these structural effects mayaid theory development, allowing us to identifycombinations of social processes underlying theemergence of network structures (Contractor et al.,2006). In the next section, we discuss why conventional regression models may be an inadequatetool for network formation research and introducerecent methodological advancements, ERGMs, asa better alternative.NETWORK METHODOLOGIESresearch has attempted to address this issue byemploying corrections such as the clustering ofstandard errors or the creation of separate control variables to correct for autocorrelation amongobservations (Lincoln, 1984; Stuart, 1998), or ithas treated the problem as a sampling issue andtackled it by using elaborate weighting methodologies (Barnett, 1993; Gulati, 1995). These techniquesmay provide an insufficient solution to the study ofnetwork formation, given the dependence of observations in relational data that make it impossible tocorrectly cluster standard errors or to appropriatelycontrol for oversampling (Greene, 2008).More importantly, standard regression methodsare generally not capable of incorporating variouslocal network structures such as triads stemmingfrom either endogenous structural effects or dyadiceffects (e.g., homophily between firms with similarattributes) because they are not independent ofeach other (Wasserman and Pattison, 1996). Thus,when conventional statistical models are used fornetwork formation research, they are likely tosuffer from model misspecification, even with theinclusion of firm- or dyad-specific co-variates.Causal inference about the influence of specificfactors may be incorrect given this confoundingeffect of the endogenous structural processes(Cranmer and Desmarais, 2011; Goodreau et al.,2009; Lusher et al., 2013). In sum, although standard statistical approaches are useful for researchfrom the perspective of the individual firm (i.e.,ego-centric perspective), they are inappropriate tostudy network formation when we have the goalof examining the multiple processes that operateconcurrently to generate a global network.Conventional regression methodologyfor network formation researchExponential random graph modelsPast network research in strategy has generallyemployed regression models such as logit or probit to study network formation among firms, witha focus on testing the effect of firm or dyadicco-variates (e.g., technical capability, resource complementarity) or some network characteristics (e.g.,centrality) on tie formation (Chung, Singh, and Lee,2000; Gulati, 1995; Gulati and Gargiulo, 1999).However, for a number of reasons, these approachesimpose significant limitations when examining network formation. Standard statistical methods arebased on assumptions of independence, which areproblematic for network data that are inherentlyinterdependent (Wasserman and Faust, 1994). PastExponential Random Graph Models (ERGMs) arewell suited to address the limitations of traditional regression methodologies. ERGMs are a classof statistical models for social networks whichaccount for the presence (or absence) of network ties (Robins et al., 2007a; Snijders et al.,2006; Wasserman and Pattison, 1996). Relative totraditional regression models, ERGMs provide asuperior approach to study network formation byexplicitly modeling endogenous dependencies thatmay shape networks along with exogenous factors such as actor- or dyad-specific characteristics(Lusher et al., 2013; Robins et al., 2007a). ERGMsmodel ties as being interdependent, resulting in aCopyright 2015 John Wiley & Sons, Ltd.Strat. Mgmt. J., 37: 22–44 (2016)DOI: 10.1002/smj
Understanding Network Formation in Strategy Research: ERGMsvariety of local network configurations (as represented in Figure 1). These network configurationsare consequential patterns that may reflect important social processes such as reciprocity or clustering that simultaneously affect the formation ofa global network. The formation of ties and network structures is tested for statistical significancerelative to what might be expected through randomtie formation, conditioned on other effects in themodel. ERGMs do not require the assumption ofindependence between network ties and avoid theneed for a matched sample design or the bias correction techniques for rare events that have been usedin prior network research.ERGMs can incorporate different types ofnetwork configurations and estimate their effectson network formation. For instance, ERGMs candescribe the likelihood of the formation of reciprocal ties or whether a tie between two actors, alreadytied to a common third actor, is more likely to beobserved (i.e., triad closure) in an overall network,conditioned on the structure of the network. Giventhat structural outcomes such as triad formationhave been shown to be interdependent, such aninference is problematic for standard statisticalmethods (Wasserman and Faust, 1994).Moreover, ERGMs can accommodate any number of binary, categorical, or continuous actor- ordyad-specific co-variates as well to see whether theyare associated with the formation of network ties.ERGMs can also be used to analyze different typesof networks such as directed and nondirected networks, bipartite, and multiplex networks with various types of nodes and relationships (Lusher et al.,2013; Robins, Pattison, and Wang, 2009; Wang,2013; Wang et al., 2013). Therefore, ERGMs provide a powerful, flexible tool to separate varioussocial processes operating concurrently and evaluate the relative contribution of each on the formation of the observed network structure (Lusheret al., 2013; Robins et al., 2007a). At least in thisaspect, these broader capabilities of ERGMs are insome ways analogous to the advantages of statisticalanalysis tools such as structural equation modeling(SEM), which allow researchers to simultaneouslycapture wider system-level effects. For example,as SEM expands from conventional cause-effectregression modeling to the concurrent analysisof more complex co-variance structures, ERGMsgo beyond nodal and dyadic factors modeled inconventional logistic regression to capture diversefactors at the broader network level.Copyright 2015 John Wiley & Sons, Ltd.27ERGMs trace their origins to influential work byFrank and Strauss (1986) who applied spatial statistical approaches to networks and proposed Markovrandom graph models to directly address the issueof interdependence. The models became widelyknown as p* models from the seminal work byWasserman and Pattison (1996), which broadenedthe use of this approach to network analysis. A number of subsequent advances, including adaptationsfor local “neighborhood” network structures (Pattison and Robins, 2002) and methods for overcomingthe problem of model degeneracy (Hunter andHandcock, 2006; Robins et al., 2009; Snijders et al.,2006) have helped develop ERGMs into a viabletool for analyzing real world network data.Recently, ERGMs have begun to be adopted in awide range of social science disciplines, includingsociology, demography, communications, and political science (e.g., Atouba and Shumate, 2010; Cranmer and Desmarais, 2011; Goodreau et al., 2009;Wimmer and Lewis, 2010). A small, yet growing number of recent organizational studies usingERGMs demonstrates the potential of the methodology as a useful tool for modeling multiple socialprocesses, though these techniques have seldombeen applied to empirical research focused on strategy questions. We provide a summary of recentstudies using ERGMs in organizational research inTable S1, along with a description of how they applyERGM techniques.STATISTICAL INFERENCE THROUGHERGMSStatistical framework and dependenceassumptionsERGMs have the following form][ 𝜂A zA (x) ,Pr (X x) (1 k) exp(1)Awhere Xij is a random variable that represents a tiebetween actor i to actor j (Xij 1 if there is a tie fromactor i to j, and 0 otherwise). These ties are represented in an n n adjacency matrix (n number ofactors in the network) denoted as X, and x denotes amatrix of realized ties in the network. When ties arenondirectional (edges), X is symmetric. When tiesare directional (arcs), Xij Xji. The A refers to different network configuration types. The zA (x) termsStrat. Mgmt. J., 37: 22–44 (2016)DOI: 10.1002/smj
28J. Y. (Rose) Kim et al.are model co-variates, denoting any set of A networkstatistics calculated on x and theorized to affect theprobability of this network forming. Examples of zstatistics are the number of ties, the number of tiesbetween firms with a shared nodal characteristic, orthe number of closed triads and so on. Equation 1may be modified by replacing zA (x) with zA (x, P)to accommodate additional co-variate informationP such as firm- or dyad-specific characteristics. The𝜂 A coefficients are unknown parameters to be estimated, and they determine the effect of the networkstatistics included in the model for the observednetwork. The k stands for the quantity from thenumerator summed over all possible networks withn actors. It constrains the probabilities to sum to 1.Simply put, ERGMs place more or less weight onnetworks with certain features, as determined by 𝜂 A(i.e., parameters), and zA (network statistics). Theequation above can also be expressed in terms ofthe conditional log-odds (logit) of individual ties:)) ( ( 𝜂A 𝛿zA (x) , (2)logit P Xij 1 n, XijcAwhere Xijc denotes the rest of the network other thanthe single variable Xij , and 𝛿 Z A is the amount bywhich zA changes when Xij is changed from 0 to1. The presence of Xijc in the conditional statementin the left-hand side of the equation represents themutual dependence of ties, showing how ERGMsexplicitly accommodate interdependent observations. This alternative specification also clarifies theinterpretation of 𝜂 A vector, the coefficients of interest. If forming a tie increases zA by 1, then all otherthings being equal, the log-odds of that tie formingincrease by 𝜂 A .To implement ERGMs, it is important to understand the dependence assumptions that define theways in which the observed ties may be related(Brandes et al., 2013; Pattison and Robins, 2002;Robins et al., 2007a). A particular dependenceassumption determines the different types of network configurations to be included in a model(Lusher et al., 2013; Robins et al., 2007a). The simplest dependence assumption is that all possible distinct ties are independent of one another; they occurrandomly with a certain fixed probability. A second assumption is that dyads are independent of oneanother. For example, reciprocity in a directed network is a form of dependency assumption where thetwo possible directed ties within a dyad are dependent on each other. This assumption is sometimesCopyright 2015 John Wiley & Sons, Ltd.referred to as “dyadic independence” because thereare no other effects outside the dyad that influencethe formation of ties, and so the dyads are independent of each other. However, these two assumption
1 Department of Management, Gatton College of Business & Economics, University of Kentucky, Lexington, Kentucky, U.S.A. . 3 Department of Management and Organization, Michael G. Foster School of Business, University of Washington, Seattle, Washington, U.S.A. Research summary: This article uses Exponential Random Graph Models (ERGMs) to advance