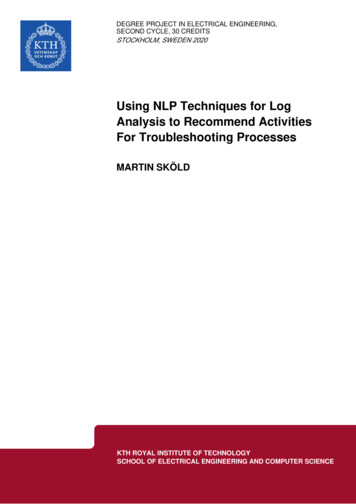
Transcription
FACULTY OF EDUCATION AND BUSINESS STUDIESDepartment of Business and Economics StudiesSearch Engine Optimization and the connection withKnowledge GraphsMilla Marianna HietalaOliver Marshall2021Student thesis, Master degree (one year), CreditsBusiness AdministrationMaster Programme in Business Administration (MBA): Business ManagementMaster Thesis in Business Administration 15 CreditsSupervisor: Ehsanul Huda ChowdhuryExaminer: Maria Fregidou-Malama
AbstractTitle: Search Engine Optimization and the connection with Knowledge GraphsLevel: Thesis for Master’s Degree in Business AdministrationAuthors: Milla Marianna Hietala and Oliver MarshallSupervisor: Ehsanul Huda ChowdhuryExaminer: Maria Fregidou-MalamaDate: 28-01-2021Aim: The aim of this study is to analyze the usage of Search Engine Optimization andKnowledge Graphs and the connection between them to achieve profitable business visibilityand reach.Methods: Following a qualitative method together with an inductive approach, ten marketingprofessionals were interviewed via an online questionnaire. To conduct this study bothprimary and secondary data was utilized. Scientific theory together with empirical findingswere linked and discussed in the analysis chapter.Findings: This study establishes current Search Engine Optimization utilization bybusinesses regarding common techniques and methods. We demonstrate their effectivenesson the Google Knowledge Graph, Google My Business and resulting positive businessimpact for increased visibility and reach. Difficulties remain in accurate tracking proceduresto analyze quantifiable results.Contribution of the thesis: This study contributes to the literature of both Search EngineOptimization and Knowledge Graphs by providing a new perspective on how these subjectshave been utilized in modern marketing. In addition, this study provides an understanding ofthe benefits of SEO utilization on Knowledge Graphs.Suggestions for further research: We suggest more extensive investigation on the elementsand utilization of Knowledge Graphs; how the structure can be affected; which techniques aremost effective on a bigger scale and how effectively the benefits can be measured.Key Words: Search Engine, Search Engine Optimization, SEO, Knowledge Graphs, GoogleMy Business, Google Search Engine, Online Marketing.I
AcknowledgementsWe would like to thank our supervisor Ehsanul Huda Chowdhury for his valuable help andadvice throughout the whole research process, the examiner Maria Fregidou-Malama for herconstructive criticism and guidance, our class colleagues which led the seminar oppositiongiving us new angles to explore and all professors who supported us during this program.We would also like to thank the interviewees for providing their insights and making thisresearch possible.Lastly, a special thank you for our family and friends for the support we received during theprocess. Without their endless encouragement this thesis would not have been possible.University of Gävle, SwedenJanuary 28, 2021Milla Marianna Hietala & Oliver MarshallII
Table of Content1Chapter 1. Introduction1.1 Background11.2 Motivation of the study21.3 Problematization21.4 Research Aim and Research Questions41.5 Delimitations41.6 Disposition45Chapter 2. Literature Review2.1 Knowledge Graph52.1.1 Definition of Knowledge Graph52.1.2 Semantic Search72.1.3 Google Knowledge Graph102.1.4 Business applicability of Knowledge Graphs132.2 SEO142.2.1 Search engine and Search engine result page (SERP)142.2.2 Search engine optimization (SEO)152.2.3 Keyword selection162.2.4 Black Hat SEO vs. White Hat SEO182.2.5 The benefits of SEO for business202.3 Theoretical Framework22Chapter 3. Methodology253.1 Research Approach253.1.1 Qualitative and quantitative approach253.1.2 Inductive approach263.2 Data collection263.2.1 Secondary data263.2.2 Primary Data273.3 Selection of respondents283.4 Operationalization of the interview questions313.5 Data analysis353.6 Validity and reliability363.7 The ethical aspects363.8 Limitations of the Methodology3738Chapter 4. Empirical Results384.1 BackgroundIII
4.2 SEO394.3 Google Knowledge Graph414.4 Google My Business424.5 Summary of Empirical Findings4446Chapter 5. Discussion5.1. SEO465.2 Google Knowledge Graph495.3 Google My Business5052Chapter 6. Conclusion6.1 Discussion of the research questions526.1.1 How do businesses practice SEO?526.1.2 How can businesses utilize search engine optimization to benefit from and influenceknowledge graphs?536.2 Implications546.3 Limitations and suggestions for future research55References57Appendices66Appendix 166IV
List of TablesTable 1: Summary of Knowledge graph definitions based on peer-reviewed journal articles.6Table 2: Concise overview of the evolution of semantic search as basis for knowledge graphs.9Table 3: Examples of white hat and black hat SEO methods (own compilation).19Table 4: Emphasizing the importance of SEO for marketing in previous studies.21Table 5: Interview information (own compilation).30Table 6: Interview questions and purpose (own compilation).31Table 7: Summary of Empirical Findings.44List of FiguresFigure 1: Semantic Search by Amerland, 2014, page 11.Figure 2: “Shakespeare” Google search page, Google (2020), own search.810Figure 3: “Gallerian Nian” Google search page and Google My Business listing,Google (2020), own search.12Figure 4: Google search result page, Google (2020), own search.14Figure 5: Theoretical Framework.24V
ABBREVIATIONSGMB - Google My BusinessIT - Information technologyPPC - Price Per ClickRDF - Resource Description FrameworkSEO - Search Engine OptimizationSERP - Search Engine Result PageVI
This page is left intentionally blankVII
Chapter 1. IntroductionThis chapter presents the background of the study, explains the motivation of the study and theproblematization of the topic is discussed. Additionally, the research aim and questions are stated.1.1 BackgroundEver since its introduction, the internet has become an important platform for businesses to interactwith their customers (Pant and Srinivasan, 2010). With the number of web users continuously risingon a daily basis and surpassing half of the earth’s population in 2017 (Ballatore, Graham, Sen, 2017),the need for companies to provide an online presence has become undeniable.As a result of inherent growth, the internet has been cluttered with information that inhibits effectivenavigation and the location of key knowledge. Therefore, search engines have become increasinglyimportant to access relevant information with many people utilizing them as a starting point for theirweb navigation. An indicator of their success can be seen by the search engine of Googleaccomplishing the feat of one billion unique monthly visitors as the first website in the world in 2011(Baye et al., 2015). While searches encompass a broad spectrum, most commonly researched topicsare in relation to products and services underlining the importance to businesses (Dou et al., 2010).Through the introduction of the Knowledge Graph in 2012, Google has established furtheroptimization for its search engine and provided an important technological advancement for the futureof information search (Singhal, 2012). Defined as a database shaped by user searches and keywordapplications, this machine learning technology provides information and recommendations beyondregular search terms to provide users an all-encompassing overview and incite further learning(Nigam et al, 2020). While primarily developed for search enhancements, businesses have adoptedthe tool and Knowledge Graphs have evolved to an unknown common practice utilized in many areassuch as Social Media (Lian et al., 2017), provision of business intelligence (Bellomarini et al. 2019)and voice assistants (Dong, 2019).In light of constant changes to search algorithms by search engines and modern techniques to reachaudiences, businesses and marketers need to stay up to date to stand out from the masses. As such,search engine optimization (SEO), has become an often-utilized marketing strategy and valuable assetto distinguish products from competitors by increasing search engine rankings through the use ofspecified keywords by website integration (Dramilio, et al., 2020; Sheffield, 2020).1
This study focuses on Search Engine Optimization and the extended connection to KnowledgeGraphs. Our goal is to establish a profound overview of commonly utilized techniques in conjunctionwith a mostly undervalued topic in current business literature. By generating insights into currentusage of SEO by marketing professionals and their respective companies we can establish links toKnowledge Graphs and the possibility to increase business visibility and reach.1.2 Motivation of the studyThrough our extensive literature review in the field of search engine optimization, we realized theexistence of Knowledge Graphs and their growing effect on modern life from a consumer perspective.Moving forward we would like to investigate in which way businesses are aware of this topic andhow they utilize search engine optimization to benefit from and influence Knowledge Graphs. As therecent development of Knowledge Graphs is not common knowledge to all marketers, we want toprovide an overview and highlight the existence of this tool.Our motivation is to firstly, demonstrate the marketing strategy of search engine optimization, theembedding of keywords, links, images in websites to generate website traffic through increasedsearch rankings in search engines. Secondly, we link the topic with knowledge Graphs, a new toolwhich automates the collection of data from websites via algorithms to provide search engine users afast and broader overview of their searched query. Nowadays, Knowledge Graphs are omnipresentand provide the basis for Google searches and many of the current recommendations on for exampleYouTube, Netflix, Facebook, LinkedIn, and many more (Nigam et al., 2020).Previous research in this field has been conducted on Knowledge Graphs mostly from an ITperspective analyzing integration and advancement of technology (Fathalla et al. 2017; Coursey,Mihalcea, 2009; Xiaoxue,2019), the benefactors of Artificial Intelligence application (Bellomarini et.al, 2019), concise information sharing through Knowledge Graphs and social media (Lian et al.,2017). While there has been much research written from a business and marketing perspective in theform of blog posts (White, 2020; Job, 2019; Pecánek, 2020), little to no peer-reviewed academicresearch journals have focused upon this topic giving us a unique angle and opportunity to engage.1.3 ProblematizationDifferent digital marketing tools have been modifying the field of marketing over the past decade(Schaefer and Hetman, 2019). Because of the technology improvements, it is getting easier to target2
consumers in more specific and personal content (Kostin, 2018) which makes the marketing moreefficient and cost-efficient.Since with the knowledge graph, the idea is to collect, store, and utilize the searched keywords datato offer results that are accurate and relevant (Brack et al., 2020), it makes the targeted marketingeasier to put into practice.With the knowledge graph, there are huge amounts of collected data that can be used and reused(Nigam et al., 2020) and the challenge comes, when choosing how to utilize it the most effective way.In order for companies to appear in knowledge graphs, they need to have traffic on their website andmost clicks on search results (Tran and Takasu, 2019). This means it is crucial for companies to havetheir SEO working for search engines to find the created content and make it accessible in the searchresults pages.Companies can improve their visibility and rank on search results with SEO (Yang et al., 2019) andsince it’s linked to knowledge graphs modern-day marketers should be aware of how to utilize thisconnection.Knowledge graphs have not been reviewed from a business and marketing perspective in manyacademic research journals but mainly in the industry experts’ blog posts (Job, 2019; Pecánek, 2020).There is no research on how widely the tool is known among current marketers.So far knowledge graphs have been researched mainly from the IT perspective of analyzingintegration and advancement of technology (Fathalla et al. 2017; Coursey, Mihalcea, 2009;Xiaoxue,2011). We identified a research gap by the lack of academic research regarding theutilization of Knowledge Graphs from a business perspective. In addition, we believe SEO to have apositive business impact on Knowledge Graphs which will be focused upon in this research.3
1.4 Research Aim and Research QuestionsThe aim of this study is to analyze the utilization of SEO and Knowledge Graphs. Firstly, businesspractices of SEO are studied. Secondly, our research engages common SEO practices and establishescurrent and potential usage on Knowledge Graphs to increase businesses visibility and reach. Withour research we demonstrate a beneficial connection between the two topics.To achieve the aim, two research questions are formed:RQ1: How do businesses practice SEO?RQ2: How can businesses utilize search engine optimization to benefit from and influenceKnowledge Graphs?1.5 DelimitationsDifficulties in the interview selection process due to a very specialized topic narrow the scope andresponses of this study. In total 10 interviews were conducted with respondents from differentbackgrounds, experience levels and countries. Despite our interviewees being chosen carefully, andbeing handpicked marketing professionals, we could not ensure previously existing knowledge onthe topics at hand. These factors and due to the nature of this research provide a specified scope whichlimits our analysis to the marketing field and specifically to SEO, whereas a broader approach couldencompass the entirety of the field of Knowledge Graphs. While we provide an extensive overviewof our research questions our qualitative research is mostly unrestricted, focusing entirely onmarketing professionals regardless of industries, management positions or countries.1.6 DispositionThis research begins with an introductory chapter that describes the research background,problematization, motivation of the study together with the aim and research questions. Chapter twoconsists of different theoretical frameworks of previous studies and relevant concepts are discussedin detail. Chapter three explains the methodology of this study. The choice of data collection isexplained, and empirical data is presented, including the basic information about the respondents.Chapter 4 deals with the results of the empirical findings and chapter 5 follows an in-depth analysiswith comparisons to the theoretical framework, applicability, and outcomes. In the last chapter, theconclusion of the study is presented along with the answered research questions and aim. The chapterends with a summarization of contributions, limitations, and suggestions for future research.4
Chapter 2. Literature Review2.1 Knowledge GraphThis chapter explains the definition of Knowledge Graphs, its basis of semantic search, the Googleknowledge graph and further in-depth analysis of Knowledge Graph business practices.2.1.1 Definition of Knowledge GraphIn recent times, the term “Knowledge Graph” has been used abundantly by both businesses andresearchers to describe a complex technology on the basis of data storage and search enhancement(Nigam et al., 2020). Coined and brought into popularity by Google’s Knowledge Graph in 2012, thetopic has risen in fame without the company giving a clear definition or explanation of its exactprocedures (Ehrlinger & Wöß, 2016). Several researchers (Paulheim, 2016; Ehrlinger & Wöß, 2016)have noted the lack of generalized definition and attempted to create an overview and common groundfor future utilization (see Table 1).According to Pujara et al. (2013), Knowledge Graphs are described as systems encompassingtechniques to gain new knowledge from the internet. Through the combination of facts an interrelationis created to enhance knowledge generation. This definition is supported by Paulheim (2016) whohimself describes the term as, real world entities and connections combined in a graph form, to formprobable relations specifically to allow affiliations with each other over the range of differentconfined topics. In contrast Färber et al. (2016) mention a more technical definition, describing theKnowledge Graph as an “Resource Description Framework” or RDF graph defined by a subject,predicate and object which act as translators of extracted knowledge. After a thorough analysis ofsimilarities and issues in each definition Ehrlinger & Wöß (2016) combine existing knowledge toconstruct a clear description of knowledge graphs as a method to generate and include informationinto a complex knowledge base, also named ontology, where software establishes conclusions toassume new knowledge on the topic at hand. By storing large data sets derived from existing datathroughout the internet, knowledge graphs can find relations and suggest further searches or resultsand therefore incite further learning or ultimately business value for companies. Simply put, aknowledge graph links information together to display the most probable search result despite userambiguity.5
Table 1: Summary of Knowledge graph definitions based on peer-reviewed journal articles.ScholarsPujara et al.,Research IssuesKnowledge GraphExtraction of knowledge,IdentificationChallenges of implementation,(2013)Färber et al.,ContributionDefinitionKnowledge GraphDefinition, TechnicalData OriginViewpoint, ValidityKnowledge graphKnowledge graphrefinement surveyconstruction, Definition,of approaches andRefinement of existingevaluation methodsknowledge graphs,Definition ofOverview of existingKnowledge Graphsdefinitions, Summary,(2016)Paulheim,(2016)Ehrlinger & Wöß,(2016)Nigam et al.,(2020)Knowledge graph explanationApplication ofOverview of businessKnowledge Graphsapplications, General usage,for ServiceDefinitionProvidingPlatforms6
2.1.2 Semantic SearchTo explain the basis of Knowledge Graphs we must first look at the evolution of search in the recentyears (see Table 2). With increasing amounts of information and knowledge available throughout theinternet, it has become more and more difficult to search and find relevant results. Improvements tosearch practices are mandatory to keep up with current standards, therefore searches have changedfrom a stationary tool to include further information or apparent intelligent thinking when displayingits results. This is the effect of semantic search.First mentions of the concept arose by Guha et al. (2003), explaining in detail the development of thesemantic web to include further linkage between terms beyond pure hyperlinks and thereforesystematically laying the groundwork for inherent advantages for search engines in the form ofsemantic search. While the basis of their research was conjured around assumptions about futuredevelopment, further studies since then have been created to optimize their ideas for increasedinformation retrieval.Fernandez et al. (2008) relay the first successes of semantic search systems with ontologies, advancedknowledge management systems storing numerous data, specifically for business intranets. Whilethese results were promising for 2008, they also mention the lack of attempts of semantic searchapplication to the internet and demonstrate research proving the possibility of semantic search basedon ontologies in an unrestricted environment as the entirety of the world wide web.After further advancement Amerland (2014) discusses the large-scale implementation of semanticsearch in the world wide web and describes the transition from single web page results based onkeyword-activation with a probability of entailing relevant information to a search mechanicproviding intelligent results leading to a direct answer. The switch from links provided by keywordsto semantic search proved beneficial for the end user to find direct access to web pages related to thesearch question. According to the author the reasoning for the delayed launch across the internet wasin part mostly due to difficulties in handling large amounts of data and scalability. To ensure semanticsearch works over the trillions of existing web pages it has to be firstly automatic and secondly stillensure the required quality. While quality in searches used to be also reliant on the users searchqueries, semantic search diminishes ambiguity and allows the search engine to make assumptions onthe expectations of the searcher by calculations based on user preferences and previous searches.7
To demonstrate the impact and importance on the construction of Knowledge Graphs we must lookat the 3 components of semantic search which paved the way for its development.Figure 1: Semantic Search by Amerland, 2014, page 11. URI or Universal Resource Identifier defines a weblink, or name of an entity, which can beestablished as the initial set of data starting the search. It contributes to amassing largenumbers of raw data about the search query without further defining or categorizing itsvalidity. Its use is therefore limited and requires further classification. This is achieved via the RDF or Resource Description Framework translating the data via aset list of rules from one database to another. Amerland describes the process as givingmeaning to raw data indexed for machine use and the translation into understandable humanterms. During the transcription procedure the technology can fail to distinguish betweendistinct words, as uses and meanings can be differential depending on relevant contexttherefore an additional step is mandatory to fulfil an adequate search. To provide a reasonable answer for ambiguous queries and provide context beyond the searchterm an Ontology is used. These are a database collection of meanings based on classes withspecific rules to combine data. Through the usage of ontologies data can be linked with logicalconnections which are similar to human comprehension and intelligence.8
Ultimately the combination of all factors of semantic search led to the construction of knowledgegraphs as an extension of the existing technology (Cheng et al., 2017). At this stage we can establishfew differences in technological standpoints but more so in visual representation by businessescovered in the next chapters.Table 2: Concise overview of the evolution of semantic search as basis for knowledge graphs.ScholarsResearch IssuesContributionGuha, McCool,Semantic SearchConcept of semantic search,Miller,Implementation, Suggestion(2003)Fernandez et al.,Semantic SearchExpansion of semantic search,for the InternetEvolution from intranets,(2008)Amerland,Unrestricted OntologiesGoogle SemanticDefinition of semantic search,Searchexplanation of widespread(2014)implementation in the WWW,definition of knowledge graphsCheng et al.,(2017)Three levelKnowledge graph technology,customer serviceimplementation, practical usesknowledge graphs9
2.1.3 Google Knowledge GraphWith their blog post in 2012 Google introduced their newest product update as the Knowledge Graphto enhance their semantic search in a way to incorporate real life figures, objects, places, or entitiesmore engagingly with increased user friendliness (Singhal, 2012). They disclosed the scope of theirknowledge graph to contain over 500 million objects and 3.5 billion facts interrelated within eachother at its implementation. With the technology updating based on users' search terms, frequencyand relevant data backed by renowned sources, Google promises the relevance of search results.Applicable for both Desktop and Mobile search, this implementation proved a new age for searchengines (Lee, 2012). Since then, the blog post has been cited over 600 times according to GoogleScholar and sparked interest in Knowledge Graphs of both researchers and businesses.The Google knowledge graph contains summarized information in Knowledge Fields aiming to givea concise overview of the specific topic and establish further recommendations of connected fields(Sullivan, 2020). Below, the search query for “Shakespeare” resulted in detailed information ofWilliam Shakespeare’s life and work. The information is generated automatically and displayeddirectly without further need of additional search. Related searches containing similar famous artistscan be found which have been frequently used as follow-up to the initial search query and could beof interest to the user.Figure 2: “Shakespeare” Google search page, Google (2020), own search.10
According to Venetis et al. (2011) approximately 20% of all web searches are based on finding localinformation, with this number doubling for searches from mobile phones. With advanced technologyable to pinpoint the exact location of users the importance of having a company listing, availableacross search platforms, has been increased. Khan et al. (2015) demonstrate the importance of localsearch for marketeers by highlighting the users' need of quick decision making on the spot. Generallylocal searches on mobile phones are made by people wanting fast decisions and answers, thereforefactors such as reputation, closeness and visual representation are important for users' choices. Clearbusiness listings can provide major value for companies and let them stand out from the masses.To display relevant information about businesses “Google My Business” is utilized to enableautomatically generated business listings via Knowledge Graphs. Companies can claim these fieldsby providing valid proof of ownership for customization of information for their customers. Thisplatform includes display of Google Maps, opening times, Q&A sections, reviews and links to socialmedia. Companies also have the option to show special offers, promotions and upload relevantbusiness photos. Customers can directly engage with companies and ask questions or leave theircomments in the form of reviews with ratings ranging from 1-5.Below the search page for “Galleria Nian”, a shopping center in Gävle, Sweden, can be found whichdisplays its Google My Business listing automatically generated by the Knowledge Graph. In thiscase, the business has taken care of their GMB page by providing an additional informativedescription of the available services and up-to-date information regarding COVID-19 practices andregulations. Regular engagement with customer Questions in the Q&A section as well as a largeamount of google reviews provide an overview of what a customer can expect by visiting thisshopping center.11
Figure 3: “Gallerian Nian” Google search page and Google My Business listing, Google (2020),own search.12
2.1.4 Business applicability of Knowledge GraphsIn modern times knowledge graphs have become widespread in their business application withcompanies engaging the practicality and benefiting from their uses. According to Ehrlinger & Wöß(2016) large corporations like Facebook, Microsoft and Yahoo have followed suit after Google’s ownimplementation and developed knowledge graphs to support semantic searching and visualization.The usage has become a standard procedure rather than an exception.Zou (2020) analyzes in detail the usage of knowledge graphs and categorizes four main areas whichhave been identified for application: Question answering systems, by enhancing semantic awareness of social chatbots & digitalassistants (Siri, Alexa etc.) Recommender systems, support of accuracy and diversity Information retrieval, in form of search engine enhancements Domain specific utilization, e.g., in the medical, cyber security, news, and education sectorsNigam et al. (2020) focus primarily on clarifying the current uses of knowledge graphs in daily life,mentioning specifically the underlying impact they have had on service providers. Enrichingrecommendation systems has been a primary use of knowledge graphs to ensure connections whichmake sense and will be beneficial for users. In this regard the authors mention specific exampleswhich have benefitted from this development such as the financial sector, LinkedIn, Facebook, andNetflix. By engaging more frequently with the platform, recommendations can be enriched to enhancenot only personal but recommendations of all users. For video on demand providers such as Netflix,Hulu and Youtube this research proves valuable due to the increasing amount of content available onthe web (Orlandi et al., 2018). Via knowledge graphs users receive accurate representations of theirpreferences which proves beneficial for all parties involved.Lastly, it is worth mentioning a current research topic by Amazon which is focusing on developing aproduct graph for all products in the world (Dong et al., 2020). Upon successful implementation thisconstruction will provide improved accuracy, consistency, and completeness of data for Amazon'sproduct catalogue potentially changing the way we search for products and shop in the future.13
2.2 SEOThis section describes the core concepts of SEO: search engine, search engine optimization, the keymethods and White & Black Hat SEO.2.2.1 Search engine and Search engine result page (SERP)A web search engine is software, such as Google and Bing, which collects data from websites thatare stored in databases and from there provide the most relevant result for the searched topic (Li etal., 2019). The search engine shows search engine result pages (SERPs) consisting of a list of indexedwebsites for specific keywords (Rana, 2016). As figure 4. indicates the search engine result pageshows how a specific website is displayed and evaluated by the search engine.Figure 4: Google search result page, Google (2020), own search.The highlighted sections on the Google search result page are discussed further: The search box is the input section of a search engine where users c
I Abstract Title: Search Engine Optimization and the connection with Knowledge Graphs Level: Thesis for Master's Degree in Business Administration Authors: Milla Marianna Hietala and Oliver Marshall Supervisor: Ehsanul Huda Chowdhury Examiner: Maria Fregidou-Malama Date: 28-01-2021 Aim: The aim of this study is to analyze the usage of Search Engine Optimization and